The Rise and Stall of Data Marketplaces: A Critical Look
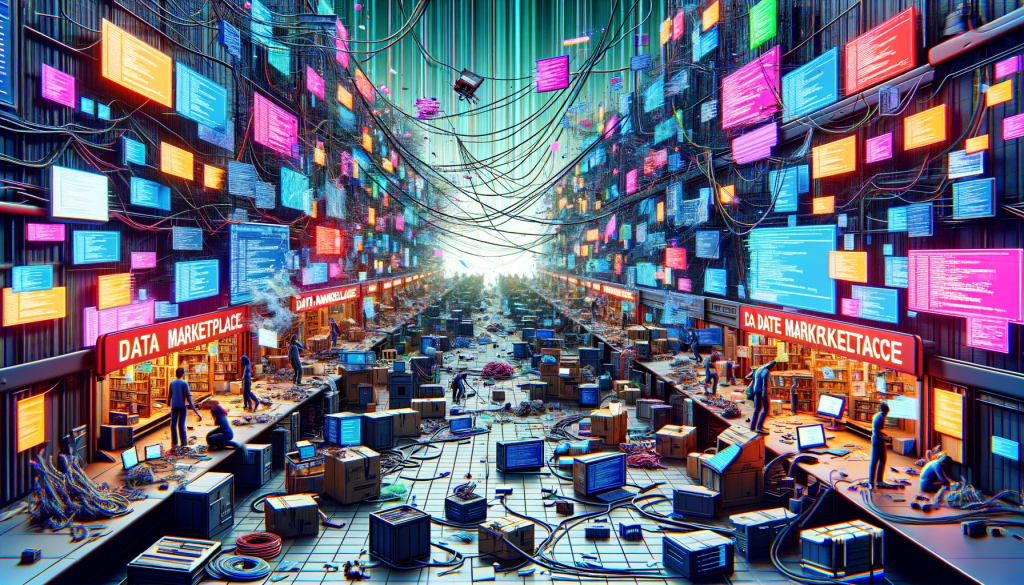
The pathway to mass data adoption is littered with the carcasses of data marketplace after data marketplace. With the explosion of data science, Large Language Models and data creation volumes, this should be the era of the data marketplace. Every year I read about dozens of new data marketplaces, lists and guides. At the same pace I also read about marketplace after marketplace no longer being maintained and many pivoting business models. Why is there no central marketplace for data? Because the data market is very different than most other markets, and traditional marketplace models aren’t well adapted to it. In this article we’ll dive into the issues and possible solutions.
Marketplace Structures vary wildly
To understand the dysfunction of the data market it’s important to look at other markets which are functioning more efficiently. Let’s first start with the home services market (think Angie’s List or Handy). Modern home services markets typically consist of providers ranging from landscapers to electricians to roofing experts. Most service providers neatly fit their services into a single category of service. When something goes wrong with your home, such as you develop a crack in your driveway, it’s pretty clear that you’re looking for the category Driveway Repair. When your toilet stops working, you know you need to go to the Plumber Category. Discovery in this type of market is very simple because of the logical grouping of service providers and the clear distinction between categories of service. It’s also simplified because buyer needs in the market are very similar. From the marketplace’s point of view every buyer is trying to remedy an issue or make some type of home improvement. Buyers don’t have highly specific needs that differ from the norm.
Another example of a functional market is home rentals. While once highly fragmented, it has become heavily consolidated. Buyers in this market have several personas (vacationer, business traveler, remote worker, event planner, etc), but still, only a small, fixed number of buyer roles exist. On the seller side it’s a little harder to put properties into buckets. You end up with homes filtered by attributes such as the number of rooms, the price range, the location, etc. This type of market forces the buyer to do more work, wading through hundreds of properties and thousands of photos to make a purchase decision. At the end of the day though, the function of a home rental is almost always a place for sleep and leisure. While people may have very different preferences for a type of property, there aren’t that many different ways in which someone will use a property.
Both of these examples share commonalities. There are a small number of buyer personas, and for the most part, the sellers’ products within a category are highly interchangeable. In both of these examples, the sellers’ products are fairly simple to group together in ways that the buyer will understand with little to no education. When a buyer looks at a product listing, it’s simple to understand the benefit it provides, as most of the information required to make a purchase decision is quickly digestible. This template is shared across large numbers of markets: event tickets, medical services, restaurant reservations, etc.
Data Marketplaces are the exception
Data offers a massive departure from the archetypical marketplace for a few reasons. First, the number of buyer personas is massive. Just to name a few of them: you have product managers looking for data to power a product feature, marketers looking for consumer audiences, investors looking for data on hundreds of extremely different markets, insurance actuaries trying to measure obscure risks, telcos trying to track competitors, etc. The list is nearly infinite. These buyers have thousands of different titles and work across every industry.

Adding to the buyer complexity, the selling side of the data market is even more complex. Data products don’t neatly fit into categories like home services do. Imagine a dataset that is a collection of all network traffic across private corporate networks across the world. This data could be transformed in an almost infinite number of ways:
1. Filter the traffic to web traffic and aggregate by website and you have a web traffic dataset
2. Filter the traffic to popular accounting software URLs and you have an accounting software market share tracker
3. Aggregate by headers in the network packets and you have a network hardware tracker
4. Correlate with a database of malicious traffic types and you have a cybersecurity risk index dataset
So what category of data is this underlying data? Is it web traffic, hardware data or cybersecurity data? And if you pick one of these categories, is the end user going to know to look for it there? What if this is only one dataset of many that a data seller produces? How do you classify the seller overall?
The final significant challenge is that even the most detailed description of a data product often isn’t enough for a buyer to have high confidence that the product meets their needs. Because use cases for data are so varied, without extensive testing as well as knowledge of different ways to apply data, it’s challenging to know if a data product meets the buyers’ need based solely on a one-page description of the data.
Data Market Structure
Within this global data market, where buyers have no single persona and the data itself is difficult to classify and summarize, how has the market structure settled? Sub optimally. It has ended up highly fragmented with different marketplaces targeting different buyer personas. Healthcare marketplaces for healthcare data buyers, finance data marketplaces for financial data buyers, real estate data marketplaces for real estate data buyers. Data vendors have also been forced to simplify their message to target a narrower set of use cases to make it more understandable. So, problem solved right? Not exactly.
Marketplace Verticalization
The issues with verticalized data marketplaces are severalfold. First there are still a multitude of personas within a finance data marketplace. You have financial analysts, data engineers, data scientists, quantitative developers and many more. These different personas don’t all speak the same language when it comes to data and their use cases can also vary significantly. Some data marketplaces cater to people that understand SQL, others to people looking for API data and yet others to people looking for research reports based on data. Despite the variability, this is actually the smaller problem. The larger problem is that the data vendors still don’t neatly fit into these marketplaces. A data vendor needs to decide whether they are areal estate vendor or a finance data vendor. They then need to build sales collateral and a go to market motion around a small set of verticals because trying to be every solution to everyone is a difficult proposition for most companies.
The last and biggest problem with the data market, even after you force the vendors into a single vertical and you have them target a single buyer persona is discovery. Now, in a verticalized marketplace, you end up with let’s say 100 real estate data vendors selling to the same types of real estate buyers, but the reality is that although the marketing materials for these 100 will sound very similar, they are actually quite different. Once each vendor attempts to compress their complex data offering into a paragraph or two of text, no one can tell the difference. This leads data-buying firms to build out large teams of people to wade through all of these vendors, conduct calls with them to learn as much about the data as possible, to try to figure out which will help in a particular situation. The problem here is that the datasets themselves are changing over time, as are the people sourcing the data. Institutional knowledge is being constantly lost both through attrition as well as the natural evolution of the data.
A Better Data Marketplace, again
Despite these clear failings, there seems to be no end to the number of companies launching new lists of datasets to “help”. Just as the Internet outgrew the list-based discovery approach (think AOL and Yahoo), so too has the data market. In my previous business, Adaptive Management, we also thought we could make a better list. We’d add hundreds of vendors, dozens of filters and keyword searching. Just as had been experienced by the marketplaces before us, this approach didn’t work. Despite all the fancy tools no one could find what they were looking for unless they were looking for something so obvious that a search engine would be the best way to find it. The descriptions of the vendors were just too short, too narrow to fully capture what the potential of each product was.
Solving the Data Market correctly
So how can this be solved? When starting Nomad Data, our goal was to solve this discovery issue which was ultimately leading to a fracturing of the data market. We first spoke to dozens of different buyer personas to understand exactly what was wrong with lists of data. They all said the same thing. They had a very tough time knowing if the data vendor whose description they were reading was actually a fit for what they needed. The descriptions were not specific enough to their problem. What they wanted was a search engine where they could explain in natural language the data they needed, and then be shown the matching data. This of course was easier said than done. The main reasons why this is hard to do:
1. People do a poor job explaining what they are looking for
2. Few, if any, data vendors have an exhaustive list of use cases for how their data can be used. Typically, they have a handful at best and hope their clients will figure the rest out
3. The vendors’ products are constantly evolving. The suite expands, the coverage changes, so any website or list cataloging this data will quickly become stale
4. It’s extremely hard to figure out the use cases of a dataset just by looking at the schema or raw data
We realized the only way to make natural language work for data discovery is if we could somehow crowdsource the information we needed about all the world’s data vendors in a way that we were constantly learning. We needed to couple this with human intelligence from our end to prime the pump so to speak.
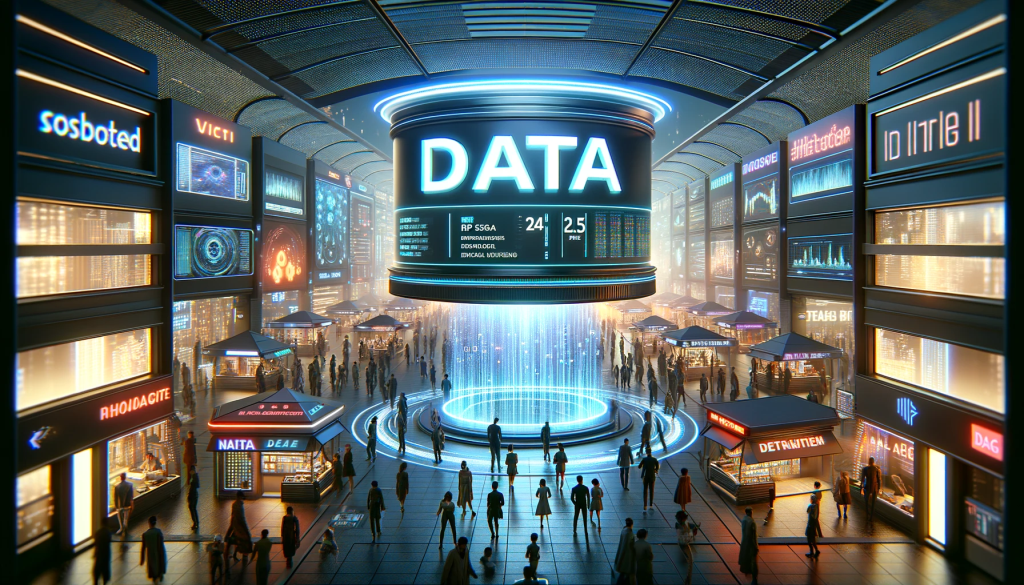
Data requires a new type of marketplace
In Nomad’s reinvented data search, users first describe in natural language the data they require: “I need a dataset of all public works projects ongoing across the world. I need it updated monthly and it must have project name, start date and expected completion date.” We then use a well-trained AI model to evaluate the request itself to make sure it is well formed and clearly explains what is needed. We then use a custom-built AI matching engine to compare the information we know about every data vendor and its data to this request.
For the top-ranking matches, Nomad sends an automated email to the vendors, showing them the actual request and asking them to state whether they have the needed data and how it can help the client. This requires a human on the vendor side to effectively train Nomad Data about the data they have. In many cases, the vendors themselves are not even sure if their data can meet the need. They often end up doing internal research. With each request, Nomad Data is learning new information about a data vendor. As this scales across all data searches, Nomad is continuously learning about the world’s data, preventing the internal catalog from getting stale.
Nomad’s approach dramatically reduces the friction to locate data. More importantly, it doesn’t force data vendors to “choose” a vertical or category for their data at the detriment of other opportunities. Nomad helps vendors learn about use cases they had never imagined for their data. Many companies new to selling data will even provide Nomad with information about data they may sell in the future, in order to see the matching requests, which ultimately help them choose a direction to start their data monetization journey.
The way data discovery works at Nomad is not dissimilar to how a traditional search engine works. When a user looks for something in a search engine, the site does its best to order results by relevance. For obscure things, the searcher will scroll through many pages of results to find the one they are looking for. As soon as the user clicks, they are taken to the site, but they’ve also just trained the search engine what the right answer was to a particular query. As the volume on the search engine grows, it builds up a data moat. This data is the core asset of a search engine. Others may come along with their own search engine, but without similar usage volumes, their results will be inferior. The same is true in data, except the queries are actually far more complex (ie. they can’t be distilled down to a simple phrase),which is why it requires a similar mechanism for learning.
Conclusion
Despite the fact that data lists don’t work, we will continue to see new ones pop up. A big trend we’ve seen recently is companies building their own internal lists. Lists of data they own, create, or buy. We see company after company running into the same issues of discovery internally. Even the most sophisticated corporate business users report difficulty finding the data they need.
As new models of discovery evolve we will hopefully see the birth of a successful cross-industry data marketplace. The data market, more than most, needs standardization. Because there is no central actor to impose, or even suggest standards for things like pricing, delivery mechanism and schema, it adds massive cost for everyone involved. When a company has aggregated significant data buying scale across industries, and can start to push broad standards, you will see costs collapse and volumes explode. This will allow the true potential of data to be achieved, and it will help foster an even richer ecosystem of data types and tools.