Building Type Identification Data
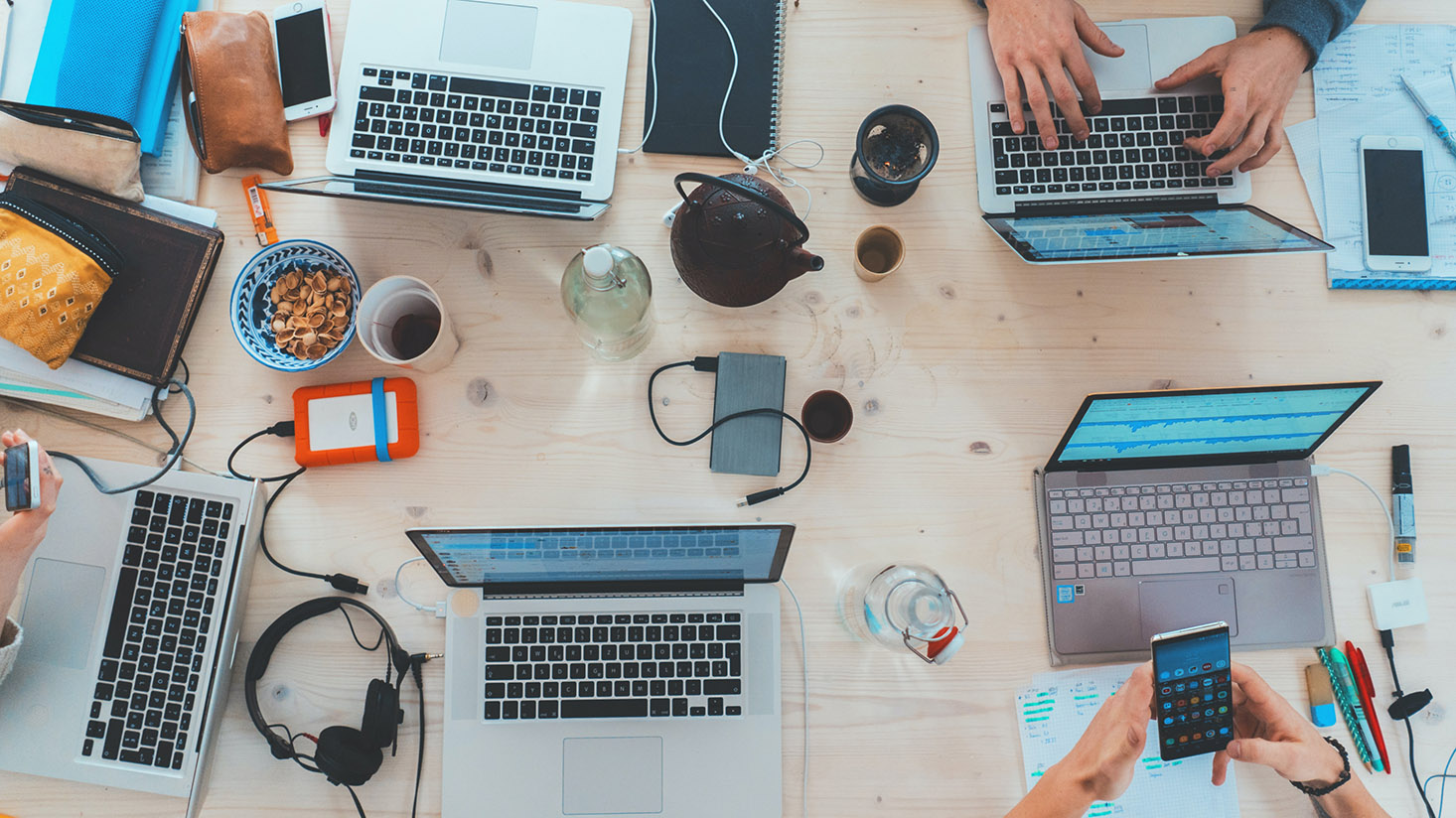
Introduction
Understanding the type of building located at a specific address has historically been a challenging task. Before the digital age, this information was gathered through manual surveys, public records, and local knowledge, methods that were time-consuming and often inaccurate. The advent of sensors, the internet, and connected devices, along with the proliferation of software and databases, has revolutionized the way we gather and analyze data about buildings. This transformation has enabled professionals across various industries to obtain real-time insights into building types, ranging from residential properties like apartments and condominiums to commercial spaces such as retail strips and shopping malls.
The importance of data in identifying building types cannot be overstated. Previously, individuals and businesses were in the dark, waiting weeks or months to understand changes in the landscape of urban and suburban areas. Now, with the right data, changes can be understood in real-time, allowing for more informed decision-making. This shift has been particularly beneficial in Canada, where the diversity of building types presents unique challenges and opportunities.
Historically, the reliance on antiquated methods meant that much of the decision-making was based on incomplete or outdated information. The introduction of connected devices and the internet has paved the way for the collection of vast amounts of data, making it easier to categorize and understand the types of buildings at any given location. This article will explore how specific categories of datasets can provide better insights into identifying building types, a crucial aspect for various business professionals.
Geolocation Data
Geolocation data has become an invaluable resource in identifying building types. With the ability to match addresses to points of interest (POIs) and analyze foot traffic patterns, geolocation data providers offer a comprehensive view of the types of buildings found at specific locations. This data category has seen significant technological advancements, allowing for the categorization of buildings with unprecedented accuracy.
- History of Geolocation Data: The evolution of geolocation technology, from basic GPS tracking to the development of sophisticated POI databases, has been instrumental in its ability to provide insights into building types.
- Examples of Geolocation Data: Databases containing millions of POIs, including building footprints and exact polygons belonging to thousands of brands, are examples of how geolocation data can be utilized.
- Industries and Roles: Real estate developers, urban planners, and retail strategists are among the professionals who benefit from geolocation data to make informed decisions.
- Technology Advances: The integration of AI and machine learning with geolocation data has accelerated its usefulness, enabling dynamic categorization of buildings based on foot traffic and other patterns.
Specific uses of geolocation data in identifying building types include:
- Matching addresses to detailed POI databases to determine the building category.
- Analyzing foot traffic patterns to distinguish between commercial and residential buildings.
- Utilizing unique datasets for live demonstrations to showcase the capabilities of geolocation data in real-time building identification.
Real Estate Data
Real estate data provides another layer of insight into building types. By tracking commercial properties across Canada, real estate data providers offer a wealth of information that can be used to categorize buildings effectively.
- History of Real Estate Data: The collection of real estate data has evolved from manual property surveys to comprehensive digital databases that include detailed information about commercial properties.
- Examples of Real Estate Data: Digital systems that compile information on commercial properties, allowing users to pull data in various formats for analysis.
- Industries and Roles: Investors, market researchers, and commercial real estate professionals rely on real estate data to understand market trends and property types.
- Technology Advances: The digitization of property records and the development of online platforms have made real estate data more accessible and actionable.
Real estate data can be specifically used to:
- Identify commercial property types across Canada, aiding in market analysis and investment decisions.
- Compile and analyze data on commercial properties to understand trends and categorize buildings.
Web Scraping Data
Web scraping data providers offer a unique approach to identifying building types by extracting structural building information from online sources. This data type complements geolocation and real estate data by providing additional layers of detail.
- History of Web Scraping Data: Web scraping has grown from simple data extraction techniques to sophisticated methods that gather detailed information from a variety of online sources.
- Examples of Web Scraping Data: Structural building information extracted from property, building, and COPE data sources, offering a comprehensive view of building types.
- Industries and Roles: Data analysts, urban planners, and real estate developers use web scraping data to enhance their understanding of building structures and types.
- Technology Advances: The advancement of web scraping technologies and algorithms has enabled the extraction of more accurate and relevant data.
Web scraping data can be used to:
- Extract structural information about buildings, providing insights into their types and uses.
- Join property data with other relevant datasets to offer a more comprehensive analysis of building types.
Conclusion
The importance of data in identifying building types cannot be understated. With access to geolocation, real estate, and web scraping data, business professionals can gain better insights into the types of buildings found at specific addresses. This information is crucial for making informed decisions in various industries, including real estate, urban planning, and retail strategy.
As organizations become more data-driven, the discovery and utilization of diverse data types will be critical to understanding complex topics like building identification. The future may see the monetization of new data types, providing additional insights into building types and further enhancing decision-making processes.
The evolution of data collection and analysis technologies promises to unlock the value hidden in decades-old documents and modern government filings. With the help of AI and machine learning, the potential to categorize and understand building types on a granular level is becoming a reality, offering exciting opportunities for professionals across various industries.
Appendix
Industries and roles that could benefit from building type identification data include investors, consultants, insurance companies, market researchers, and urban planners. These professionals face challenges in understanding the dynamic real estate market and making strategic decisions based on accurate information.
Data has transformed these industries by providing insights that were previously inaccessible, enabling a deeper understanding of market trends and building types. As the future unfolds, AI and machine learning are poised to further revolutionize the way we analyze and interpret data, unlocking new possibilities for innovation and efficiency.