Commodity Cost Insights
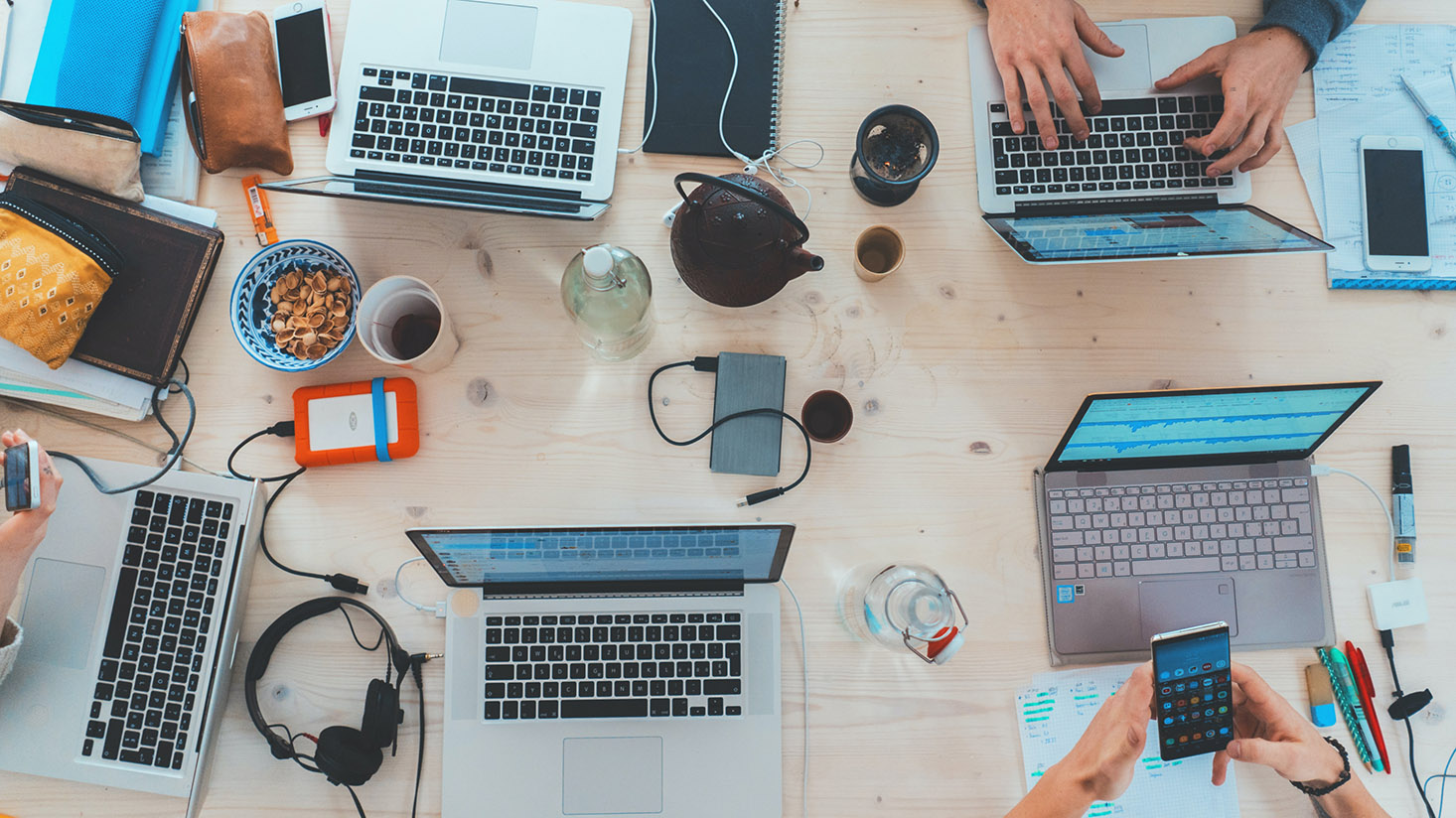
Introduction
Understanding the cost of inputs and their sensitivity on finished products has always been a complex challenge for businesses across various sectors. Historically, firms relied on antiquated methods to gauge these costs, often resulting in delayed and less accurate insights. Before the advent of sophisticated data collection and analysis methods, companies had to rely on manual surveys, expert opinions, and rudimentary financial reports to estimate the impact of commodity prices on their operations. This often meant operating in the dark, with decisions based on outdated or speculative information, leading to inefficiencies and missed opportunities.
The introduction of sensors, the internet, and connected devices has revolutionized the way businesses access and utilize data. The proliferation of software and the digital storage of events and transactions have made it possible to track and analyze data in real-time. This technological advancement has provided firms with the ability to understand changes in commodity costs and their impact on finished products with unprecedented speed and accuracy.
The importance of data in navigating these complexities cannot be overstated. In the past, businesses would wait weeks or months to understand shifts in commodity prices and their effects on production costs and margins. Today, with the right data, companies can monitor these changes in real-time, allowing for more informed decision-making and strategic planning. This shift towards data-driven insights represents a significant leap forward in how businesses manage their input costs and assess their impact on product pricing and profitability.
Financial Data Insights
One of the key data types that have emerged to address these challenges is financial data. Financial data providers, leveraging AI-driven commodity market insights, have transformed the landscape of cost analysis. Through the use of Natural Language Processing (NLP) technology, these providers collect, clean, and normalize various non-structured data, offering businesses a comprehensive view of market trends and commodity price fluctuations.
Historically, financial data was limited to structured formats such as stock prices and exchange rates. However, the technology advances in AI and NLP have enabled the extraction of valuable insights from a wide range of sources, including news articles, financial reports, and social media. This expansion of data sources has significantly increased the volume and variety of data available, accelerating the pace at which businesses can gain insights into commodity costs.
Industries such as manufacturing, automotive, and technology have historically used financial data to inform their procurement and pricing strategies. The ability to analyze commodity market trends and price sensitivity allows these sectors to better manage their input costs, optimize their supply chains, and adjust their product pricing in response to market changes.
Specifically, financial data can be used to:
- Track real-time commodity prices
- Analyze historical price trends
- Forecast future price movements
- Assess the impact of geopolitical events on commodity markets
For example, in the automotive sector, understanding the sensitivity of auto production costs and margins to changes in palladium prices is crucial. Financial data provides the insights needed to navigate these complexities, enabling firms to make strategic decisions that enhance their competitiveness and profitability.
Technology Data Insights
Technology data providers offer another critical perspective on understanding the cost of inputs and their impact on finished products. By providing detailed information on components and their pricing, these providers help businesses align their needs and requirements with market realities. The evolution of technology data has been closely tied to the advancements in manufacturing and supply chain management software, which have made it easier to track and analyze component costs and availability.
Historically, technology data was primarily used by industries involved in electronics manufacturing and IT infrastructure development. However, as the importance of technology components in a wide range of products has grown, so has the use of this data across various sectors. The ability to access detailed component categories and pricing information has become a key factor in managing production costs and optimizing product design.
Technology data can be used to:
- Identify cost-effective components
- Monitor supply chain disruptions
- Optimize product design for cost efficiency
- Forecast component price trends
For instance, in the development of new electronic devices, understanding the cost and availability of specific components can significantly influence design decisions and production timelines. Technology data provides the insights necessary for these strategic considerations, contributing to more efficient and cost-effective product development.
Automotive Data Insights
The automotive sector, in particular, has benefited from specialized data insights related to the cost of inputs and the sensitivity of finished products to these costs. Automotive data providers offer expertise, datasets, and content that cover a wide range of factors influencing production costs and margins. This includes insights into material costs, labor expenses, and the impact of regulatory changes on the automotive industry.
Historically, automotive data was used primarily by manufacturers and suppliers to inform production planning and cost management. However, as the automotive industry has evolved, the use of this data has expanded to include financial analysts, investors, and regulatory bodies. The ability to access detailed insights into the cost of inputs and their impact on product pricing and profitability has become a critical competitive advantage in the automotive sector.
Automotive data can be used to:
- Analyze the impact of material cost fluctuations on production costs
- Assess the sensitivity of product margins to changes in input costs
- Inform strategic sourcing and procurement decisions
- Forecast industry trends and regulatory impacts
For example, understanding the sensitivity of auto production costs to changes in palladium prices is essential for automotive manufacturers. Automotive data provides the comprehensive insights needed to navigate these challenges, enabling firms to optimize their production processes and pricing strategies.
Conclusion
The importance of data in understanding the cost of inputs and their sensitivity on finished products cannot be overstated. Access to diverse types of data, including financial, technology, and automotive data, has empowered businesses to gain real-time insights into commodity prices and market trends. This has enabled more informed decision-making, strategic planning, and competitive positioning across various industries.
As organizations become increasingly data-driven, the discovery and utilization of relevant data will be critical to their success. The ability to analyze and interpret data related to input costs and product pricing will continue to be a key factor in managing profitability and achieving business objectives.
Looking ahead, corporations are likely to explore new opportunities to monetize the valuable data they have been creating for decades. This could lead to the emergence of new types of data that provide additional insights into the cost of inputs and their impact on finished products. As these data sources evolve, businesses will have even greater opportunities to enhance their understanding of market dynamics and optimize their operations accordingly.
Appendix
Industries and roles that could benefit from access to data on the cost of inputs and their sensitivity on finished products include investors, consultants, insurance companies, market researchers, and more. These stakeholders face various challenges, such as managing risk, optimizing investment strategies, and understanding market trends. Data has transformed these industries by providing actionable insights that inform decision-making and strategic planning.
The future of data utilization in these sectors is promising, with advancements in AI and machine learning poised to unlock the value hidden in decades-old documents and modern government filings. As technology continues to evolve, the potential for data to drive innovation and efficiency across industries is limitless.