Consumer Risk Score Insights
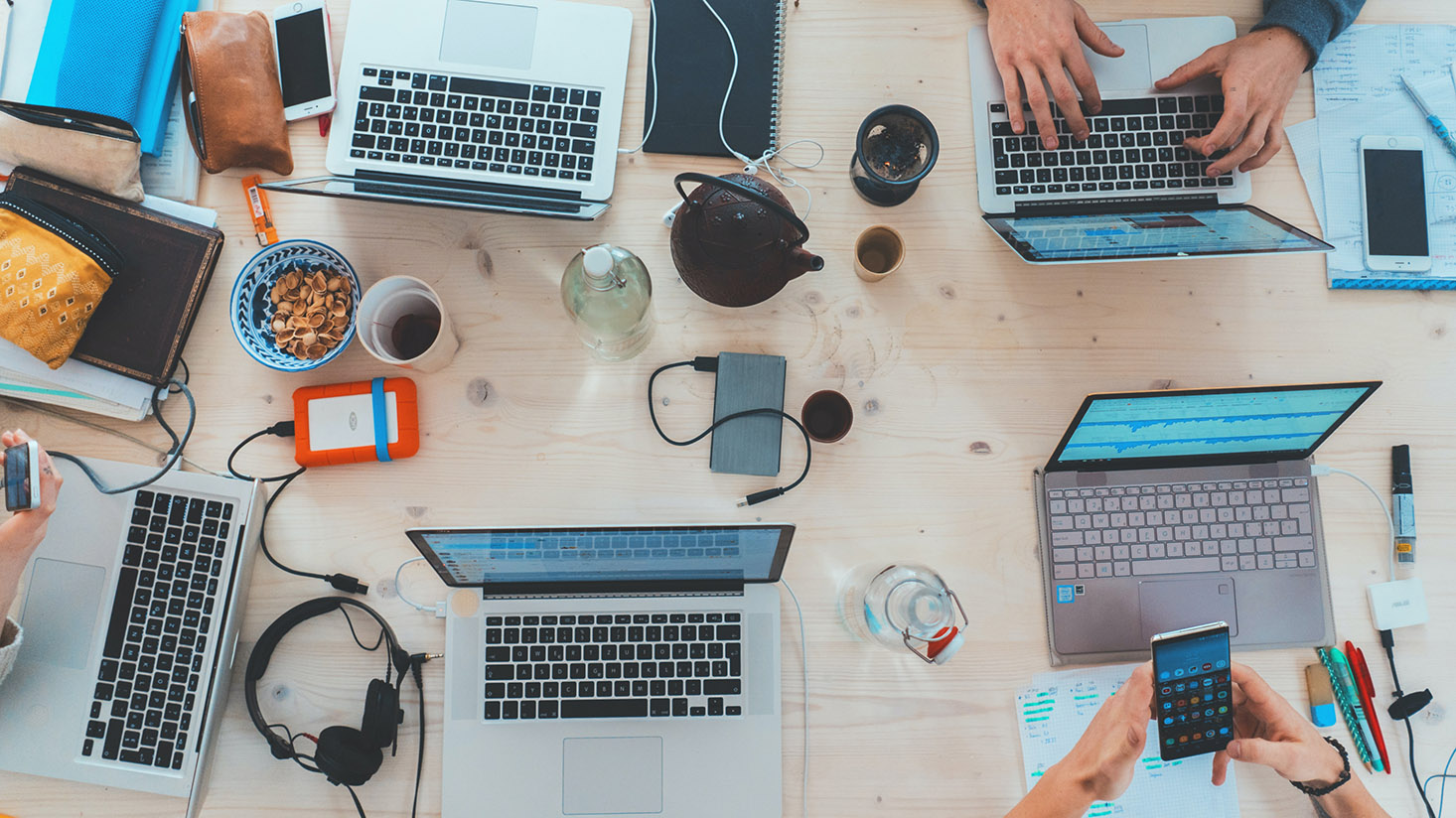
Introduction
Understanding consumer behavior and assessing risk have always been critical components of financial decision-making. Historically, this process was fraught with challenges, as firms relied on antiquated methods to gauge consumer reliability. Before the digital revolution, insights were primarily derived from limited sources such as payment histories on loans and credit cards, with many consumers remaining 'invisible' due to a lack of traditional credit history. In the era before comprehensive data collection, businesses often operated in the dark, making decisions based on incomplete information and waiting weeks or months to understand market changes.
The advent of the internet, connected devices, and the proliferation of software into many processes has dramatically transformed this landscape. Now, every transaction and interaction can be stored, analyzed, and leveraged for insights. This shift towards a data-driven approach has enabled real-time analysis, shedding light on consumer behavior and risk in ways previously unimaginable.
The importance of data in understanding consumer risk cannot be overstated. With the right datasets, businesses can now access a wealth of information that was once out of reach, allowing for more accurate risk models and informed decision-making. This article will explore how specific categories of datasets, such as credit data, transaction data, and consumer behavior data, can provide invaluable insights into consumer risk scores.
Credit Data
The role of credit data in understanding consumer risk is well-established. Traditionally, this data has been sourced from credit bureaus, offering insights based on loan repayments, credit card usage, and other financial obligations. However, the landscape of credit data is evolving, with alternative scores based on smartphone and web behavioral metadata emerging as powerful tools. These alternative scores offer insights into the 'unscorable' population, bridging the gap between traditional credit reports and the modern consumer's digital footprint.
- Examples of Credit Data: Alternative scores, traditional credit reports, transactional data.
- Industries Benefiting: Financial services, lending institutions, retail banking.
- Technology Advances: The use of smartphone and web behavioral metadata to derive alternative credit scores.
- Usage: Enhancing risk models, identifying creditworthy individuals among unscorable populations.
Transaction Data
Transaction data provides a direct window into consumer spending habits, offering insights that go beyond traditional credit data. By analyzing payments to utility companies and overall spending patterns, businesses can gain a nuanced understanding of consumer financial health and behavior. This data type is particularly valuable for developing comprehensive risk models that account for everyday financial activities.
- Examples of Transaction Data: Payments to utility companies, complete spend profiles.
- Industries Benefiting: Financial technology, consumer lending, utility providers.
- Technology Advances: The ability to aggregate and analyze bank transaction data.
- Usage: Developing nuanced risk models, understanding consumer spending habits.
Consumer Behavior Data
Consumer behavior data, particularly from non-traditional sources like telecommunications and utility payments, offers a rich source of insights into consumer reliability and risk. With access to vast databases of active records, businesses can now consider a broader spectrum of consumer activities when assessing risk. This data is especially crucial for identifying creditworthy individuals among those with thin or no traditional credit files.
- Examples of Consumer Behavior Data: Telecommunications, pay TV, and utility payment histories.
- Industries Benefiting: Credit scoring agencies, telecommunications, utilities.
- Technology Advances: The collection and analysis of non-traditional payment data.
- Usage: Expanding the scope of risk assessment, improving access to credit for underserved populations.
Conclusion
The integration of diverse data types into the assessment of consumer risk represents a significant advancement in financial decision-making. By leveraging credit data, transaction data, and consumer behavior data, businesses can develop more accurate and inclusive risk models. This data-driven approach not only enhances understanding but also facilitates real-time decision-making, allowing for a more dynamic response to market changes.
As organizations continue to become more data-driven, the importance of data discovery in understanding consumer risk and behavior will only grow. The future of risk assessment lies in the ability to harness and analyze a wide range of data sources, potentially including new types of data that companies are only beginning to explore.
The evolution of data collection and analysis technologies promises to unlock even greater insights, with artificial intelligence playing a key role in deciphering patterns hidden within decades-old documents or modern digital interactions. As we move forward, the ability to understand and predict consumer behavior and risk will increasingly rely on the strategic use of data.
Appendix
Industries and roles that stand to benefit from advancements in consumer risk data include investors, consultants, insurance companies, market researchers, and more. These stakeholders face the common challenge of accurately assessing consumer risk and behavior, a task that has been transformed by the availability of comprehensive data.
The future of these industries lies in the continued integration of data into decision-making processes, with AI and machine learning offering new avenues for unlocking the value hidden within vast datasets. As data becomes more central to understanding consumer risk, the potential for innovation and improved outcomes across sectors is immense.