Empower AI Models with Robust English to ASL Data
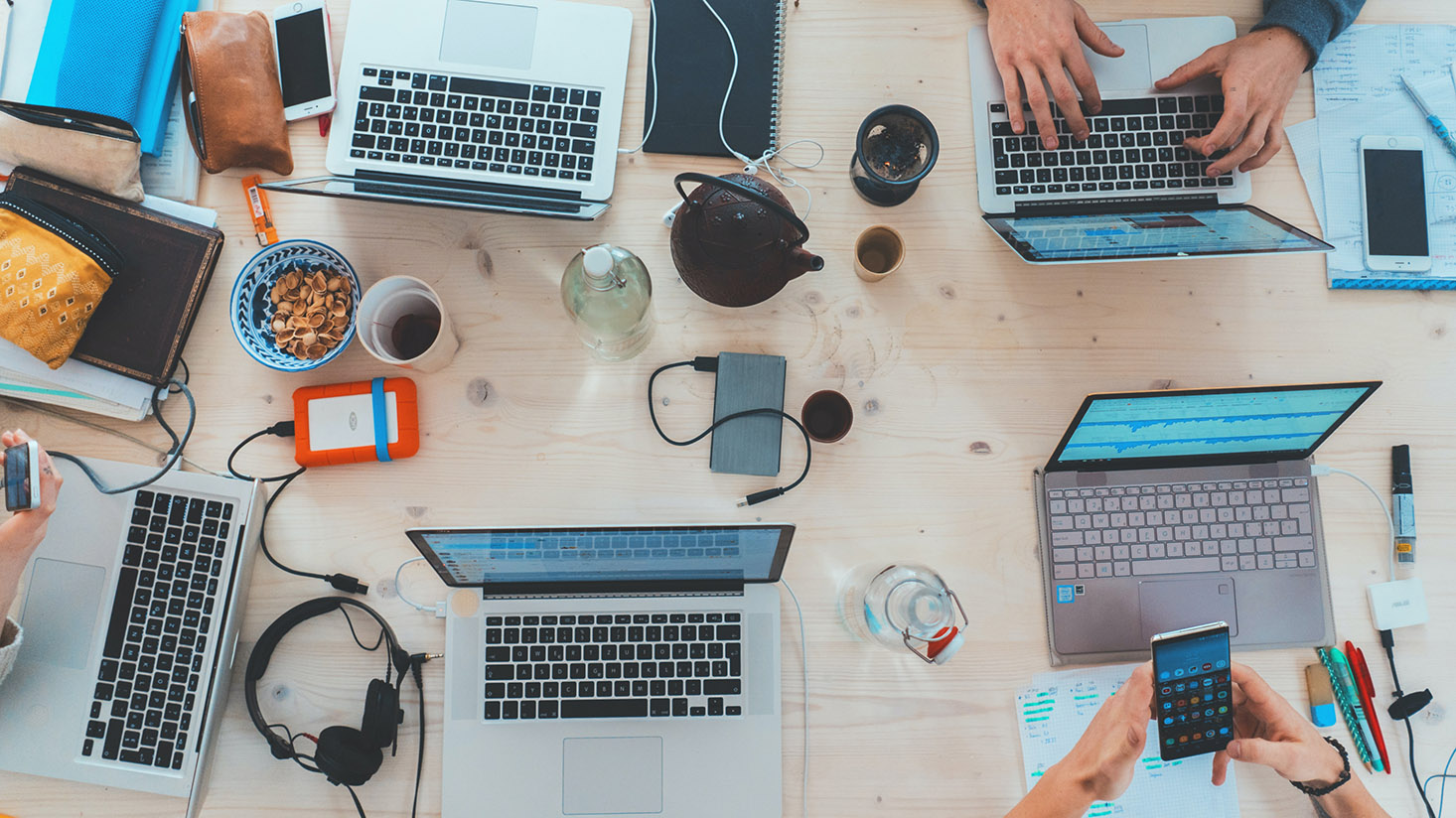
Empower AI Models with Robust English to ASL Data
Introduction
In the dynamic landscape of artificial intelligence, translating spoken or written English into American Sign Language (ASL) has always been a daunting challenge. Historically, this process relied heavily on manual interpretations and the expertise of individuals well-versed in both languages. The limited availability of automated resources made it arduous to achieve high accuracy in translation, often leading to significant delays and discrepancies in communication. Organizations lacked the necessary tools to provide instantaneous, accurate translations, thereby hindering the efficacy of ASL as an inclusive communication medium.
Fortunes have changed with the advent of digital technologies. The introduction of AI and machine learning has made it possible to understand and process languages at an unprecedented scale. Deep learning models, powered by vast datasets, have the capability to recognize and translate languages, including ASL, with greater precision. However, the cornerstone of any successful AI application, especially in language translation, is comprehensive training data.
Previously, organizations depended on limited datasets, often containing outdated or inconsistent data. The reliance was on rudimentary methods such as static dictionaries or basic gesture illustrations which could not adapt to the linguistic nuances of ASL. While these resources provided some aid, the lack of context and fluidity rendered them inadequate for real-time translation needs. It wasn't until the role of external training datasets was recognized that substantial progress was made, emphasizing the need for annotated videos and labeled data to fuel model understanding.
Now, through robust datasets that encompass a variety of signing nuances, organizations can gain real-time insights into ASL communication dynamics. The digital age has ushered in an era where devices, sensors, and external data search serve as catalysts for meaningful data acquisition. This shift has equipped AI systems with the ability to perform more nuanced translations swiftly and accurately, eliminating the guesswork that once plagued the process.
The implementation of integrated software solutions has further bolstered efforts to address the gaps in ASL translation. These innovations have unleashed unprecedented opportunities to automate and enhance sign language interpretation experiences, leading to more inclusive interactions across various sectors. However, the foundation for these strides is the availability of multifaceted datasets that serve as the backbone of intelligent AI systems.
Access to comprehensive training data means organizations are no longer in the dark, waiting weeks or months to analyze the effectiveness of their models. Today, with access to real-time datasets, professionals can make informed adjustments instantaneously, paving the way for more effective communication strategies and fortified connection standards between diverse communities.
AI Training Data
Historical Context
The evolution of AI training data is a journey marked by transformative technological strides. Initially, the data used to train early AI models in sign language were sparse and consisted primarily of isolated signs captured in controlled environments. Over time, advancements in both recording technology and computational methodologies have enriched these datasets, moving from simple motion captures to intricate sign language annotations that capture complex syntactical and contextual elements.
Examples and Applications
AI training data relevant to English to ASL translation encompasses diverse sources, offering intricate visual cues essential for categories of data to ensure accuracy. Examples include:
- Signbanks: Platforms like the Yale University ASL Signbank and Rutgers University Sign Bank provide expansive collections of annotated ASL videos. These resources play a critical role in training algorithms to understand linguistic nuances and regional variations in ASL.
- Gesture Recognition Datasets: These include vast collections of images and video clips that account for a wide range of gestures, lighting conditions, and viewing angles. Such diversity allows AI models to be trained to recognize gestures across different environments.
- High-Tech Multimodal Datasets: Resources like How2Sign incorporate holistic data capturing video, audio, and 3D pose estimations. This multimodal approach enriches training datasets, providing AI models with a deeper contextual understanding.
Industry and Technological Advances
Diverse industries, from educational institutions to tech innovators, have historically sought robust datasets to improve ASL communication. The continued progress in the technology that supports data collection, such as high-resolution cameras and sophisticated annotation software, has catalyzed the growth and depth of these datasets, making them more accessible and easier to integrate into AI systems.
Accelerating Data Availability
The acceleration in the availability of training data for AI models is pivotal to advancing ASL translation. With technological innovations reducing storage costs and increasing processing power, datasets have exponentially grown in size and scope. The accumulation of comprehensive video datasets, enriched annotations, and advanced metadata has turbocharged deep learning capabilities.
Impact on Understanding English to ASL Translation
These datasets empower organizations to fine-tune models, enabling them to perceive subtle elements of ASL. Five critical contributions include:
- Improved Accuracy: Rich, annotated video datasets facilitate better model training, resulting in highly accurate translations.
- Enhanced Contextual Interpretation: Multimodal data provide contextual insights beyond mere gesture recognition, an element crucial for translations.
- Increased Responsiveness: Real-time dataset accessibility ensures continuous updates and improvements to model outputs.
- Greater Inclusivity: Broad gesture datasets capture wide arrays of signing nuances, accommodating varied signing styles and regional differences.
- Effortless Integration: Standardized annotation practices enable seamless dataset integration into varied AI frameworks.
Conclusion
Throughout this article, we’ve illustrated the pivotal role data plays in advancing English to ASL translation. As highlighted, the development of robust datasets is crucial for AI models aiming to bridge communication gaps between English speakers and the deaf or hearing-impaired community. By harnessing the power of AI through well-structured datasets, companies can offer more inclusive solutions and foster greater understanding.
Organizations that master data discovery and embrace a data-driven culture will continue to lead in innovative language solutions. The increasing focus on data monetization suggests a future where valuable insights can be drawn from untapped resources. As companies transition toward becoming more data-centric, the availability of diversified datasets will provide unparalleled advantages.
Looking ahead, the possibilities for new data types are endless. From enhanced biometric data capturing emotional undertones during signing to augmented reality datasets that can facilitate real-time translations, the future holds promise for even more sophisticated AI-driven translations.
Data is indeed the lifeline for innovation, and as organizations refine their approaches to capturing and utilizing data, the horizon expands for more impactful applications. AI’s efficacy is anchored in its ability to learn from detailed datasets, driving communication forward into an era of inclusion and understanding.
The future of AI in language translation is undoubtedly bright, with organizations continually striving to improve data quality and diversity. By adopting a forward-thinking approach to data management and exploration, businesses can unlock the potential of AI to foster richer interactions across languages.
Appendix
Who Benefits from ASL Training Data?
The impact of AI-powered ASL translation extends across multiple industries and roles:
- Investors: Investing in AI-driven language solutions opens avenues for substantial returns in an era demanding inclusivity.
- Consultants: Consultants can harness data insights to advise clients on strategically implementing AI-powered translation tools.
- Educational Institutions: Schools and universities can adopt these technologies to create inclusive learning environments for ASL users.
- Healthcare Providers: AI-enhanced communication tools ensure seamless interactions with ASL users, improving patient care and experience.
- Market Researchers: Researchers can explore emerging trends in communication technologies, aiding in the development of future solutions.
Transforming Industries with AI and ASL
Data has transformed industries by addressing complex communication challenges faced by ASL users. With the integration of AI-driven translation tools, barriers are reduced, fostering a more inclusive society. Problems that once plagued the industry, such as misinterpretations and inconsistencies, are now systematically addressed through data-centric solutions.
Businesses across sectors have realized that AI combined with comprehensive datasets can streamline operations and enhance user experiences. Breakthroughs like real-time translations have become integral to brand differentiation, demonstrating value to consumers.
As we forge ahead, the role of AI is expanding, with the potential to unlock valuable insights from historical records and modern data sources. Domains such as finance are already witnessing enhancements through intelligent data processing, setting the stage for similar advancements in translation applications.
In the future, leveraging AI to capitalize on underutilized data assets could transform conventional applications into innovative, game-changing services.
Businesses that are forward-looking in their data strategies will be best positioned to harness AI's full potential, reinforcing their contributions to a more inclusive, communicative society.