Enhance Telco Strategy with Enrichment Data for Churn Prediction Insights
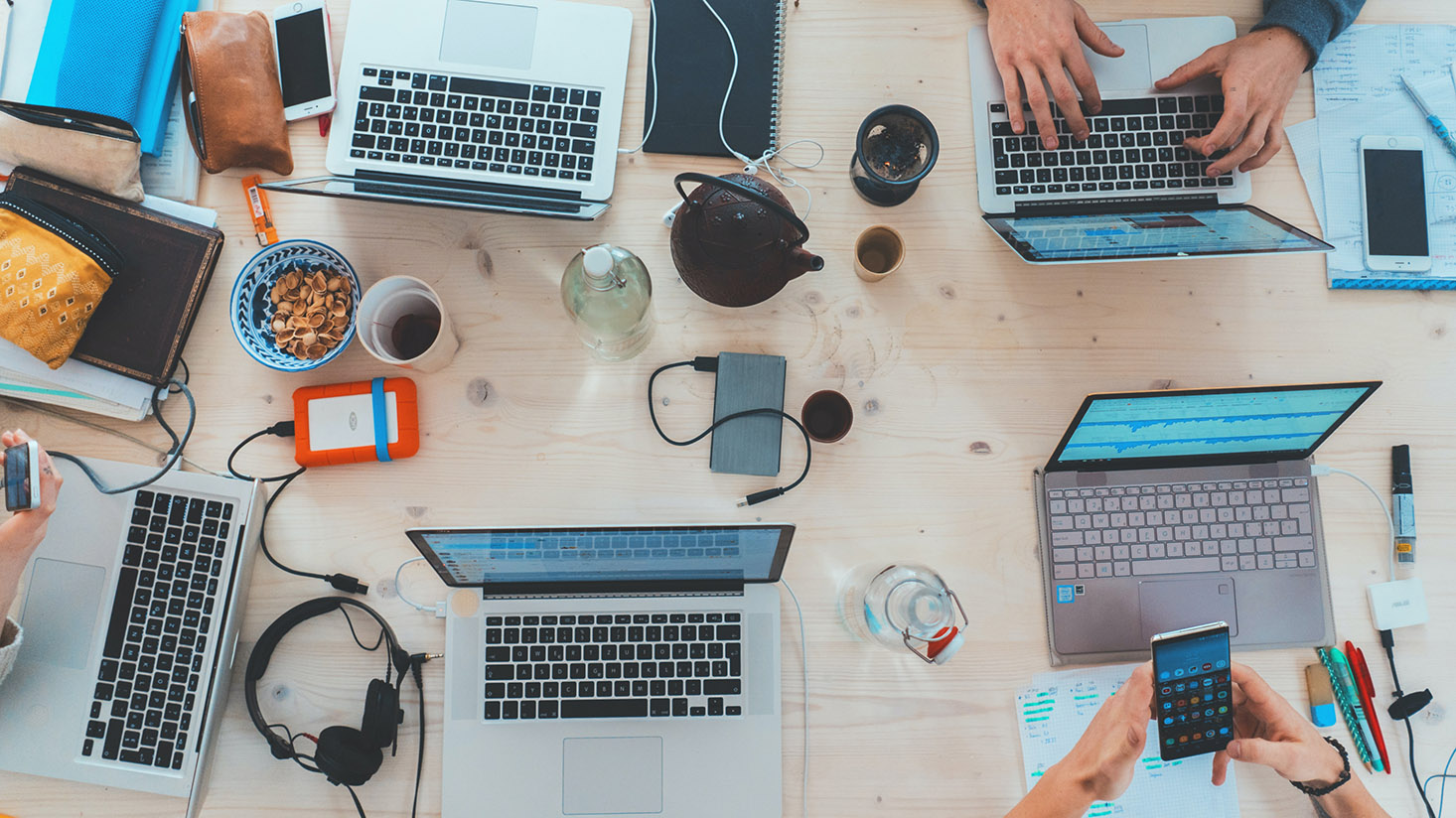
Introduction
The telecommunications industry, particularly in regions such as Brazil, faces a perennial challenge: customer churn. Understanding why subscribers switch operators, terminate their contracts, or alter their usage patterns has always been crucial for strategic planning and customer retention. Yet, historically, this insight has been elusive, a dark room in which telcos stumbled around waiting weeks or months for market surveys and quarterly reports. The early days involved relying on qualitative methods such as customer service feedback and basic billing data.
Before the proliferation of advanced data sources, many telecommunications firms relied on limited customer identifiers, like subscriber demographics captured at the point of sale or basic usage metrics extracted from billing systems. There was no cohesive or dynamic dataset that accurately reflected customer behavior or external influences that could affect their decision-making processes. The datasets were static, historical, and often months outdated, leaving operators with a narrow understanding of real-time market dynamics.
The advent of sensors, ubiquitous internet connectivity, and connected devices radically transformed data accessibility. We now store every keystroke, mouse click, and phone ping, aggregating a massive repository of actionable information in real-time. An era of unprecedented insight was birthed from this digital revolution, yielding opportunities for data-informed strategic actions and churn prediction models.
With the integration of types of data such as consumer demographics, identity, and behavioral datasets, the telecommunications industry finds itself better equipped to predict and manage churn. These data are not only used in isolation but amalgamated with proprietary telecom data to form a comprehensive, multi-dimensional view of every subscriber.
The ability for operators to correlate a user's cell phone activity with behavioral data, socio-economic status, and geographical movements is becoming the foundation for predicting shifts in consumer allegiance. Practically overnight, telcos have moved from operating in the past to gaining real-time insights that enhance competitiveness and customer satisfaction.
This article explores the numerous external data sources that hold the potential to enrich churn predictive analytics, turning a once speculative guessing game into a science-driven strategy for customer retention and engagement.
Consumer Behavior Data
Consumer behavior data offers a lens into the preferences and tendencies of individuals, going beyond mere transaction records. Historically, this information was sparse and predominantly gathered through painstaking surveys and market research initiatives by specialists. Fast forward to modern times, advancements in technology have led to an accumulation and refinement of behavioral data sources, greatly enhancing how businesses understand their consumer bases.
Nowadays, telcos can leverage expansive datasets that include behavioral metrics and lifestyle segmentation, giving a holistic overview of customer tendencies. Notably, companies that provide this type of data can often link these insights directly to subscriber phone numbers, effectively enabling telcos to map behavior patterns across communities and predictive models.
Roles and industries that have utilized consumer behavior data include marketing strategists aiming to optimize customer journeys, retail managers understanding shopping behaviors, and financial services evaluating creditworthiness through spending habits.
The explosive growth of social media, e-commerce, and digital marketing platforms has spurred a data fervor, where behavioral datasets now capture online interactions, purchase history, search intents, and even advertisement engagements. The ability to append this information to telecom client profiles enables more accurate churn prediction algorithms.
Utilizing Consumer Behavior Data for Churn Prediction
- Understanding Erasure: By observing customer engagement patterns and changes, operators can forecast the likelihood of churn when engagement drops signal diminishing interest or dissatisfaction.
- Sentiment Analysis: Knowing how customers feel about service offerings through behavioral feedback cues allows firms to intervene proactively in churn cases.
- Personalized Offers: Insights inform personalization strategies in marketing communications and customized offerings aimed at retaining flagging customers.
- Pattern Recognition: Overarching behavior patterns can be identified, targeting interventions at customers showcasing characteristics comparable to previous churners.
- Loyalty Improvements: By understanding what behaviors indicate contentment, telcos can craft strategic initiatives enhancing customer loyalty and retention.
People and Demographic Data
People and demographic data are indispensable components in gaining insights into churn potential. Historically, demographic information was confined to census data or public records, often considered static in nature providing a snapshot rather than a continuum of personal evolution.
In present times, the wealth of AI-driven platforms and administrative databases have opened a robust pool of demographic details ranging from age, gender, income levels, family size, employment status, and even corroborated resume information. Telcos can match phone numbers with enriched demographics, enhancing their understanding of the consumer landscape and predispositions towards switching operators.
This type of data is especially used by industries such as political campaigners organizing voter outreach, e-commerce platforms tailoring product recommendations, and insurance companies constructing risk profiles based on neighborhood and population stats.
Technology advancements have broadened the capture and aggregation of these data points, enabling more precise analytics and personalized interventions. In the churn prediction context, these datasets provide indispensable indicators of subscriber behavior, preferences, and likelihoods, illuminating the customer’s future actions.
How People and Demographic Data Aid Churn Prediction
- Segment Targeting: Granular demographics enable targeted loyalty campaigns to demographic segments more inclined to switch providers, such as young professionals or retirees.
- Predictive Modelling Enhancement: Demographic data use demographic regressions to forecast potential churn, refining models to account for socio-economic and lifestyle variances.
- Custom Tailored Packages: Operators can create customer packages specifically matching lifestyle and demographics, improving satisfaction and reducing churn.
- Risk Assessment: Identifying high-risk groups based on economic conditions, location, or age group enhances proactive retention strategies.
- Community Analytics: Aggregated trends of whole communities may reveal wider shifting patterns, enabling proactive community-focused interventions.
Identity Resolution Data
Identity resolution data – specializing in verifying and connecting disparate personal identifiers across databases – has been pivotal across industries for consolidating customer profiles and ensuring data reliability. Initially, this data was highly fragmented and challenging to unify, often existing in scattered formats across various systems, from customer records to public databases.
As technology evolved, so did identity resolution techniques, employing pattern recognition, machine learning, and expansive internet coverage to harmonize personal identifiers globally. The telecom sector can tap into identity resolution data to unify subscriber information with external behavior and demographic data-streams, producing a comprehensive, unified dataset.
Traditionally used by sectors keen on security and verification, such as financial services, law enforcement, and tech companies that require robust authentication protocols, identity resolution now also plays a pivotal role in customer analytics and personalized marketing.
This data category accelerates the depth of understanding regarding customer journeys, aura, and predictive behaviors tailored to anti-churn models, opening a robust avenue for scanning future intentions.
Applying Identity Resolution Data in Churn Strategies
- Profile Building: Merging various data sources into a singular, aligned customer profile facilitates enhanced predictive accuracy.
- Fraud Detection: Identifying potentially fraudulent or risky profiles through cross-verification curtails unforeseen losses and customer dissatisfaction.
- Behavioral Attribution: Recognizing behavioral tendencies attributed to authenticated profiles aids in designing targeted retention strategies.
- Interaction Mapping: Multi-channel interaction mapping through identity data gives an intricate view of customer transitions and identifies weak spots leading to churn.
- Security Strengthening: Enhanced identity verification bolsters trust and incentivizes customer loyalty and continued engagement with the telecom provider.
Conclusion
In the rapidly evolving realm of telecommunication, data stands as a pillar for strategic initiatives. Understanding customer churn, a significant concern for telecom operators, now leans heavily on the integration of varied data sources. Enhanced insights gained from consumer behavior, people demographics, and identity resolution datasets equip firms with predictive prowess and customer-centric strategies.
Corporations are increasingly encouraged to adopt a data-driven mindset, pivoting to insights that shape strategies, enhance engagement, and keep attrition at bay. The utilization of comprehensive, real-time data feeds ensures decisions are not just reactionary but foresighted, crafting a tailored response to customer needs and preferences.
Telcos specifically are capitalizing on the potential to not only minimize churn but optimize operational efficiencies and discover new revenue streams by utilizing these disparate data types. An unabated pursuit towards data enrichment pivots telcos from surviving in a competitive market to thriving, driven by deeply personalized and analytically sound customer interactions.
The potential for further growth in this domain is immense. Emerging data forms, perhaps blending more intricate consumer preferences with macroeconomic indicators, can offer even deeper insights into what drives churn and what can effectively minimize it.
The data marketplace is experiencing a vibrant evolution, with more organizations discovering that they inadvertently sit on potentially valuable datasets that, when correlated with public data, present a lucrative opportunity for data monetization.
In conclusion, the integration of diverse datasets supersedes traditional limitations, paving the way for smarter, more accurate churn prediction models. Data-driven strategies are not optional; they are the cornerstone to unlocking future successes and sustaining market relevance.
Appendix
Telecommunications providers, analysts, data scientists, and strategists stand at the forefront of benefiting from enriched data insights designed to predict and manage churn. Through the augmentation of smartphone identifiers with broader data sets, these sectors are empowered to tailor and refine customer interactions.
In the investment realm, enriched datasets aid market analysts to foretell the performance of telecom stocks, identifying operators that strategically deploy data insights to gain competitive edge. This foresight provides actionable intelligence for investors in buy, hold, or sell decisions.
Consultants specializing in business strategy and market growth can use these insights to craft better strategies for clients, enabling improved retention outcomes and more engaging customer experiences. By leveraging AI and data analytics, they unlock greater potential in client-customer dynamics.
Insurance companies can utilize enriched churn forecasts to assess risk exposure related to telecom industry coverage, establishing more precise premium calculations and coverage terms that account for stabilized customer bases.
Market researchers across various sectors find enriched data instrumental in dissecting trends, curating consumer reports, and furnishing insights into telecom dynamics that outline broader market shifts driven by subscriber behavior.
Looking ahead, the role of Artificial Intelligence (AI) and advanced data analytics opens new possibilities. AI can decipher hidden patterns, transforming previously locked documents or government filings into valuable datasets for customer analytics. This evolution promises a revolutionary leap in understanding churn dynamics, ensuring a future where strategies are not merely formulated but meticulously tailored.