Factory Traffic Patterns: Leveraging Geolocation and Insights Data
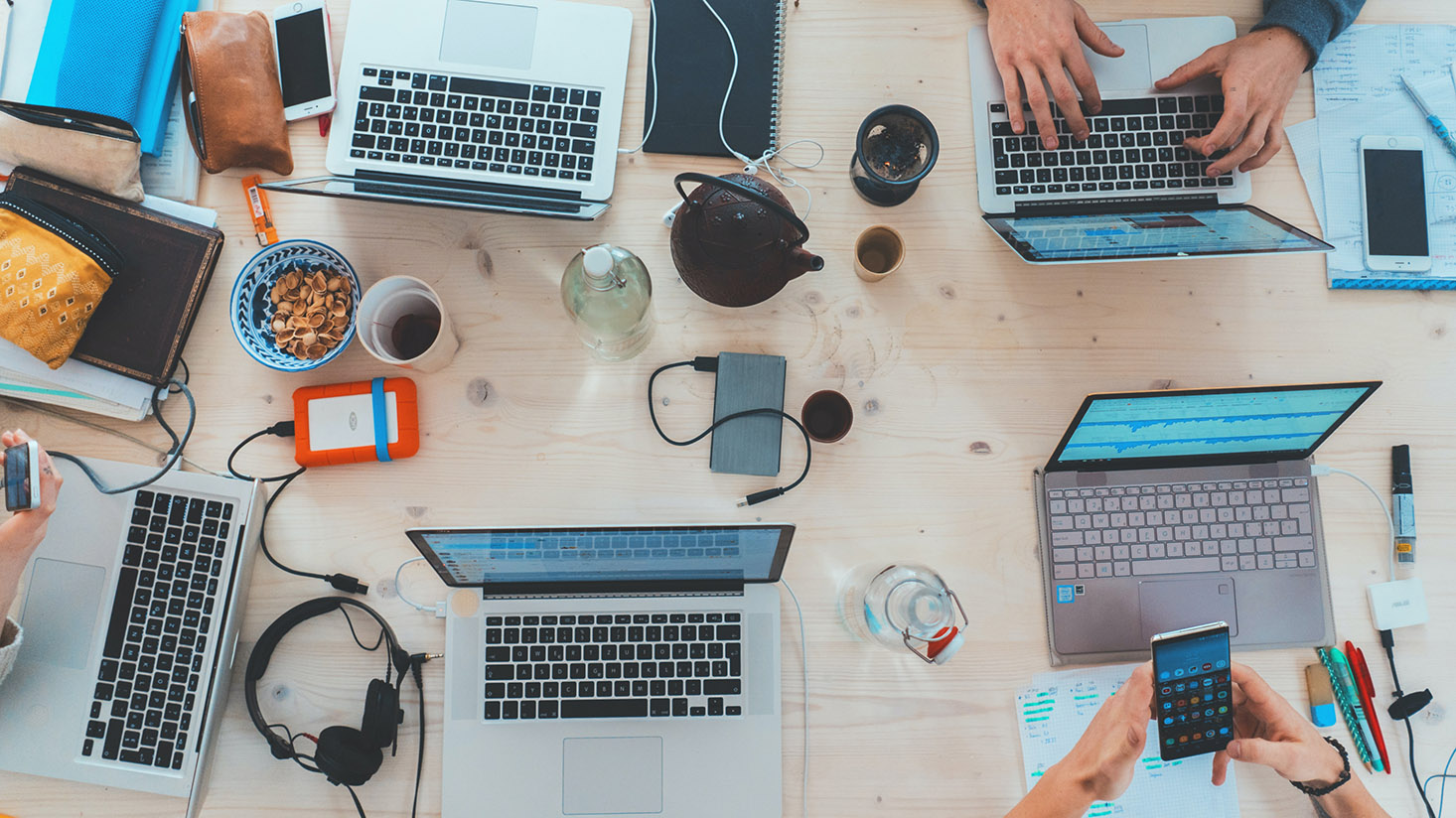
Introduction
The management of factory traffic has always been a complex and often opaque subject. Historically, gaining insights into factory traffic patterns was fraught with challenges. Before the age of connected devices and advanced data systems, businesses had little choice but to rely on manual counting or sporadic reporting from local observers. These methods were not only labor-intensive but also prone to errors and inconsistencies.
In the era before data, factory managers would consult shipping logs and utilize telephone updates to gauge activity. The reliance on such antiquated techniques meant that real-time adjustments to logistics fell short of efficiency, often resulting in lost time and decreased productivity. It was a guessing game where decisions were based on intuition rather than hard evidence.
Fortunately, with the advancement of technology, tools have emerged to revolutionize how factories track and analyze traffic. The rise of sensors, internet connectivity, and ubiquitous databases has reshaped the landscape of data gathering, effectively illuminating areas that were once dark spots in operational visibility. Technologies such as geolocation data and foot traffic analytics are now pivotal in monitoring and understanding factory dynamics.
Data plays a crucial role in comprehending factory traffic. It provides instantaneous, precise insights into shipment volumes and employee presence—a far cry from the weeks, even months, businesses used to spend assimilating such information. Today, decisions can be made in real time, offering flexibility and optimization previously thought impossible.
With the advent of external data sources, understanding factory traffic patterns has graduated from guesswork to an exact science. This chaotic or unstructured data is often harnessed by forward-thinking companies to streamline operations and make data-driven decisions that drive productivity and profitability.
We stand at the frontier of unprecedented data availability. From tracking the number of trucks entering and exiting a facility to discerning the ebb and flow of employee vehicles in parking lots, data offers a comprehensive perspective on both shipment volumes and operational shifts. The transformation it provides not only enhances a company's immediate logistics but also supports long-term strategic planning.
Geolocation Data
Geolocation data has emerged as a transformative tool in understanding traffic patterns, both at factories and beyond. The history of geolocation data is intertwined with the advent of GPS technology, which has gradually permeated various sectors, from automotive to mobile devices. GPS technology provides precise location-based insights that are indispensable in today's fast-paced industrial landscape.
Some practical examples of geolocation data include tracking vehicular movement to measure employee presence at factories or determining the number of trucks that enter and exit industrial premises. Historically, industries such as logistics, supply chain, and urban planning have leveraged this data to optimize routes and schedules.
Technological advancements in GPS data collection and analysis have fueled this development, offering an unprecedented scale of data granularity and accuracy. The acceleration of available data points has been rapid, with data now gathered at milliseconds intervals, enabling a highly detailed vision of factory operations.
Specifically, geolocation data can be used to learn about factory traffic in various ways:
- Traffic Pattern Analysis: Understanding the frequency and timing of truck arrivals and departures.
- Employee Tracking: Monitoring the number of employee vehicles present to gauge workforce abundance.
- Route Optimization: Identifying efficient routes for supply chain logistics to reduce costs and time.
- Shift Scheduling: Aligning shifts and workforce deployment with peak traffic periods for operational efficiency.
- Competitive Benchmarking: Analyzing traffic data of competing factories to gain strategic insights into their operations.
In essence, geolocation data not only reveals the quantifiable side of factory operations but also aids in strategizing for enhanced productivity and foresighted planning.
Conclusion
To sum up, the importance of data in understanding factory traffic cannot be overstated. Access to a diverse range of data sources enables businesses to be more agile and informed. With timely and accurate data, decisions are no longer based on assumptions or outdated reports, allowing companies to react swiftly to market or operational changes.
As organizations strive to be increasingly data-driven, they are discovering that the pursuit of better decision-making is directly tied to their ability to access high-quality and relevant data. This shift is evident in the growing trend of companies seeking to monetize their data for new revenue streams while improving their internal processes.
Moreover, the future holds intriguing possibilities for data, as new types emerge from the development of advanced sensors, machine learning algorithms, and integrated information systems. Predictive analytics, AI-powered forecasting, and even more granular real-time data may soon become the norm in tracking and understanding factory traffic.
Looking ahead, we can anticipate data innovations that further refine our understanding of factory operations. The ability to harness data not just from traditional sources but also from emerging platforms will empower businesses to tap into real-time insights and drive strategic planning.
The potential for this type of data-driven evolution presents an exciting opportunity for businesses and industries that have historically depended on inefficient methodologies. Leading the charge, savvy companies are well-positioned to capitalize on these insights, shaping the future of industrial operations with data as the cornerstone of their strategy.
Indeed, the confluence of data availability and technological advancement has set the stage for a new era in factory traffic analysis, where data is not just an asset but a driver of transformation and growth.
Appendix
There are numerous roles and industries that stand to benefit significantly from the analysis of factory traffic data. Among these, logistics managers, planners, and analysts are key figures who rely heavily on data to streamline operations and manage supply chains more efficiently.
Industries such as retail, distribution, manufacturing, and transport sectors are also heavily affected by traffic patterns at their facilities. For example, to avoid bottlenecks and wasted resources, managers use data to optimize truck scheduling and reduce turnaround times. Data has helped them reallocate labor and redesign workflows, ushering in improved performance and cost savings.
Investors, market researchers, and consultants also find value in traffic data. By understanding traffic volumes and patterns, they can glean insights into a factory's operational efficiency and performance, which in turn influences investment decisions and strategic recommendations.
The future holds the promise of AI potentially unlocking marginalized data to create even deeper insights. From predictive analytics to autonomous logistics, the fusion of external data and AI is set to break new ground in the analytics domain.
Thus, data not only helps to solve today's problems but also paves the way for future innovation and improvement within various industries. As data becomes increasingly pivotal, organizations that position themselves as data-driven entities will be at the forefront of their respective fields.
Hence, businesses across all sectors are encouraged to harness the power of data to unlock new potentials and drive their industries toward a more informed and efficient future.