Farm Machinery Lifespan Data
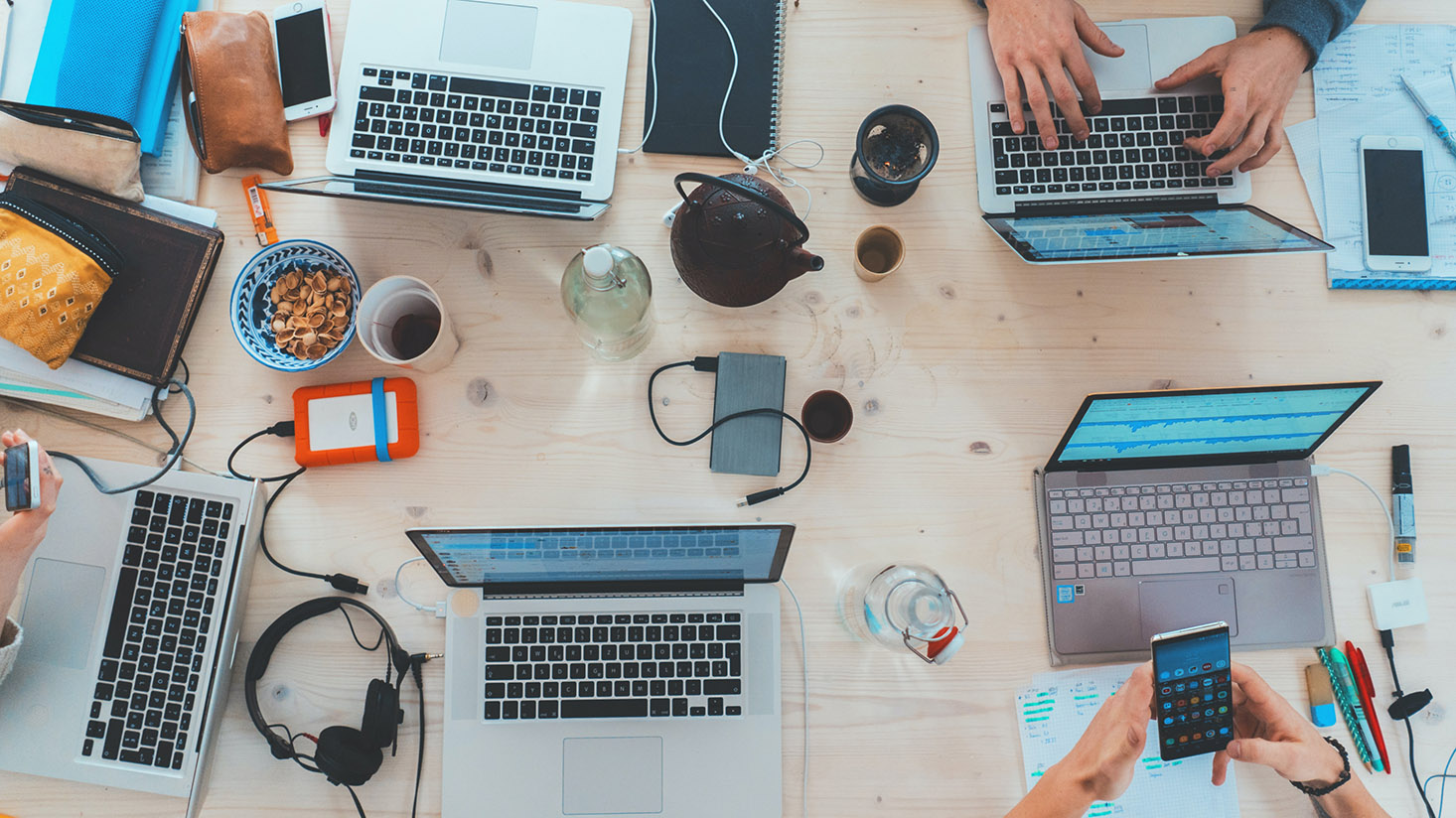
Introduction
Understanding the lifespan and maintenance needs of farm machinery in the US has historically been a complex and often imprecise endeavor. Before the digital age, insights into farm machinery maintenance and fleet life expectancy were largely anecdotal, derived from manual records or the personal experience of farmers and fleet managers. These methods, while valuable, lacked the precision and scalability of modern data analysis. Early attempts to track machinery health and predict maintenance needs relied on basic mechanical indicators and infrequent, manual inspections.
The advent of sensors, the internet, and connected devices has revolutionized the way we gather and analyze data on farm machinery. Previously, stakeholders had to wait weeks or months to understand changes in machinery health or performance. Now, real-time data collection and analysis offer immediate insights, enabling proactive maintenance and more accurate life expectancy predictions. This shift not only enhances operational efficiency but also contributes to significant cost savings and increased machinery uptime.
The importance of data in understanding farm machinery maintenance and fleet life expectancy cannot be overstated. In the past, the lack of precise data meant that decisions were often made based on best guesses rather than hard evidence. The proliferation of software and the move towards storing every event in databases have paved the way for a more data-driven approach. This evolution has provided a wealth of information that can be used to optimize machinery use, predict maintenance needs, and ultimately extend the life of valuable farm equipment.
Historically, the types of data used to track and predict farm machinery maintenance were limited. Maintenance logs, if kept, were often paper-based and subject to loss or damage. Financial records provided some insight into the costs associated with machinery upkeep but offered little in the way of predictive analytics. The introduction of connected machinery and the Internet of Things (IoT) has allowed for the collection of a much broader range of data, including operational metrics, usage patterns, and environmental conditions, all of which contribute to a more comprehensive understanding of machinery health and longevity.
The transition from antiquated methods to modern data analytics has not only improved the accuracy of maintenance and life expectancy predictions but has also enabled a shift from reactive to proactive maintenance strategies. This proactive approach minimizes downtime, reduces repair costs, and extends the operational life of machinery, providing significant benefits to farmers and fleet managers alike.
In summary, the evolution of data collection and analysis techniques has transformed the way we understand and manage farm machinery maintenance and life expectancy. The ability to collect and analyze real-time data has provided unprecedented insights, enabling more informed decision-making and optimizing the performance and longevity of farm equipment.
Industrials Data
Historical Context and Technological Advances
The collection and analysis of industrials data, particularly in the context of farm machinery, have undergone significant evolution over the years. Initially, data collection was manual and sporadic, with limited tools available for analysis. The introduction of sensors and connected devices has dramatically increased the volume and variety of data available. This technological advancement has enabled the collection of detailed information on machinery usage, maintenance needs, and operational efficiency.
Examples of industrials data relevant to farm machinery include economic life span, overhaul parts and labor costs, sales data, and information on financing and leasing. This data provides a comprehensive view of the machinery's performance, maintenance needs, and overall value over time. Industries such as agriculture, manufacturing, and finance have historically relied on this data to make informed decisions regarding machinery management and investment.
The proliferation of data in this category is accelerating, thanks to advances in data collection and analysis technologies. These advances have made it possible to gather detailed information on machinery performance and maintenance needs in real-time, providing valuable insights that were previously unattainable.
Utilizing Industrials Data for Insights
Industrials data can be used to gain a deeper understanding of farm machinery maintenance and fleet life expectancy in several ways:
- Economic Life Span: Data on the economic life span of farm machinery helps stakeholders understand how long equipment can be expected to operate before requiring significant overhaul or replacement.
- Overhaul Parts and Labor: Information on parts and labor costs associated with machinery overhaul provides insights into the maintenance expenses over the equipment's lifespan.
- Sales and Financing Data: Tracking farm machinery sales and financing trends offers insights into replacement cycles and the overall market health.
- Depreciation and Future Value: Data on equipment depreciation and future value predictions aid in financial planning and investment decisions.
By leveraging industrials data, stakeholders can make more informed decisions regarding machinery maintenance, replacement, and investment. This data-driven approach leads to optimized machinery use, reduced downtime, and extended equipment life, ultimately contributing to greater operational efficiency and cost savings.
Conclusion
The importance of data in understanding and managing farm machinery maintenance and fleet life expectancy cannot be overstated. The transition from manual, anecdotal methods to data-driven analytics has revolutionized the way stakeholders approach machinery management. Real-time data collection and analysis provide immediate insights, enabling proactive maintenance strategies and more accurate life expectancy predictions.
As organizations become increasingly data-driven, the discovery and utilization of relevant data types will be critical to optimizing farm machinery performance and longevity. The ability to analyze vast amounts of real-time data offers unprecedented opportunities for improving operational efficiency and reducing costs.
Looking to the future, the potential for monetizing valuable data generated by farm machinery is significant. As companies continue to collect and analyze data, new insights into machinery maintenance, performance, and life expectancy will emerge, providing further opportunities for optimization and innovation.
The advent of artificial intelligence (AI) and machine learning technologies holds the promise of unlocking even greater value from data. These technologies have the potential to analyze historical and real-time data in ways that were previously impossible, offering new insights and predictive capabilities that will further enhance machinery management and maintenance strategies.
Appendix
Industries and roles that could benefit from access to farm machinery lifespan and maintenance data include investors, consultants, insurance companies, market researchers, and agricultural professionals. These stakeholders face challenges related to machinery management, investment decisions, and operational efficiency. Data has transformed these industries by providing actionable insights that inform strategic decision-making.
The future of data in farm machinery management is bright, with AI and machine learning poised to unlock even greater value from existing and future datasets. As these technologies continue to evolve, the potential for innovative solutions to longstanding challenges in machinery maintenance and management is immense.