Gain Actionable Insights with Detailed Grocery SKU Data
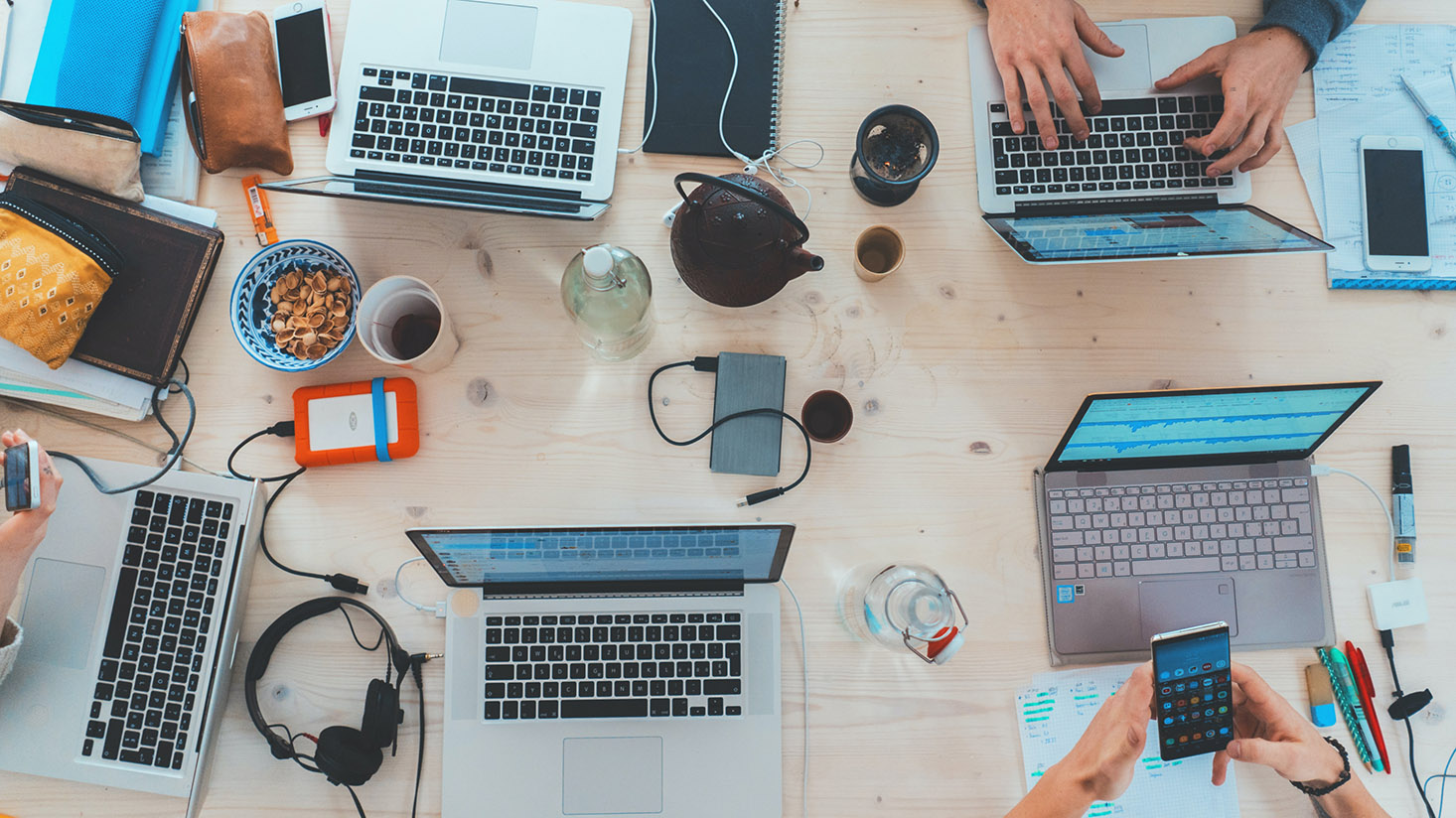
Introduction
The grocery retail landscape is a complex ecosystem, influenced by numerous factors ranging from consumer preferences to supply chain dynamics. Historically, gaining insights into the nuances of this industry, particularly at the SKU (Stock Keeping Unit) level, was a daunting task. Traditional methods of gathering and analyzing data were fraught with challenges. Retailers and manufacturers often relied on broad, aggregate data that didn't capture the intricacies required to make informed decisions. Surveys, limited-point audits, and manually maintained records were among the few tools available, providing limited visibility into consumer behavior and market trends.
Prior to the digital age, the grocery sector had to make do without the treasure trove of data we take for granted today. Decision-makers depended heavily on anecdotal evidence, intuition, and simple historical sales data aggregated at the category level. Such reliance often led to inaccuracies and inefficiencies in inventory management, marketing strategies, and customer engagement – crucial areas where specific, real-time insights could have made all the difference.
However, the proliferation of technology, especially the internet, sensors, and connected devices, has radically transformed the landscape. Modern data collection methods enable businesses to capture vast amounts of granular data, essential for deciphering the intricate patterns and preferences of consumers. Point-of-Sale (POS) systems, online grocery platforms, and loyalty programs are just a few examples of innovations that have enriched the data available to the industry.
The significance of data in the modern grocery ecosystem cannot be overstated. It has illuminated areas that were formerly in shadow, offering a near-immediate understanding of shifts in consumer trends and inventory dynamics. This capability allows businesses not only to react in real-time but to anticipate market movements and strategize accordingly.
With SKU level data, retailers and manufacturers can now track individual products with extraordinary precision. This detailed information supports a more refined approach to supply chain management, promotional strategies, and customer relationship management. Furthermore, the advent of various data categories has bridged the gap between raw data and strategic insights, empowering businesses to operate with a newfound agility.
The capacity to quickly adapt to changes in consumer preferences or supply disruptions, harnessed from real-time data, means businesses can maintain competitiveness and foster customer loyalty even in the face of evolving market conditions. As we'll explore below, different types of data are pivotal in painting a comprehensive picture of SKU level activity in the grocery sector.
Transaction Data
Transaction Data has emerged as a cornerstone in understanding grocery SKU dynamics. This data type traces its origins to the introduction of electronic point-of-sale systems, which enabled the collection of detailed records of every transaction made at a checkout terminal. Initially adopted by large grocery chains to streamline operations and reduce human error, these systems have evolved into sophisticated data gathering tools.
Today, transaction data offers a micro-level view of consumer activity, tracking purchases down to the SKU level. This granular data provides insights into how specific products perform, capturing nuances across different store locations, time periods, and consumer segments. From regional grocers to nationwide chains, transaction data is invaluable for tailoring inventory and marketing strategies to meet customer demand accurately.
This data's growth has been fueled by technological advancements, including the integration of POS data with advanced analytics platforms. Such combinations allow businesses to observe trends over time, identify emerging patterns, and make data-informed decisions. Furthermore, transaction data is being supplemented with online sales records from partners such as InstaCart and Shipt, broadening its scope to encompass both in-store and online shopper behavior.
Key applications of transaction data in the grocery sector include:
- Inventory Management: Ensures optimal stock levels to meet demand without overstocking.
- Pricing Strategies: Guides dynamic pricing models based on demand fluctuations.
- Promotional Campaigns: Informs targeted advertising based on purchasing patterns.
- Vendor Negotiations: Supports negotiation terms with suppliers using detailed sales data.
- Trend Analysis: Enables tracking of emerging product trends and changing consumer preferences.
With transaction data becoming increasingly detailed and prevalent, the grocery sector is moving towards a state where predictive analytics and machine learning models can be deployed to enhance business outcomes. By continuously feeding these models with fresh data, retailers can simulate scenarios, forecast sales, and proactively adjust strategies to optimize performance.
Consumer Behavior Data
Consumer Behavior Data has revolutionized the grocery sector by providing insights into the motivations and actions of shoppers. Initially, understanding consumer behavior was confined to qualitative studies and surveys, offering a snapshot view rather than a comprehensive picture. The advent of digital datasets now provides a continuous, dynamic view of consumer actions and preferences.
This data is typically derived from loyalty programs, shopping apps, and transactional data at both individual and household levels. By mapping detailed transactions against a range of demographics and behavioral patterns, businesses can extrapolate valuable insights about their customers, tailoring their products and services to better meet consumer needs.
Consumer behavior data is crucial in tracking the shift from in-store to online shopping, a trend accelerated by digital transformation in the retail sector. By understanding how and why consumer preferences change across different channels, grocery chains can effectively strategize their omni-channel retailing efforts.
Specific applications of consumer behavior data in the grocery industry include:
- Customer Segmentation: Divides consumers into distinct groups based on purchasing habits.
- Loyalty Programs: Enhances program design to boost customer retention and lifetime value.
- Product Development: Informs innovation based on observed consumer needs and preferences.
- Marketing Personalization: Tailors messages to specific consumer segments to increase engagement.
- Channel Optimization: Guides retailers on optimal channel strategies based on consumer behavior trends.
The acceleration of consumer behavior data is driven by the continuous enhancement of data collection techniques and the growing ability of businesses to process and organize vast datasets. As businesses embrace these developments, they can better foresee market shifts, allowing for strategic foresight and planning.
The future of consumer behavior data holds promise with the integration of AI and machine learning, which can facilitate deeper dives into data and unlock new insights into consumer tendencies. This emerging synergy is positioning retailers to engage with consumers more effectively, crafting experiences that resonate on a personal level.
Conclusion
To summarize, external data is invaluable in enhancing the understanding of grocery SKU dynamics, shedding light on the unseen complexities that influence consumer behavior and product performance. As data-driven strategies penetrate deeper into the retail sector, the potential for optimizing operations, marketing, and customer relations grows exponentially.
The variety of categories of data available today creates a rich tapestry of information that enables businesses to pivot with precision, anticipate market shifts, and forge stronger connections with their customers. The power of data lies in its ability to turn the abstract into the tangible, allowing organizations to not just react, but to lead.
In this data-centric age, organizations are increasingly recognizing the value of becoming more data-driven. As they seek to monetize their data, businesses can extract new value from their existing information repositories, turning potential insights into actionable strategies. The grocery sector, like many others, stands on the brink of unlocking unprecedented potential through the innovative use of data.
Looking forward, the field could see the emergence of new types of data sourced from IoT devices within stores, enhanced datasets from AI-driven consumer interaction tools, or even detailed environmental sensors that affect store dynamics. These potential data streams could augment existing insights and propel the grocery industry towards even greater efficiency and understanding.
The role of data discovery, facilitated by platforms such as Nomad Data, will be pivotal in helping businesses navigate their data needs and find the right data solutions to achieve their strategic objectives. The pursuit of knowledge and the drive to innovate will continue to be fueled by the power of data, and those with the tools to harness it will be at the forefront of change.
Appendix: Industry Roles and Benefits
The potential beneficiaries of detailed grocery SKU data span across various industries and roles. The omnipresence of data analytics has permeated numerous sectors, providing insights and solutions to longstanding challenges. Here's a look at some of the key players:
Investors and Analysts: Armed with precise SKU data, investors can make informed decisions about investments in retail stocks, based on product performance trends and consumer demand forecasts. This clarity on consumer purchasing dynamics provides a robust basis for evaluating potential returns.
Retail Consultants: SKU data offers consultants a granular view of retail operations, allowing them to recommend strategies that improve efficiency and effectiveness, whether in marketing, inventory management, or customer engagement.
Consumer Packaged Goods (CPG) Companies: With enhanced insights, CPG firms can innovate their product lines to better meet consumer needs, optimize supply chains, and tailor marketing efforts to precisely target desired customer segments.
Insurance Companies: Understanding SKU-level sales patterns aids in risk assessment and premium calculations, particularly where goods perishability or Target consumer bases are concerned. Predictive analytics can anticipate market conditions affecting coverage needs.
Market Researchers: In-depth SKU data facilitates impactful studies on market trends, consumer preferences, and brand loyalty, aiding companies in aligning product portfolios with evolving consumer tastes.
As data technologies evolve, AI could further unlock the hidden value within vast arrays of historical data and modern filings, translating raw information into intuitive, actionable insights. Future-driven businesses recognize that embracing these technological advancements will not only shape their success but also redefine the competitive landscape.