Global Trend Analysis Data
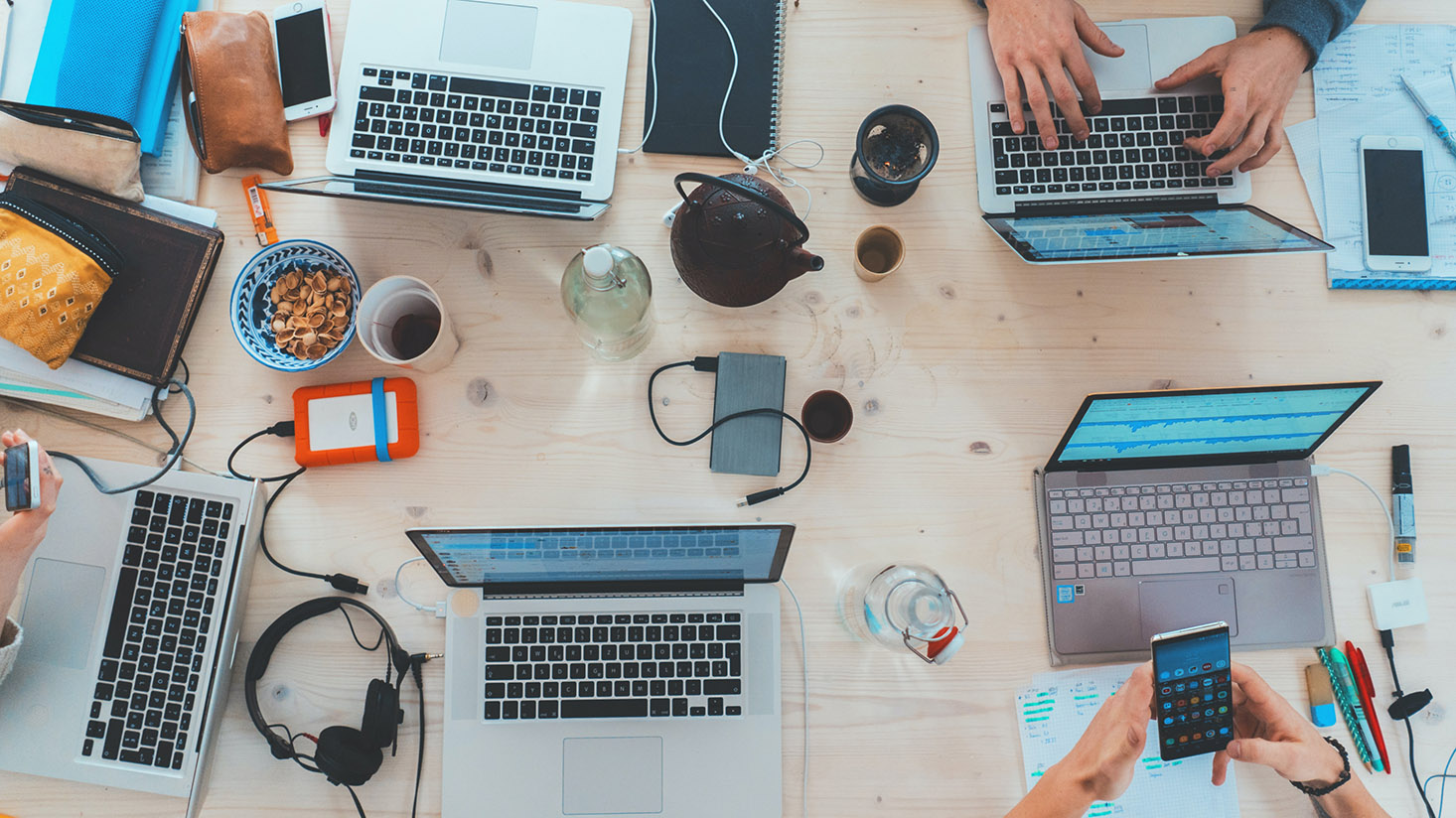
Introduction
In the realm of business intelligence and market research, understanding global trends has always been a cornerstone for strategic decision-making. Historically, gaining insights into these trends was a cumbersome process, fraught with delays and inaccuracies. Before the digital revolution, firms relied on manual surveys, paper-based records, and anecdotal evidence to gauge market sentiments and shifts. These antiquated methods often meant that businesses were making decisions based on outdated or incomplete information.
The advent of the internet, connected devices, and sophisticated software has dramatically transformed this landscape. The proliferation of sensors and the ubiquity of digital communication have made it possible to collect and analyze data on an unprecedented scale. This shift towards a data-driven approach has enabled businesses to track changes in real-time, offering a level of insight that was previously unimaginable.
One of the most significant areas of interest in this data-driven era is the analysis of text-based correspondence data, including social media posts, emails, and other forms of digital communication. This type of data, enriched with metadata such as location and time stamps, offers a treasure trove of information for detecting trends over time and across regions. The challenge, however, has been in harnessing this vast amount of data to extract meaningful insights.
Historically, the lack of sophisticated tools and technologies meant that analyzing this type of data was not only difficult but often impossible. Businesses had to rely on broad, general trends, missing out on the nuanced insights that could be gleaned from a more detailed analysis. The introduction of advanced data analytics tools and AI technologies has changed the game, allowing for the extraction of insights from large datasets quickly and accurately.
The importance of this type of data in understanding global trends cannot be overstated. In a world where trends can emerge and evolve rapidly, having access to real-time data allows businesses to stay ahead of the curve. Whether it's tracking the spread of a global pandemic or understanding consumer sentiment in different regions, text-based correspondence data offers a unique lens through which to view the world.
As we move forward, the role of data in understanding and predicting global trends will only increase. The ability to quickly and accurately analyze text-based correspondence data will be a key differentiator for businesses looking to navigate the complexities of the global market.
Media Measurement Data
The advent of media measurement data has been a game-changer in the analysis of global trends. This category of data encompasses a wide range of sources, from social media platforms to news outlets, offering a comprehensive view of public discourse and sentiment. The ability to measure and analyze this data has provided businesses with unprecedented insights into consumer behavior, market trends, and global events.
Historically, media measurement was limited to simple metrics such as circulation numbers for print media or viewership ratings for television. However, the digital revolution has expanded the scope of media measurement to include detailed analytics on user engagement, sentiment analysis, and trend detection. This shift has been driven by advances in AI and machine learning technologies, which have made it possible to process and analyze large datasets in real time.
Examples of media measurement data include:
- Social media analytics: Tracking mentions, hashtags, and sentiment on platforms like Twitter, Facebook, and Instagram.
- Online news monitoring: Analyzing the frequency and sentiment of news articles related to specific topics or events.
- Consumer reviews and feedback: Aggregating and analyzing customer reviews from e-commerce sites and other online platforms.
Industries ranging from marketing and public relations to finance and healthcare have leveraged media measurement data to gain insights into consumer preferences, market dynamics, and global events. The technology advances in data analytics and AI have been instrumental in the growth of this data category, enabling businesses to sift through vast amounts of information to find relevant insights.
The acceleration of media measurement data has been remarkable, with the volume and variety of data growing exponentially. This growth has been fueled by the increasing digitalization of communication and the proliferation of online platforms. As a result, businesses now have access to a wealth of data that can be used to track global trends, monitor brand sentiment, and understand consumer behavior in real-time.
Specifically, media measurement data can be used to:
- Identify emerging trends: By analyzing social media conversations and online news articles, businesses can detect new trends as they emerge.
- Monitor brand sentiment: Tracking mentions and sentiment around a brand can help businesses understand public perception and identify areas for improvement.
- Understand consumer behavior: Analyzing consumer reviews and feedback can provide insights into customer preferences and buying behavior.
Examples of how media measurement data has been used to gain insights include tracking the spread of the COVID-19 pandemic, monitoring public sentiment during elections, and understanding consumer reactions to new product launches. The ability to quickly and accurately analyze this data has provided businesses with a competitive edge in a rapidly changing world.
Audio Data
Another critical category of data that has emerged in recent years is audio data. This type of data includes recordings from social media, podcasts, news broadcasts, and other audio sources. The analysis of audio data offers unique insights that are not available through text-based analysis alone, such as tone of voice, sentiment, and emotional context.
Historically, the analysis of audio data was limited by the technology available for processing and transcribing recordings. Advances in speech recognition and natural language processing technologies have made it possible to automatically transcribe and analyze audio data at scale. This has opened up new avenues for understanding consumer sentiment, market trends, and global events through the analysis of spoken conversations.
Examples of audio data include:
- Social media voice posts: Analyzing voice messages and posts on platforms like WhatsApp and Facebook.
- Podcast episodes: Transcribing and analyzing content from popular podcasts related to specific topics or industries.
- News broadcasts: Monitoring and analyzing news reports and interviews for sentiment and key themes.
Industries such as media and entertainment, marketing, and public relations have increasingly turned to audio data to gain deeper insights into consumer behavior and public sentiment. The ability to analyze the tone and emotional context of spoken conversations offers a level of nuance that is difficult to achieve through text-based analysis alone.
The growth of audio data has been driven by the increasing popularity of podcasts, voice-based social media posts, and the proliferation of smart speakers and voice assistants. As a result, businesses now have access to a rich source of data that can be used to enhance their understanding of global trends and consumer preferences.
Specifically, audio data can be used to:
- Understand consumer sentiment: Analyzing the tone and emotion in voice recordings can provide insights into how consumers feel about a brand or product.
- Identify key themes and topics: Transcribing and analyzing audio content can reveal the main themes and topics of conversation related to specific events or trends.
- Monitor brand mentions: Tracking mentions of a brand in podcasts and news broadcasts can help businesses understand how they are being discussed in the media.
Examples of how audio data has been used to gain insights include monitoring public sentiment during political campaigns, understanding consumer reactions to product announcements, and tracking the spread of misinformation during global events. The ability to analyze audio data at scale has provided businesses with a powerful tool for understanding the world around them.
Conclusion
The importance of data in understanding and predicting global trends cannot be overstated. As we have seen, categories of data such as media measurement and audio data offer unique insights that can help businesses navigate the complexities of the global market. The ability to analyze text-based correspondence data, enriched with metadata such as location and time stamps, has opened up new possibilities for detecting trends and understanding consumer behavior.
As organizations become more data-driven, the role of data discovery will be critical in unlocking the value hidden in vast datasets. The proliferation of digital communication and the advances in data analytics and AI technologies have made it possible to collect and analyze data on an unprecedented scale. This shift towards a data-driven approach has enabled businesses to track changes in real time, offering a level of insight that was previously unimaginable.
Looking to the future, corporations are increasingly looking to monetize the valuable data they have been creating for decades. The analysis of text-based correspondence data, including social media posts, emails, and other forms of digital communication, will continue to play a key role in understanding global trends. As new types of data emerge, businesses will have access to even more insights that can help them make better decisions.
The potential for data to transform industries and roles is immense. From investors and consultants to insurance companies and market researchers, the ability to leverage data for insights has become a critical capability. The future may see AI technologies unlocking the value hidden in decades-old documents or modern government filings, offering new perspectives on global trends and consumer behavior.
In conclusion, the role of data in understanding global trends is more important than ever. As businesses strive to stay ahead of the curve, the ability to quickly and accurately analyze data will be a key differentiator. The insights gained from media measurement and audio data, among other categories, will continue to provide businesses with the tools they need to navigate the rapidly changing global landscape.
Appendix
The transformation brought about by the availability and analysis of data has been profound across various industries and roles. Investors, consultants, insurance companies, and market researchers are just a few examples of those who have benefited from this data-driven revolution. The ability to access and analyze data has transformed the way these industries operate, offering new insights and opportunities for growth.
For investors, access to real-time data on market trends and consumer sentiment has become a critical tool for making informed decisions. Consultants leverage data to provide strategic advice to their clients, helping them to navigate complex market dynamics. Insurance companies use data to assess risk and tailor their products to meet the needs of their customers. Market researchers rely on data to understand consumer behavior and identify emerging trends.
The future of data analysis holds great promise. With the continued advancement of AI and machine learning technologies, the potential to unlock the value hidden in data is immense. From analyzing decades-old documents to modern government filings, AI has the potential to reveal insights that were previously inaccessible. This could revolutionize the way businesses and industries operate, offering new perspectives on global trends and consumer behavior.
In conclusion, the role of data in transforming industries and roles cannot be understated. As we move forward, the ability to leverage data for insights will continue to be a critical capability for businesses and professionals alike. The future of data analysis is bright, with AI and machine learning technologies leading the way in unlocking the value hidden in vast datasets.