Harness Financial and NLP Data for Enhanced Text Analytics Insights
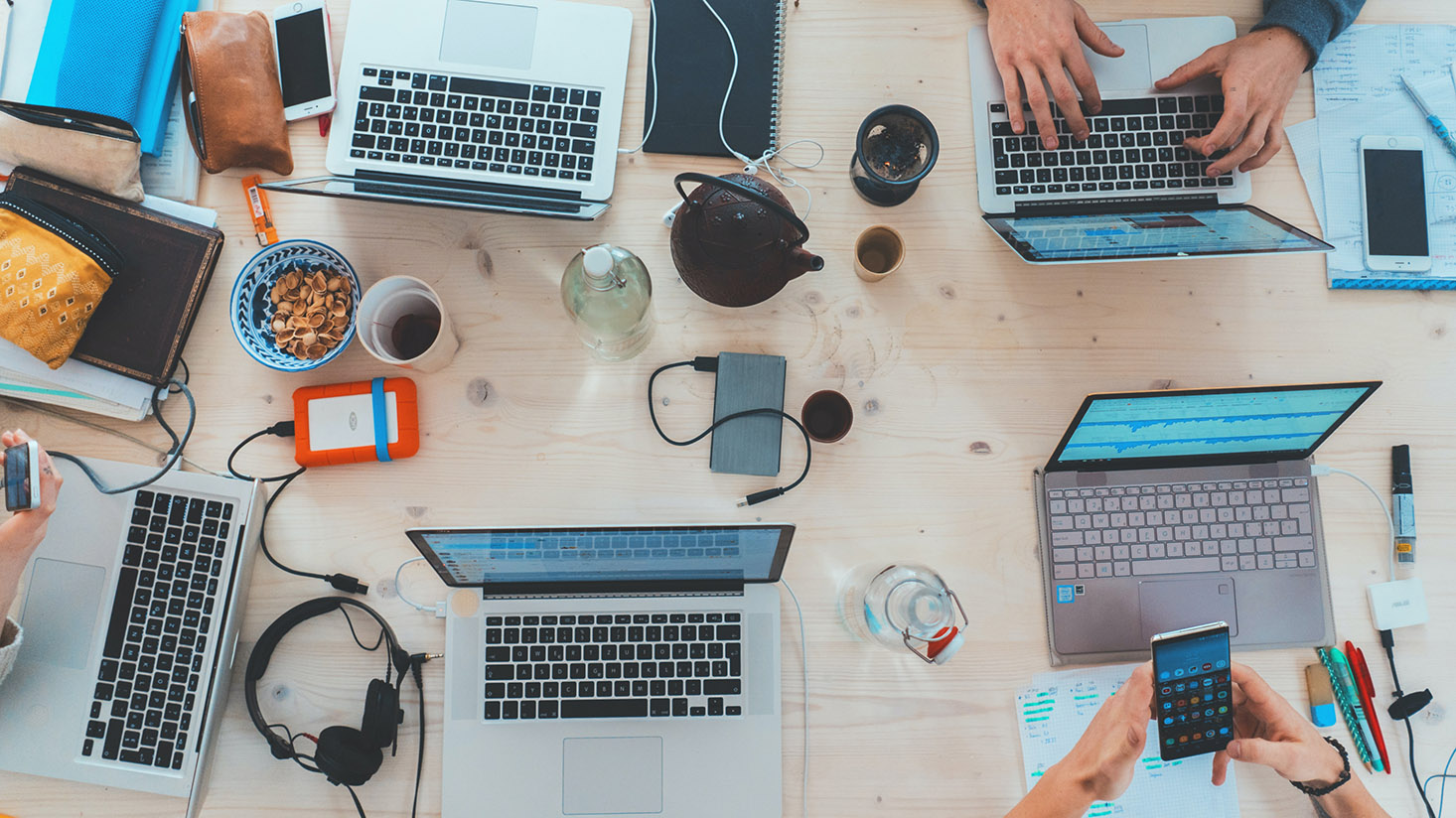
Introduction
Natural Language Processing (NLP) and Text Analytics have become critical tools in dissecting large volumes of unstructured data to glean actionable insights. Historically, deriving insights from text data was fraught with challenges. This struggle was primarily due to the lack of sophisticated technology and comprehensive data. In earlier times, analysts relied on manual methods such as reading through articles, reports, and financial documents, often leading to subjective interpretations and oversight. Other analog methods included listening to speeches or financial reports, a labor-intensive task that offered little precision or efficiency. Before the advent of formal data practices, businesses were primarily guided by intuition and traditional news cycles, which meant decision-making could be slow and reactive.
The revolution in data capture and analysis came with the introduction of sensors, the widespread adoption of the internet, and the proliferation of connected devices. These innovations allowed for real-time data collection and analytics, transforming how businesses operate and strategize. Furthermore, the evolution of software that records and compiles every digital interaction has amplified the availability and granularity of data.
This shift has profoundly impacted industries, providing them with the tools to understand data dynamics in real time. In finance, the ability to track investor sentiment, news flow, and social media trends has demystified previously elusive market trends. In the retail sector, understanding consumer preferences and behaviors is facilitated by analyzing textual feedback from multiple platforms.
Recognizing the importance of data, organizations now prioritize data storage and analysis, allowing for instant reactionary strategies instead of waiting weeks or months to gauge market changes. This transition underscores a broader trend towards data-driven decision-making.
The power of real-time analytics cannot be overstated. It allows businesses to pivot strategies quickly, seizing market opportunities or mitigating risks before they impact the bottom line. Such an approach requires a robust understanding and application of categories of data including sentiment, news, and financial metrics.
Understanding how these categories of data enrich insights is crucial for businesses seeking to leverage NLP and Text Analytics. This article delves into different data types and how they illuminate various facets of text-based analytics.
Financial Markets Data
History and Evolution
Financial markets data has always been at the forefront of investment and trade decision-making. Traditionally, financial insights were derived from stock prices, company reports, and primary news articles. Analysts developed manual models to predict trends based purely on financial statements and periodic disclosures. However, this static data rarely captured real-time investor sentiment or market news dynamics.
With advancements in technology, especially AI-driven NLP, the scope and depth of financial markets data have expanded. AI platforms now process investor sentiment trends by analyzing vast amounts of financial media, social media, and live broadcasts.
Historically, industries such as investment banking, portfolio management, and financial consulting have heavily relied on this data. The introduction of memory-based sentiment models that account for news decay has been a breakthroughs in understanding market fluctuations.
The technology has dramatically expanded with processes such as continuous API signal delivery and integration capabilities with other large datasets. This accelerates the pace at which data can be turned into insights.
The real-time integration of news sentiment now provides external data in identifying stocks that might be poorly impacted by negative news or have the potential for positive movement based on favorable sentiment.
Applications in NLP Text Analytics
Financial markets data offers diverse applications in NLP text analytics. Here are some key examples:
- Investor Sentiment Analysis: By analyzing social media and news, NLP can predict public sentiment on specific stocks or markets, aiding in stock selection and portfolio management.
- Market Trend Prediction: Historical sentiment scores provide clues to long-term market trends, allowing for strategic investment planning.
- Real-Time Trading Signals: Sentiment signals derived from financial markets data can inform algorithmic trading strategies, enabling traders to capitalize on immediate market shifts.
- Risk Management: Companies leverage NLP analytics to gauge potential adverse events affecting market stability, helping mitigate operational and financial risks.
- Corporate Events Impacts: By analyzing company-related news, firms can preemptively respond to events that might affect their market standing.
Business Data
History and Evolution
Business data is integral to understanding corporate operations and financial outcomes. Initially, this data was limited to public statements or periodic disclosures, making comprehensive analysis difficult. The advent of big data technologies and NLP has transformed how we gather corporate insights.
In this domain, data roles span various industries, including consulting firms, market analysis, and more. Financial events datasets sourced from news items, press releases, and social media have allowed businesses to track operations continuously.
Technological advancements have enabled clean datasets using machine-learning classifiers that automatically score events based on potential impact. This automation has expanded the dataset scale, covering thousands of companies and extensive event categories.
Thus, corporate businesses today rely on such datasets to make vital decisions based on up-to-date information rather than static historical data.
Applications in NLP Text Analytics
Business data plays a significant role in NLP analytics, offering insights into various corporate functions. Notable applications include:
- Corporate Strategy Formation: Analyzing the potency and impact of different corporate events offers insights that guide strategic planning.
- Mergers and Acquisitions (M&A) Analysis: By examining press releases and social media, businesses can assess M&A implications and understand industry movements.
- Market Positioning: Continuous monitoring of public company operations enables firms to gauge their competitive position and market dynamics.
- Performance Forecasting: Leveraging NLP, companies predict performance outcomes based on historical event impacts, thus refining their forecasts.
- Risk Assessment: Sentiment analysis of corporate communications helps in anticipating risks and implementing preemptive measures.
Conclusion
The journey towards understanding complex datasets such as NLP and Text Analytics has long been challenging but rewarding. With the integration of comprehensive financial and business data, the landscape of insights has dramatically improved. Companies are no longer relegated to speculative or slow-paced decision-making but can leverage AI and Big Data technologies to foster informed and agile strategies.
Harnessing various types of data, be it investor sentiment or corporate events, enables businesses to position themselves advantageously in a rapidly changing marketplace. This comprehensive understanding facilitates strategies that are predictive rather than solely reactive.
Data's importance in business realms cannot be overstated. Companies embracing a data-driven culture gain insights faster and can monetize hidden or historical data, bolstering profitability and competitive advantage.
The future of data holds even broader possibilities as industries become increasingly integrated. New data types and analytics will emerge, offering profound insights and driving innovations not yet imagined.
Organizations need to stay vigilant and adaptive, ensuring they remain leaders in the data-driven business sphere. Data monetization opens new revenue streams, attracting interest across various disciplines.
Thus, embracing future data landscapes requires both understanding and strategic application, vital to navigating today's and tomorrow's business environments.
Appendix
- Investors and Analysts: Investment firms and financial analysts use datasets to gauge market trends and make informed decisions. Data-driven insights translate into sound investment strategies and risk management.
- Consultants and Strategists: Data's impact is profound in consulting, where strategic advice hinges on accurate and timely analytics. NLP insights facilitate the synthesis of impactful strategies.
- Insurance Firms: Through textual analyses of corporate risks and sentiments, insurance companies assess risk profiles more accurately, tailoring their offerings and risk assessments.
- Market Researchers: Leveraging extensive datasets, researchers gain a multifaceted understanding of market dynamics and consumer mindsets, supporting comprehensive market analyses.
- Corporate Decision-makers: Executives and managers utilize data-driven insights for operational and strategic foresight, transforming decisions from speculative to informed.
The future holds exciting prospects with AI potentially unlocking decades of hidden insights within textual data. Automated text analytics will redefine how we dissect information, supporting predictions, modeling scenarios, and more.