Harness Loan Origination Data for Insightful Financial Decisions
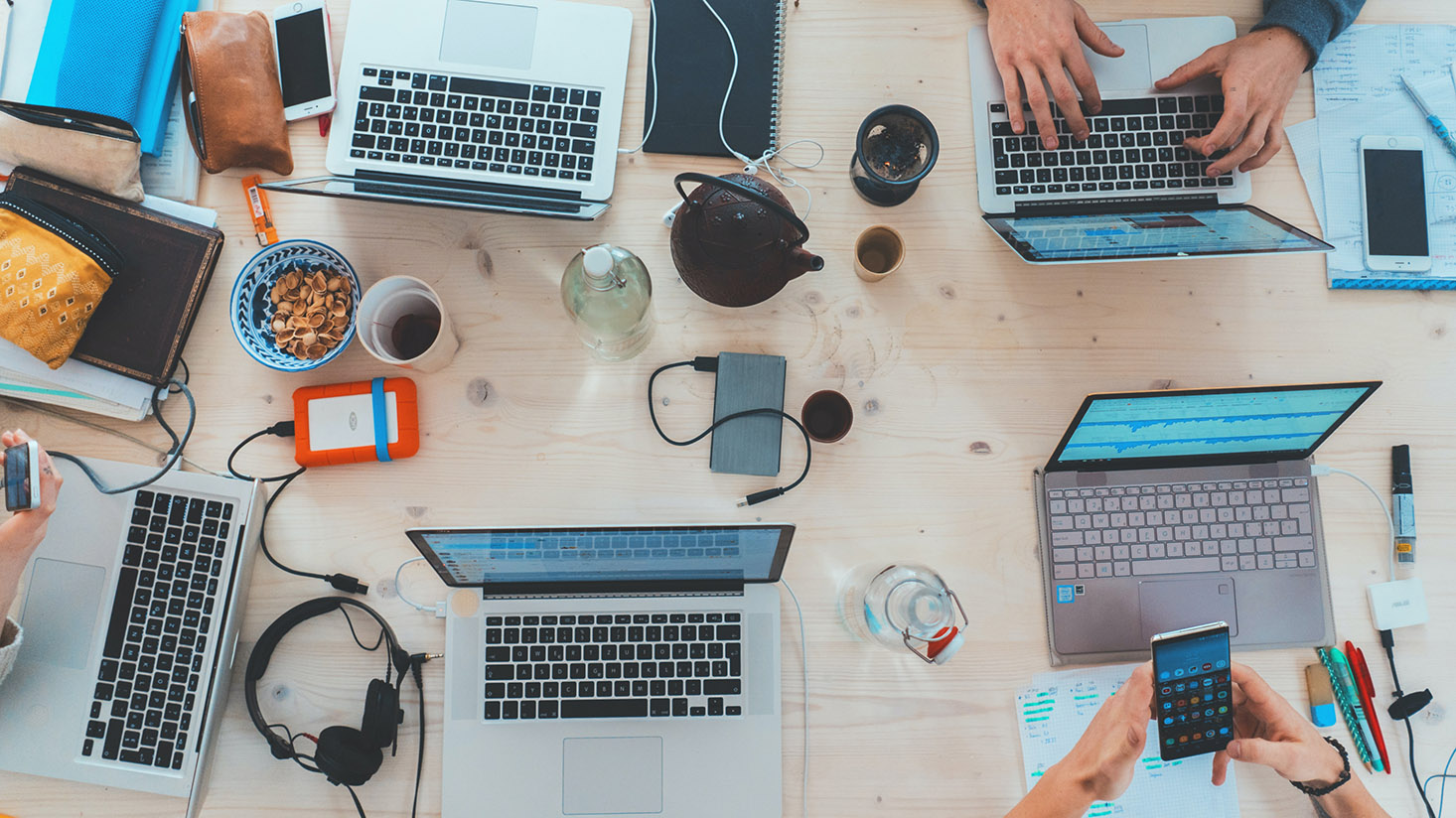
Introduction
The dynamics of loan approval and rejection have long been a subject of curiosity and critical analysis, especially as financial institutions strive to streamline lending processes while minimizing risk. Historically, insights into loan rejection rates were elusive, largely due to a lack of comprehensive data collection and analysis tools. Financial institutions depended on rudimentary methods such as manual record-keeping and paper-based applications, which were not only time-consuming but also prone to errors.
In the absence of robust datasets, understanding the nuances of loan rejections was more art than science. Bankers and loan officers relied on anecdotal evidence and intuition, making it challenging to pinpoint the true factors influencing a loan's fate. Without access to granular data, financial institutions often found themselves in the dark, waiting weeks or months for high-level trend reports that offered little actionable insight.
The advent of digital technologies marked a turning point. With the proliferation of external data, including sensor-generated and internet-based data, financial institutions began digitizing their processes. The transition toward electronic data storage and the integration of connected devices enabled real-time data collection and analysis, providing unprecedented visibility into every aspect of banking operations.
In today's data-driven environment, understanding loan rejection rates is essential for both lenders and policymakers. Armed with detailed datasets, stakeholders can observe patterns, make informed decisions, and implement strategies to improve loan approval rates. This level of insight was unheard of just a few decades ago when the financial world relied heavily on macroeconomic indicators with delayed reporting.
Data has not only revolutionized the understanding of loan rejection rates but also allowed financial professionals to respond swiftly to market changes, crafting timely and effective policies. Insights gleaned from data analysis enable financial institutions to tailor products better suited to customer needs and mitigate potential risks.
In this article, we will explore how various categories of data can illuminate the intricacies of loan rejection rates, focusing on the loan origination data and other relevant data types to unlock greater understanding and strategic advantage.
Consumer Behavior Data
History and Evolution
The rise of consumer behavior data has its roots in the evolution of digital technologies and consumer analytics. Historically, data on consumer behavior was gathered through surveys, telephone interviews, and in-person focus groups. Although insightful, these methods were limited in scope and scale. The digitization of commerce and finance has revolutionized how this data is collected and utilized.
Modern consumer behavior data provides expansive insights into the financial decisions people make, including their borrowing patterns, spending habits, and creditworthiness. This transformation has been facilitated by advances in digital storage, data processing capabilities, and cloud-based platforms which enable seamless collection and analysis.
Consumer behavior data, particularly in the context of loan origination, offers valuable insights into how credit scores, income level, and geographic location influence credit applications. As the volume of available data grows, financial institutions gain rich, nuanced insights into borrower profiles and risk factors.
Applications in Loan Origination Analysis
One significant application of consumer behavior data is in understanding loan origination trends. By analyzing data related to the origination of loans broken down by credit score, income, and geographic area, financial institutions can:
- Identify Trends: Spot trends in loan application and approval rates, adjusting strategies accordingly.
- Risk Assessment: Evaluate borrower risk more effectively, helping lenders decide whom to extend credit to.
- Product Development: Design financial products that cater more closely to consumer needs.
- Geographic Analysis: Examine how location influences lending outcomes, which is vital for regional market strategies.
- Credit Profiling: Leverage detailed borrower profiles to tailor credit offerings and improve marketing strategies.
Although loan rejection data may not be directly available, by analyzing loan origination data, financial experts can infer patterns related to rejections by examining variables such as credit scores and income brackets. This insight is critical for assessing market dynamics and borrower behavior.
Technological Advances
The growth of consumer behavior data has been accelerated by technological innovations such as Big Data analytics, machine learning, and cloud computing. These technologies facilitate processing large volumes of information, extracting meaningful insights, and enhancing decision-making processes in real time.
With growing data platforms like AWX and SNOW offering repositories and data processing capabilities, businesses are increasingly able to tap into extensive datasets on consumer loans. This acceleration makes it easier to contextualize consumer behavior within broader financial trends.
As technology continues to advance, the potential for richer, more actionable insights from consumer behavior data will expand, enhancing our understanding of financial processes and borrower patterns.
Conclusion
The transformative power of data in the financial sector is undeniable. By harnessing the wealth of information available through consumer behavior and loan origination data, businesses can gain unparalleled insight into loan rejection rates, an area once shrouded in mystery and conjecture.
Access to diverse types of data empowers financial professionals to make informed decisions, create innovative credit products, and develop targeted strategies to improve customer satisfaction and retention rates. The more data-driven an organization becomes, the more agile and responsive it can be in the face of market changes.
In a rapidly evolving financial landscape, data-driven decision-making is not just advantageous but essential. Organizations that invest in robust data strategies and embrace continuous learning from data insights are well-positioned to thrive in the competitive financial marketplace.
As companies consider data monetization and the potential of existing, untapped data assets, the financial industry is set to witness an exciting phase of innovation.
Looking forward, we can anticipate new data types emerging—from cryptocurrency transaction records to real-time financial market analytics—that offer even richer insights into loan processes and consumer behavior, redefining the future of finance.
Appendix
The financial industry comprises a diverse set of roles and stakeholders who can greatly benefit from enhanced data insights. Professionals such as investors, consultants, and insurance companies all leverage data to understand market dynamics and make informed decisions.
In particular, market researchers and analysts rely on consumer behavior and loan origination data to understand financial trends and consumer needs. By doing so, they can develop strategies that anticipate future market movements, securing competitive advantage.
Furthermore, AI holds the potential to unlock value buried within decades-old documents, transforming raw data into strategic insights that fuel future innovation.
As AI and machine learning technologies continue to evolve, their ability to analyze large datasets will lead to deeper insights and more precise predictions in financial sectors, revolutionizing how businesses interact with customers and assess risk.
The intersection of old and new data, coupled with advanced analytical technologies, heralds a new era for financial decision-making, one characterized by greater efficiency, accuracy, and innovation.
In summary, the future of the financial industry lies in its ability to capitalize on the power of data. Organizations equipped with the right tools and strategies will unlock new opportunities, delivering greater value to consumers and stakeholders alike.