Harnessing Credit Data for Consumer Loan Insights
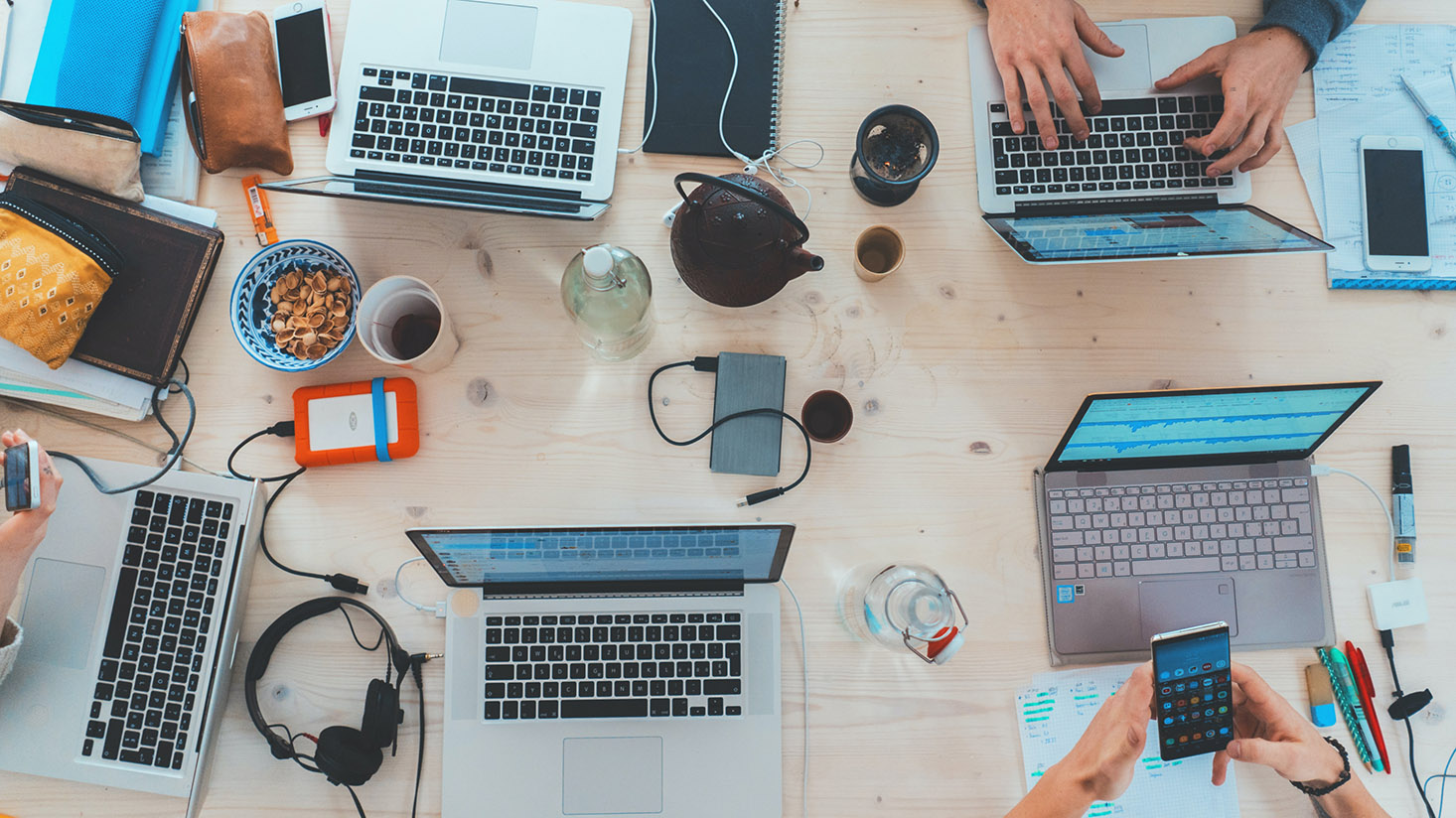
Introduction
In the ever-evolving financial landscape, the ability to glean meaningful insights from a sea of data is more paramount than ever. Historically, understanding trends and behaviors within the realm of small-dollar consumer loans was quite challenging. Lenders and financial analysts often grappled with a lack of detailed information, relying on broad, macroeconomic indicators or periodic financial reports, which were often outdated by the time they became available. Before the advent of comprehensive data solutions, financial institutions used traditional methods, such as in-person interviews or paper-based financial records, that provided limited insight into borrower behavior.
Data acquisition was often an arduous task, with firms collecting information manually or through sporadic reports. This scenario left decision-makers in the dark, struggling for a precise understanding of borrower performance and trends. Such delays could span weeks or even months, hindering the timely and informed decision-making crucial to the financial sector.
With the rise of digital solutions, the implementation of sensors, the internet, and connected devices, as well as software advancements, accessing real-time data has become significantly simpler. Technology has seamlessly integrated into financial processes, transforming every transaction, every repayment, into actionable insights stored in expansive databases.
In today's fast-paced world, the importance of data for understanding financial health and loan performance cannot be overstated. Leveraging data allows lenders to gain a minute-by-minute understanding of changes and patterns in borrower behavior, vastly improving response time and accuracy.
The shift from being data-poor to data-rich has empowered financial professionals in ways previously considered impossible. They can now easily track dynamic shifts in consumer lending trends, evaluate risk with greater precision, and implement strategic changes with confidence.
In this article, we will explore various types of data that play a critical role in understanding time series banking data for small-dollar consumer loans, enabling financial decision-makers to optimize strategies and improve outcomes.
Consumer Behavior Data
History and Evolution
The emergence of consumer behavior data has revolutionized the financial services industry. This type of data offers a granular, detailed view of consumer credit activities, such as trade line or loan level information. Historically, consumer credit data were heavily aggregated, limiting their utility for nuanced insights.
Consumer behavior data has evolved over the past two decades, moving from paper records to digital formats, with monthly histories that can date as far back as 2005. Advances in data storage and processing technologies have made these datasets increasingly accessible and valuable.
Various industries, especially lenders, credit rating agencies, and financial analysts, have historically utilized consumer behavior data to make informed decisions. They use it to evaluate creditworthiness, predict default risks, and form a strategic understanding of consumer debt patterns.
Technology's Role
Technological advances have significantly accelerated this type of data. The integration of advanced algorithms and machine learning has allowed for more precise modeling of income and financial health metrics, paving the way for more accurate lending decisions.
The proliferation of cloud computing and big data technologies has further enabled the collection and analysis of consumer behavior data on an unprecedented scale, offering detailed insights into both individual and aggregate loan performance metrics.
Applications to Consumer Loans
Consumer behavior data is pivotal in understanding small-dollar consumer loan insights. The ability to identify specific loan categories, such as small-balance consumer loans, and then analyze their performance over time can shape lending strategies significantly. Here are some specific applications:
- Delinquency Tracking: Data on repayment history and delinquency status allows lenders to monitor borrower behavior and take preemptive action if necessary.
- Risk Assessment: Predictive analytics models, based on historical data, can evaluate the potential risk associated with lending to different consumer segments.
- Income Modeling: By modeling changes in consumer income over time, lenders can better assess repayment capabilities at loan origination and throughout the loan tenure.
- Identifying Borrower Trends: By analyzing consumer behavior data, financial institutions can discern larger trends in consumer borrowing and spending, adapting their offerings accordingly.
- Behavioral Segmentation: Detailed data enables the segmentation of consumers based on their credit behavior, improving targeted financial product marketing.
Conclusion
In conclusion, the availability and utilization of detailed consumer behavior data provide invaluable insights into the world of small-dollar consumer loans. Access to this data revolutionizes the way financial professionals approach lending, risk assessment, and market adaptation.
Data-driven decision-making has become a cornerstone of the modern financial landscape. By embracing data, organizations can significantly enhance their ability to predict, respond, and succeed in an increasingly competitive market.
Furthermore, as financial institutions generate and collect more granular data, the potential for data monetization grows exponentially. Corporations are gradually recognizing the value in potentially untapped data resources they have been generating, and the trend towards monetizing such data is set to gain momentum.
Looking ahead, newer forms of data might emerge, providing insights into aspects of consumer finance previously thought as unquantifiable. Data related to social media behaviors, lifestyle patterns, and even ecological footprints might contribute to a more holistic understanding of consumer credit behavior.
In a future where data is king, businesses that embrace a data-centric strategy and commit to continuous data discovery will undoubtedly stay ahead of the curve.
Appendix
The potential beneficiaries of insights derived from consumer behavior data extend far beyond the obvious financial institutions. Investors, consultants, and insurance companies are among the many who stand to gain.
For investors, data insights can inform better investment strategies, allowing them to understand market dynamics and consumer spending patterns.
Consultants can leverage the insights to advise their clients on optimizing lending processes, improving customer retention, and identifying opportunities in underserved markets.
Insurance companies benefit by assessing risk profiles with greater accuracy, tailoring insurance products that cater to specific consumer needs.
Looking towards the future, AI offers vast potential to unlock insights from decades-old data and modern government filings, driving a new wave of innovation in finance.
The rise of external data platforms has made it easier for businesses to access diverse datasets, broadening their analytical capabilities and enhancing decision-making processes. The roles of these data-enriched industries will likely expand as more organizations recognize the value in becoming data-driven.