Harnessing Healthcare Data for Mortality Insights in North America
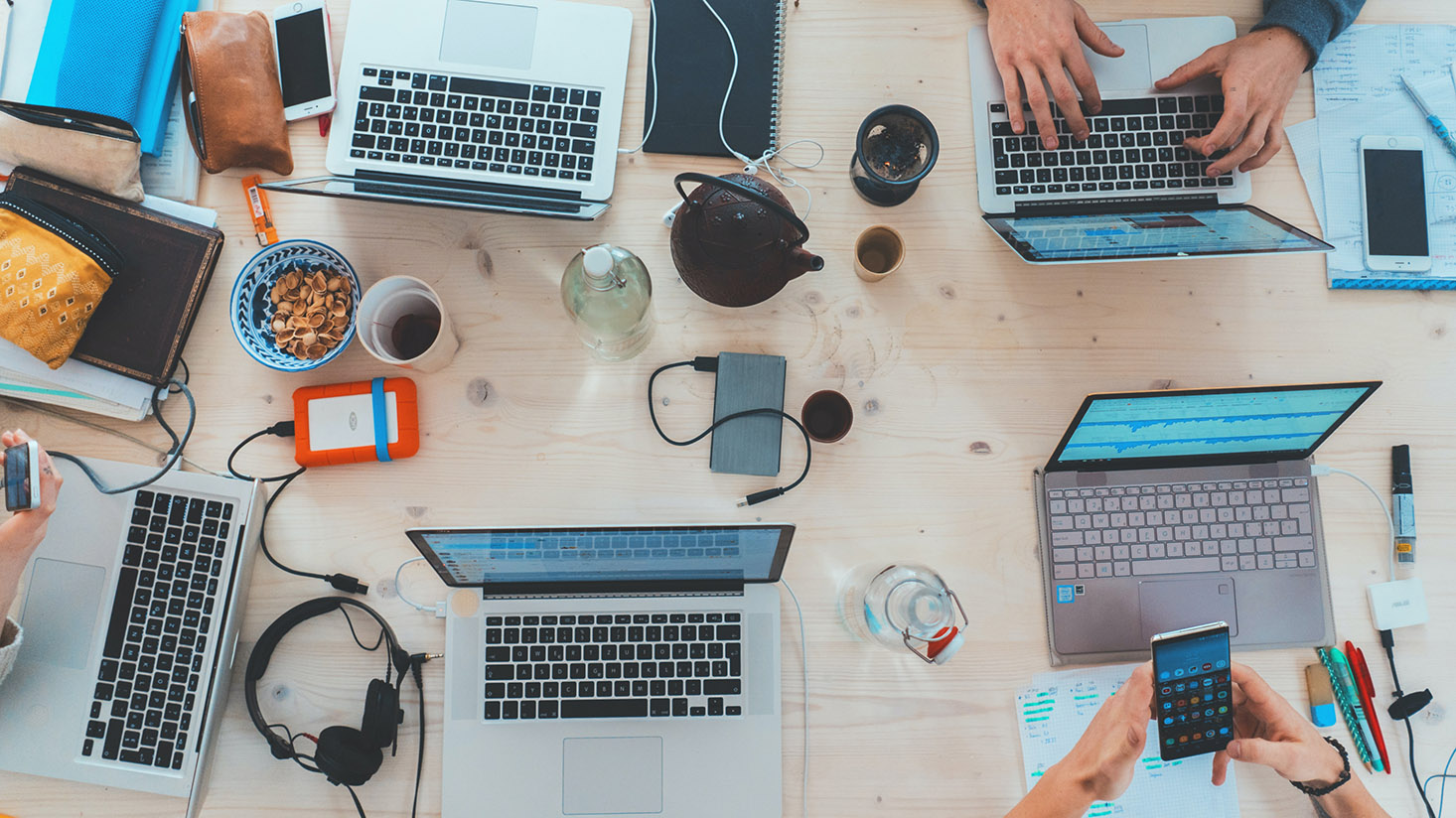
Introduction
The quest to unveil the mysteries behind mortality rates in the US and Canada has been fraught with challenges, largely due to the historical limitations in data acquisition and analysis. Before the era of big data and interconnected systems, organizations, especially in the life insurance sector, struggled to glean meaningful insights into mortality patterns. The reliance on periodic demographic surveys and officially published mortality tables meant that companies operated with delayed insights, akin to navigating through a fog with an outdated map.
Before the widespread availability of comprehensive datasets, businesses relied heavily on national health registries and periodic reports. These provided a snapshot view but lacked the granularity and real-time relevance needed for dynamic decision-making. Before the ascension of data-driven methodologies, insurers would depend on simple actuarial tables that were updated infrequently. This method provided a broad understanding but couldn't capture nuances such as the impact of lifestyle factors on mortality.
As technology advanced, the landscape began to shift dramatically with the advent of sensors, the Internet, and connected devices. This technological tide brought about an acceleration in the volume and fidelity of data being gathered, from wearables monitoring personal health metrics to robust databases cataloging health insurance claims and medical records. This data deluge opened new frontiers in analyzing mortality rates, equipping professionals with the ability to track trends and patterns in real-time.
The exponential growth in data availability has afforded companies a comprehensive lens through which to assess mortality. What once took months to comprehend now takes mere moments, thanks to real-time data assimilation and analytics. The shift from retrospective to predictive analytics has empowered professionals to forecast and adapt strategies proactively.
The advent of comprehensive datasets has proven transformative, enabling a more nuanced understanding of mortality rates that incorporates variables such as physical activity, demographics, and disease prevalence. For the first time, organizations can make informed predictions on mortality, offering more personalized services and solutions. Different types of data and sources provide unique insights, each contributing a piece to the larger puzzle.
Now, as companies bask in the glow of immediate insights, they are better positioned to discern the impacts of various factors on mortality. With robust data at their disposal, they now possess the tools to venture beyond simple descriptives and towards prescriptive and predictive solutions. The historical barriers have crumbled, paving the way for a data-empowered future in understanding and addressing mortality metrics.
Healthcare Data
Healthcare data has emerged as a cornerstone in the pursuit of understanding mortality rates in North America. Historically, healthcare data was collected through manual records kept by individual providers, which were prone to inaccuracies and rarely shared across systems. The creation of centralized digital databases, however, changed the game by aggregating vast amounts of data from various healthcare settings.
Healthcare data encompasses a range of information, such as electronic medical records (EMR), pharmacy data, and claims transactions. These datasets offer deep insights into patient histories, treatments, and outcomes. For example, an EMR contains detailed patient information, including diagnoses, medications, treatment plans, immunization dates, and laboratory results.
Technological advances such as blockchain, secure cloud environments, and tokenization have propelled the availability and usability of healthcare data. These innovations ensure the privacy of sensitive data and facilitate the integration of disparate datasets. The speed and scope of data collection and processing have significantly accelerated, providing more comprehensive views of patient health as it relates to mortality.
Specifically, external data from healthcare providers have immense potential in understanding mortality trends. With access to vast backlogs of healthcare records, insurers and healthcare stakeholders can delve into disease prevalence and lifestyle factors influencing mortality. Such data, when coupled with mortality data, can be crucial in identifying patterns and correlations that were previously invisible.
Applications of Healthcare Data
- Identifying Health Trends: By analyzing historical healthcare data, insurance companies can identify trends in health issues that correlate with mortality, allowing them to tailor their products accordingly.
- Risk Assessment and Management: Detailed healthcare data helps insurers perform more accurate risk assessments, ensuring premiums are aligned with actual health risks.
- Predictive Analytics: Utilizing machine learning models on this data helps insurers predict future mortality rates based on current health trends and demographics.
- Policy Development: Granular data can inform public health policy development by identifying at-risk populations and designing targeted interventions.
- Personalized Healthcare: As healthcare data becomes more individualized, patients can receive more personalized treatment plans, potentially reducing mortality rates.
Healthcare data is not just about improving business models; it's a tool for driving better health outcomes across populations, illuminating the path to longer, healthier lives.
Conclusion
In this evolving landscape, understanding mortality rates in North America via healthcare data is crucial. It not only supports the strategic positioning of businesses but also the health and well-being of individuals across the continent. Data has become the linchpin in deciphering the complex interplay between lifestyle and mortality rates.
Organizations must continue to invest in becoming more data-driven, harnessing the plethora of available data types to fuel their decision-making processes. As data becomes an increasingly valuable commodity, companies will likely look to monetize their data, capitalizing on the informative potential of the databases they've amassed over the years.
The future of data in mortality insights looks promising, with new intersections of data sources that could pave the way for innovative assessments and policy decisions. As we look ahead, companies might generate new datasets from smart devices and home health technologies, turning these insights into actionable strategies to combat high mortality rates.
The role of AI could prove transformative, using advanced algorithms to uncover deeper correlations from existing data. The focus on training data is essential, allowing AI to be more effective in drawing conclusions that were previously difficult to ascertain.
Conclusively, while the path to understanding mortality through data is laden with challenges, it's equally rife with opportunities. As the ecosystem of healthcare data expands, so too does our ability to comprehend and mitigate the factors contributing to mortality, crafting a future where insights can save lives.
Appendix
A diverse array of roles and industries can greatly benefit from the insights that healthcare data offers on mortality rates. Investors, seeking to back life sciences innovations, utilize this data to predict market opportunities in healthcare advancements. Insurance companies leverage these insights to refine underwriting processes, improving risk management and optimizing premium strategies.
Consultants aim to harness this data to advise healthcare systems on improving outcomes while reducing costs, identifying inefficiencies, and pinpointing areas ripe for innovation and policy improvement. Market researchers delve into these datasets to understand epidemiological trends, informing healthcare marketing strategies and public health education campaigns.
Insurance companies, particularly life insurers, have undergone a data-driven transformation. Such insights enable them to create more tailored products, manage their risk portfolios more effectively, and ultimately offer more competitive and fair policies to their clients.
In the future, the continued integration of AI and healthcare data promises even more revolutionary changes. AI will likely unlock latent insights in existing datasets and make sense of unstructured historical data, transforming predictions about mortality rates into definitive strategies for life extension and quality improvement.
The synergy of AI and data promises a robust frontier for discovering insights that were previously intangible. As data analytics becomes more sophisticated, insurance companies will find themselves better equipped to provide value to their clients and shareholders alike.
Ultimately, the transformation of traditional methods by modern data technologies signifies a hopeful path forward. Increased accuracy in mortality predictions can drive improvements in public health policy and individual healthcare, illustrating the indispensable role of data in our society's progression towards healthier living.