Harnessing Insights with Soybean Processing Data
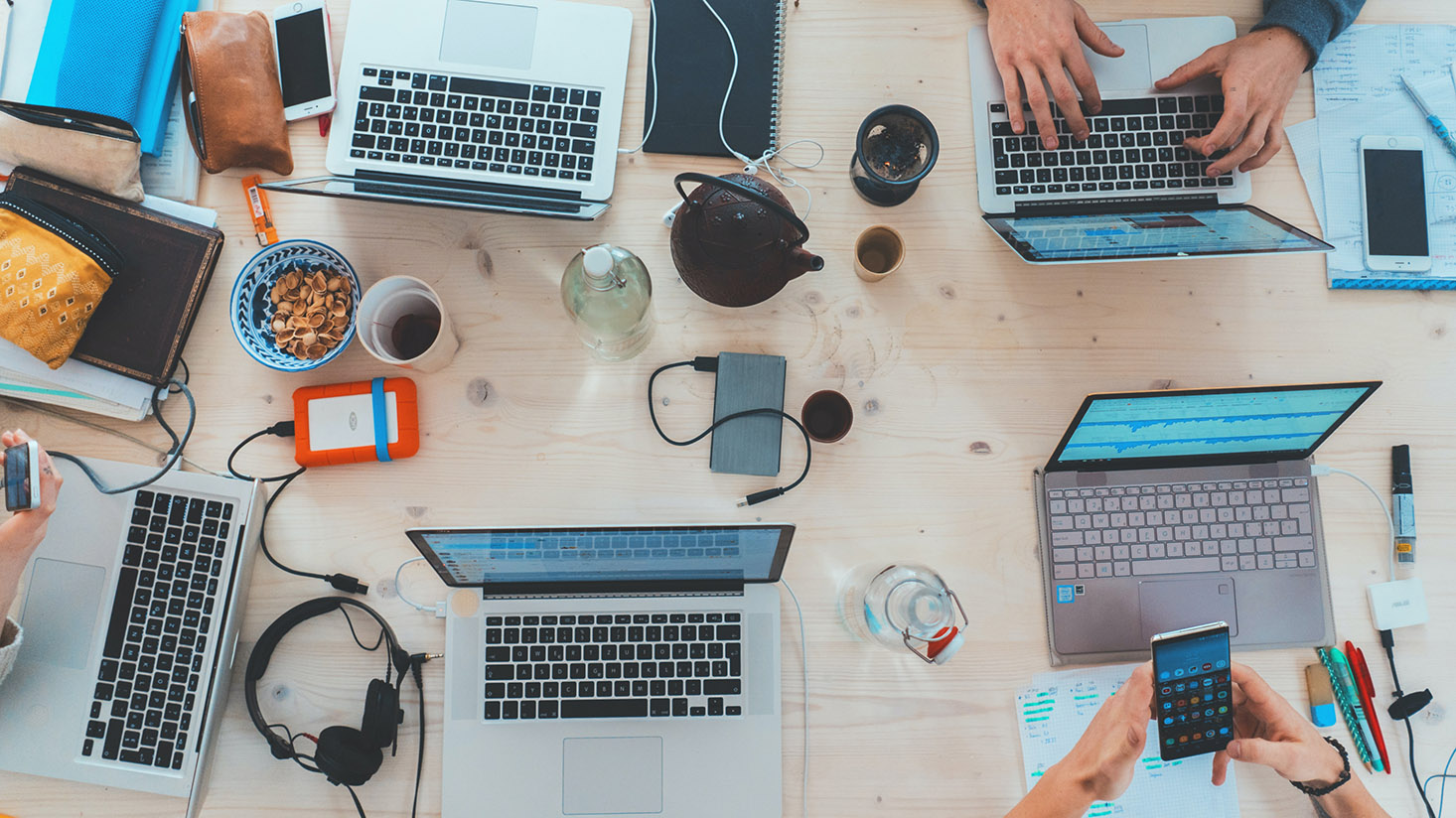
Introduction
The realm of soybean processing has long been an intriguing, yet challenging, territory for businesses seeking substantial insights. Historically, acquiring accurate and timely data about soybean processing facilities, their purchase volumes, sales, and strategies posed significant hurdles, acting like a complex enigma waiting to be decoded. In a world that craved speed and efficiency, firms found themselves often mired in outdated, imprecise data collection methods.
Before the fusion of technology with data-driven insights, businesses relied heavily on antiquated methods such as manual surveys, anecdotal evidence, and estimates to gauge the operations and market impact of soybean processing plants. These methods offered rudimentary snapshots without the comprehensive understanding required for strategic decision-making. The landscape was fraught with uncertainties, as data was scattered, inconsistent, and sometimes outright misleading.
The advent of digitalization transformed this scenario almost overnight. Sensors, the Internet, and interconnected devices became vital instruments in modernizing data collection in the soybean processing sector. Suddenly, data wasn’t just about numbers; it was a story of trends, anomalies, and real-time shifts, easily accessible through robust databases. The wave of digital integration ushered in a new era where every tiny event at a processing plant could be captured, stored, and analyzed for actionable intelligence.
With the proliferation of software across various processes, firms could accumulate vast datasets detailing every nuance of their operations. Gone were the days of being left in the dark, waiting periods stretching into weeks or even months to understand changes in soybean processing plants. Real-time data empowered professionals to make informed decisions and strategically adjust their operations, often before potential challenges escalated into detrimental problems.
Today, data has become the backbone of understanding the complexities of soybean processing. It acts as an indispensable tool, illuminating trends, optimizing processes, and unlocking opportunities that were previously concealed within layers of fragmented and uncoordinated data elements. The industry stands at a pivotal threshold where data-driven strategies and insights can revolutionize age-old practices.
For professionals invested in the soybean industry, the question isn’t whether data is necessary—it’s how to accurately harness it for transformative decisions and strategies. By leveraging various categories of data, the industry now has the opportunity to breakdown barriers and rebuild approaches that favor nuanced understanding and sustainable growth.
Commodities Data
Commodities data, encompassing global seaborne trades, is a treasure trove for those immersed in soybean processing. These datasets have evolved from mere transactional records to become pivotal analytical tools capable of revealing intricate trade flows, including volumes, load/discharge locations, buyers, and sellers.
The history of commodities data is rooted in its ability to conceptualize the movement of goods. In earlier times, this data was primarily captured through shipping manifests and trade-ledger records maintained across global ports. With each passing decade, however, commodities data carved its niche as technology progressed, enabling real-time monitoring of trade movements and transactions.
Today, professionals across numerous sectors—ranging from agribusiness to logistics—rely heavily on commodities data to navigate the interconnected world markets. Technological advancements such as satellite tracking and enhanced reporting capabilities have further brought granularity and precision into this data category, assisting industries in remaining agile amidst global shifts.
The acceleration in the amount of data available in the commodities sector is unprecedented. Automated systems capture the nuances of every trade, ensuring that data sets remain up-to-date and rich with actionable insights. This wealth of information facilitates understanding of the ever-evolving landscape of soybean processing at multiple operational levels.
**Uses of Commodities Data in Soybean Processing:**
- Real-Time Trade Monitoring: Track the global movement of soybeans to identify trends and forecast potential supply chain disruptions.
- Volume Analysis: Analyze shifts in trade volumes to adjust processing strategies accordingly.
- Regional Insights: Discover how specific regional dynamics affect trade routes and volume outputs.
- Buyer-Seller Dynamics: Identify major players influencing market dynamics and trade relationships.
- Predictive Analytics: Use historical data to model and predict future trade patterns, aiding strategic planning.
Industrials Data
Industrial data is another invaluable asset for comprehending the operational dynamics within soybean processing facilities. This data category speaks to real-time monitoring technologies examining aspects such as processing efficiency, equipment outages, and comparative analyses of national and regional facilities.
Historically, industrial data collection was a manual endeavor, with facility managers providing periodical reports detailing operational metrics. The advent of cutting-edge industrial technology has, however, reinvented this data category, transforming it into an intricate illustration of the processing ecosystem.
Industries ranging from manufacturing to energy have extensively utilized industrial data to elevate performance and optimize operations. The significance of this category lies in its precision, capacity for real-time monitoring, and ability to detect anomalies, ensuring facilities can operate at peak efficiency while minimizing downtime.
In the context of soybean processing, the importance of industrial data cannot be overstated. The acceleration of data-enabled monitoring means that insights into operational dynamics can be realized almost instantaneously. Facilities now have the capability to not only monitor but anticipate and react to anomalies that could impede productivity.
**Applications of Industrials Data in Soybean Processing:**
- Operational Efficiency Monitoring: Dodge low operational periods through accurate assessments of processing efficiency.
- Equipment Outage Insights: Utilize data on equipment outages to implement predictive maintenance schedules.
- Real-Time Optimization: Adjust processing tasks in real-time for optimal production flows.
- Comparative Analysis: Compare facility performance against national averages and identify areas for improvement.
- Processing Rate Predictions: Employ processing models to forecast rates and optimize resource allocation.
Agricultural Data
Agricultural data offers a valuable extension into the broader realm of commodity and industrial data, focusing sharply on the nuances of soybean purchases, sales, and operational shifts.
Traditionally, data on agricultural trade was gathered painstakingly through farmer reports and market analyses—often long after transactions occurred. Fortunately, with advancements in digital reporting and transaction tracking, these datasets have become ubiquitously precise, offering real-time insights into agricultural activities.
Roles within agriculture, finance, and research have all greatly benefited from the robust agricultural data now accessible. Companies positioned to analyze this data are able to draw upon it to refine business strategies, developing deeper understandings of market forces and impacts on production and pricing layers.
As agricultural data continues to multiply in volume and accessibility, professionals engaged in soybean processing stand to gain significantly. The category itself has expanded beyond mere transaction tracking to include comprehensive insights into purchasing patterns, sales dynamics, and market changes.
**Agricultural Data Applications in Soybean Processing:**
- Purchase Patterning: Track and understand purchasing volumes and patterns for proactive market engagement.
- Sales Dynamics Assessment: Analyze sales trends to strategize pricing and market approaches.
- Market Impact Analysis: Explore how global and regional agricultural trends influence soybean sales and purchases.
- Company Performance Review: Examine data by company to draw comparisons and identify industry leaders.
- Predictive Modeling: Build predictive models to forecast changes in processing and trading activities.
Conclusion
In summary, using external data, including commodities, industrial, and agricultural datasets, stands as a transformation pillar for the soybean processing sector. Access to diverse data types now empowers businesses with unprecedented insights, enabling them to make informed, strategic, and swift decisions.
Organizations must increasingly embrace data-driven avenues as an inevitable strategy to navigate complexities while uncovering hidden potentials within their operations. As a result, the ability to discover high-value data is integral, creating a ripple effect that can reverberate across the entire soybean processing landscape.
In a hyper-connected world where data monetization is becoming more prevalent, organizations seek to monetize their data assets, developing products to meet specific market needs. The soybean processing sector is no exception, likely giving rise to new data-driven services and innovations in the near future.
Potential breakthroughs in data utilization will propel the industry forward, with organizations possibly tapping into unexplored datasets to derive fresh insights. Possibilities might include sensor-generated data or Artificial Intelligence-based interpretations to transform traditional operations into dynamic, responsive entities.
Overall, the shift to data-centric operations represents an exciting frontier for soybean processing, primed to rewrite the rules and redefine industry paradigms. For industry professionals, data discovery and implementation can no longer be separate considerations—they must exist as an integral and harmonized approach to thrive amidst evolving business dynamics.
Appendix
If we explore the types of roles and industries that could benefit from soybean processing data, it becomes evident that the spectrum is vast and multifaceted. From investors to consultants, the applications of data in this sector can bring transformative changes.
In the investment realm, having access to timely and precise data can unveil market opportunities. Investors can anticipate market shifts, develop strategic alignments, and minimize risks, all enabled by granular insights from comprehensive datasets.
Consultants, on the other hand, can leverage data to provide clients structured strategies that align with broader industry trends and forecasts. With detailed operational data, they can craft bespoke solutions that address client-specific challenges.
Industries such as insurance and risk management have also seen profound impacts from external data. Accurate risk assessments and pricing models can now be created, driven by historical data and trend analysis that traditional methods couldn't furnish.
The realm of market research opens further possibilities, where researchers can blend various categories of data to unearth nuanced insights, bring innovation, and refine products tailored to the ever-shifting consumer landscape.
Heading into the future, the intersection of AI and data holds vast potential to unlock hidden values buried within existing and historic datasets. The ability to digitize and interpret old documents alongside modern filings could revolutionize operations, allowing for predictive analytics and unprecedented granular analyses.