Investment Firm AUM Insights
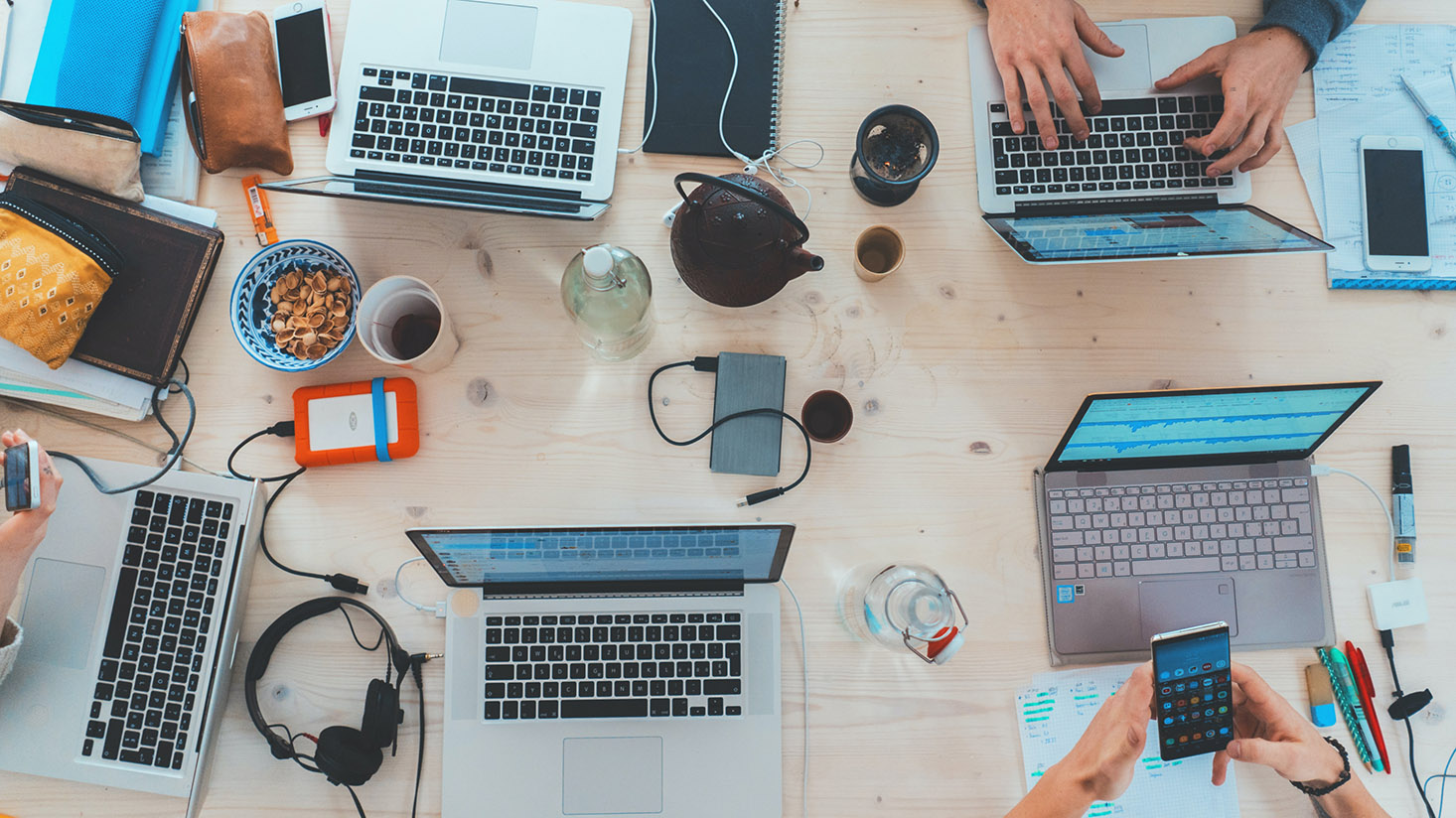
Introduction
Understanding the landscape of investment and wealth management firms, particularly in terms of assets under management (AUM), customer types, headcount, and revenue, has historically been a complex endeavor. Before the digital age, insights into these areas were primarily derived from manual surveys, financial reports, and industry hearsay. These methods were not only time-consuming but often resulted in outdated or inaccurate data by the time they were compiled and analyzed. The advent of sensors, the internet, and connected devices, alongside the proliferation of software and databases, has revolutionized the way data is collected, stored, and analyzed, making real-time insights a reality.
Previously, professionals in the financial sector had to rely on antiquated methods such as paper-based records, direct communications, and public filings to gather information on investment firms. This often meant weeks or months of waiting for data that could be quickly outdated, leaving decision-makers in the dark about current trends and opportunities. The introduction of digital data collection and analytics tools has dramatically changed this landscape.
The importance of data in understanding the dynamics of investment firms cannot be overstated. With the right datasets, business professionals can now track changes in AUM, analyze investment trends, and gain insights into the operational aspects of these firms in real-time. This shift towards data-driven decision-making has enabled a more agile and informed approach to investment and wealth management.
The transition from traditional to digital data sources has been facilitated by several technological advances. The development of sophisticated data analytics platforms, the widespread adoption of cloud storage, and the integration of artificial intelligence and machine learning algorithms have all played a part in transforming the availability and usability of data.
As a result, the amount of data available for analysis has accelerated exponentially. This surge in data availability has opened up new avenues for understanding the intricacies of investment management, from detailed AUM breakdowns by customer type to comprehensive firmographic profiles.
The shift towards a more data-centric approach in the financial sector highlights the growing need for accurate, timely, and detailed data. As we move forward, the role of data in shaping investment strategies and understanding market dynamics will only continue to grow, making it an indispensable tool for business professionals.
Financial Markets Data
The realm of financial markets data is vast, encompassing a wide range of information critical to understanding investment and wealth management firms. Historically, access to detailed data on AUM, investment strategies, and firmographic information was limited. However, technological advancements have paved the way for comprehensive databases that aggregate and analyze financial markets data, providing unprecedented insights into the operations and performance of investment firms.
Examples of financial markets data include AUM information, investment decision-maker contacts, and detailed account-level data. This type of data is invaluable for a variety of roles and industries, including investors, market researchers, and financial analysts, who rely on accurate and up-to-date information to make informed decisions.
The technology advances that have enabled the collection and analysis of financial markets data include sophisticated data aggregation platforms, advanced analytics tools, and the integration of artificial intelligence for predictive modeling. These innovations have not only increased the volume of data available but have also improved the quality and relevance of the insights derived from it.
The acceleration in the amount of financial markets data has opened up new possibilities for understanding investment firms. Business professionals can now:
- Track AUM changes in real-time, allowing for timely investment decisions.
- Analyze investment trends and strategies to identify market opportunities.
- Gain insights into firmographic profiles, including headcount and revenue estimates, to assess the health and growth potential of investment firms.
- Access detailed contact information for key decision-makers, facilitating direct engagement and networking opportunities.
These capabilities have transformed the way business professionals approach investment and wealth management, enabling a more data-driven and strategic perspective.
Financial Data
Another critical category of data relevant to understanding investment firms is financial data. This encompasses a broad spectrum of information, including hedge fund AUMs, rates of return, and comprehensive data on fund managers and investors. The history of financial data is marked by a gradual shift from fragmented and inaccessible information to consolidated and easily accessible databases.
Financial data is used by a wide range of professionals, from financial analysts and portfolio managers to market researchers and consultants. The technology advances that have facilitated the collection and analysis of financial data include the development of financial data aggregation services, the use of cloud computing for data storage and processing, and the application of machine learning algorithms for data analysis.
The amount of financial data available has seen significant growth, driven by the increasing complexity of financial markets and the demand for more granular insights. This data can be used to:
- Compare hedge fund performance, enabling investors to make more informed choices.
- Track investments made by fund managers and wealth managers, providing insights into market trends.
- Analyze firm-wide AUM and breakdowns by customer type, offering a deeper understanding of investment firm dynamics.
- Access comprehensive firmographic information, including headcount and revenue estimates, to evaluate firm performance and growth potential.
These insights are invaluable for business professionals looking to navigate the complex landscape of investment and wealth management.
Conclusion
The importance of data in understanding investment and wealth management firms cannot be overstated. With access to a wide range of data types, including financial markets data and financial data, business professionals can gain comprehensive insights into the operations, performance, and strategies of investment firms. This data-driven approach enables more informed decision-making, strategic planning, and competitive analysis.
As organizations become increasingly data-driven, the ability to discover and leverage relevant data will be critical to success. The future of data in the financial sector is likely to see even more innovative uses, as companies look to monetize the vast amounts of data they have been generating. This could include new types of data that provide additional insights into investment firm dynamics and market trends.
The role of data in shaping investment strategies and understanding market dynamics will continue to grow, making it an indispensable tool for business professionals. The ability to access and analyze a wide range of data types will be key to staying competitive in the rapidly evolving financial sector.
Appendix
The transformation brought about by data has impacted a wide range of roles and industries, including investors, consultants, insurance companies, and market researchers. These professionals face various challenges, from identifying investment opportunities to assessing market trends and firm performance. Data has revolutionized the way these challenges are addressed, providing real-time insights and enabling more strategic decision-making.
The future of data in the financial sector is likely to see further innovations, with artificial intelligence and machine learning playing a pivotal role in unlocking the value hidden in decades-old documents and modern government filings. This will open up new avenues for understanding investment and wealth management firms, driving further advancements in data-driven decision-making.