Leverage Diverse Data Sources to Analyze Input Costs and Product Sensitivity
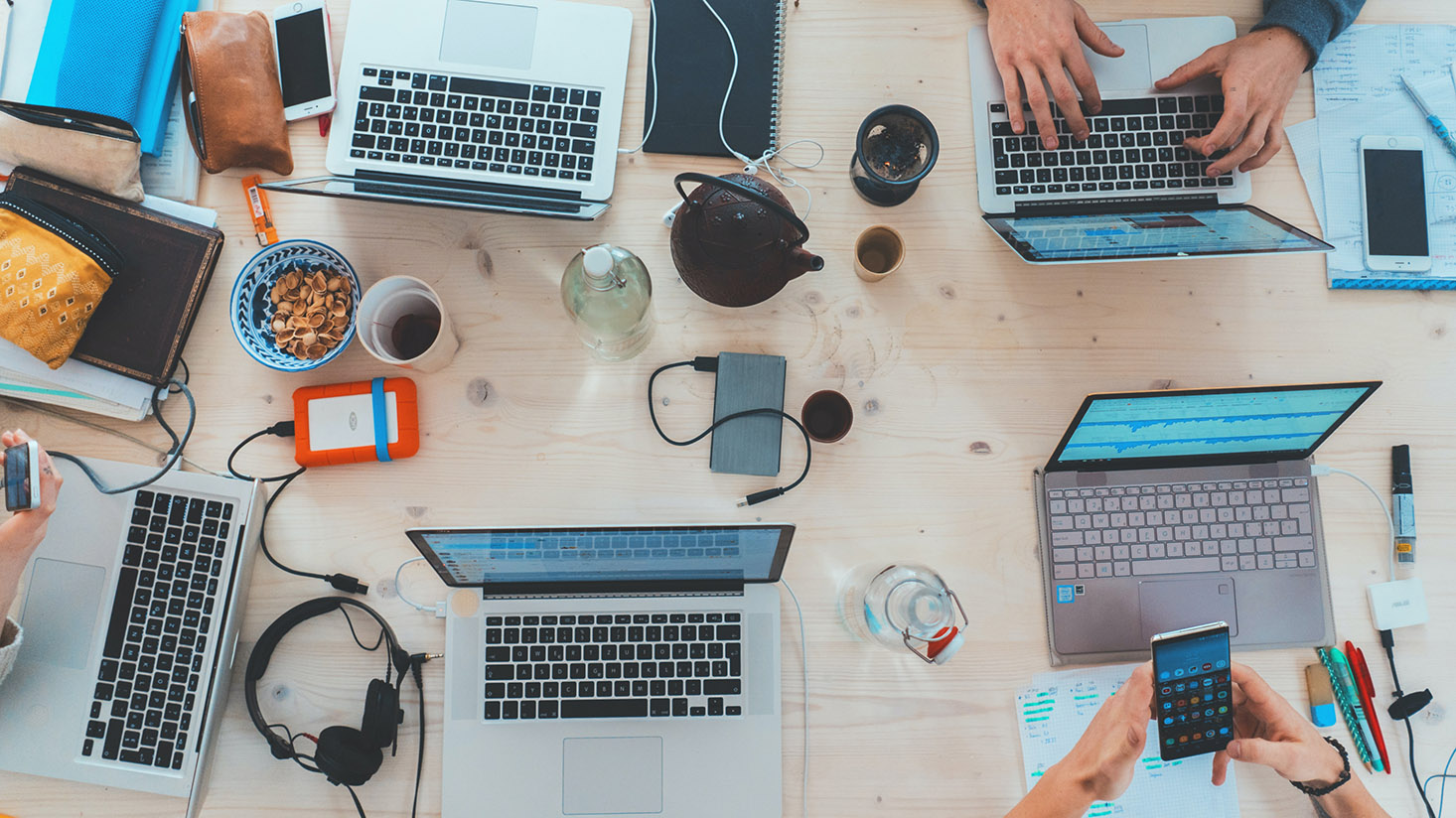
Introduction
The connectivity of modern economies has made understanding the dynamics of production costs and the impact on finished goods more critical than ever. Historically, acquiring insights into the cost of inputs and their sensitivity on finished products was a convoluted task, often riddled with inaccuracies and inefficiencies. Firms relied heavily on rudimentary methods, such as manual surveys and delayed fiscal reports. These antiquated means were subject to human error and delayed release cycles, leading to businesses running on outdated information.
Before the digitization of data, enterprises had to depend on anecdotal evidence and intuition to make crucial economic decisions. This lack of concrete data often resulted in companies being caught off guard by sudden shifts in market dynamics. It wasn't uncommon for firms to wait weeks, if not months, for detailed reports on input costs. By then, the tides would have already changed, eradicating any competitive advantage they sought.
The advent of external data, much of it driven by the proliferation of technology, has revolutionized the way businesses process information. The installation of sensors, the growth of the internet, and the interconnection of devices have made it simpler and quicker to obtain real-time data. Software solutions have cemented their place in processes worldwide, capturing every interaction, transaction, and event, promptly dumping it into expansive databases.
With today's data-rich environment, it’s become possible to meticulously track variables affecting the cost of inputs and their resultant impact on product pricing. This transformation aids businesses in pivoting strategies almost instantaneously, leveraging real-time information to stay ahead of the competition. Informed decisions based on accurate, current data enable firms to optimize operations, strategize effectively, and buffer against unexpected economic changes.
In a world steeped in data, businesses can gain deep insights with unprecedented efficiency. Recognizing the importance of data in understanding costs and product sensitivity is not merely advantageous; it’s essential. With timely intelligence, businesses not only remain competitive but can ambitiously redefine market standards.
In this article, we will explore how various categories of data contribute immensely to understanding these complexities. We will delve into the historical evolution of distinct data types, their present application, and the potential insights they offer.
Financial Data
The discipline of financial data analytics has made remarkable strides over the past few decades. As business landscapes have evolved, the importance of utilizing comprehensive data to understand input costs and product price sensitivity has soared. Financial data, ranging from commodity prices to sector-specific financial reports, has become a cornerstone in illuminating these economically pivotal dynamics.
Financial data encompasses statistics on commodities, exchange rates, and market indices, offering insights into both macro and microeconomic conditions. Traditionally, financial data primarily resided in quarterly or annual reports, with analysts laboring tirelessly to interpret dense, jargon-laden documents. This information, though often vital, was only accessible after the fact, delaying actionable business interventions.
With technological innovations, notably advancements in AI and natural language processing (NLP), the accessibility of training data has been redefined, elevating financial data's role in corporate strategies. Today, institutions can leverage real-time data feeds to instantly obtain metrics on crucial commodities, allowing for rapid reaction to market events. The integration of AI has enhanced predictive capabilities, providing foresight into input cost variability and its potential implications on end product pricing.
Industries using financial data to navigate input costs cover a wide spectrum — from manufacturing to consumer goods. Each sector keenly monitors such data to mitigate risks linked to fluctuating costs of commodities and services.
Applications of Financial Data:
- Analyzing Commodity Prices: Real-time updates on raw material costs empower businesses to adjust budgets and pricing strategies proactively.
- Sector Reports: Industry-wide financial reports provide intricate details allowing for benchmarking and trend analysis.
- Exchange Rate Monitoring: Exchange rate fluctuations can directly impact material costs; tracking these changes facilitates strategic purchasing decisions.
- Influence of Policy Changes: Understanding fiscal policy shifts allows businesses to anticipate and prepare for cost changes.
- Predictive Analysis: AI-driven models help predict future market trends, allowing companies to refine supply chain operations.
Technology Data
The technology landscape has redefined how data is utilized across industries, including its pivotal role in understanding production costs and sensitivity factors for finished products. Technology data spans across diverse applications, from software usage statistics and digital communication log analytics to hardware performance metrics.
Historically, the collection and analysis of technology data were arduous, involving manual logging and periodic assessments conducted by technology officers. As digital transformation accelerated, the complexity and volume of technology data exploded, necessitating advanced tools for management and analysis.
Today, technology data thrives in digital ecosystems, facilitated by sophisticated platforms and tools that capture, process, and analyze datasets at unparalleled speeds. This data offers deep insights into operational efficiencies, downtime reductions, and cost optimization strategies. By integrating technology data, companies can fine-tune their operations and understand how these adjustments influence the broader economic landscape.
In industries such as manufacturing, retail, and services, technology data offers vital insights. Firms extensively utilize technology data to track input costs, equipment maintenance schedules, and system efficiencies, ultimately controlling production costs.
Applications of Technology Data:
- Software Analytics: It helps in evaluating software efficiency, indicating where a firm can reduce costs by optimizing tools.
- Hardware Performance: Analysis leads to better maintenance schedules and replacement strategies, minimizing downtime and costs.
- Digital Communication: Monitoring digital workflows helps evaluate productivity levels across departments and find cost-cutting opportunities.
- System Integrations: Efficient integrations reduce transactional costs and manual errors, leading to lower operational risks.
- Cloud Utilization: Insights into cloud service usage help optimize resources and budget allocation, directly affecting input costs.
Automotive Data
Automotive data has become increasingly crucial in understanding the nuanced variables affecting production costs and finished product sensitivities. From manufacturing schedules to raw material expenditures, automotive data provides detailed insights into every facet of the automobile production line.
Earlier, automakers relied heavily on historical reports and estimates, making it challenging to fine-tune production or cost components effectively. These legacy systems made adaptation slow, invariably affecting competitive standing in a rapidly evolving market. However, the integration of modern automotive data platforms has catalyzed change within the industry.
Automotive data encapsulates the wide array of variables that manufacturers must track to remain agile, ranging from component costs and labor charges to logistic expenses and technology deployment. Access to automotive data facilitates a keener understanding of input sensitivities, allowing firms to propose effective cost management strategies.
This comprehensive data supports better resource allocation, price negotiations with suppliers, and cost forecasting, essential in an environment where comprehension of cost dynamics dictates survival and success.
Applications of Automotive Data:
- Component Cost Analysis: Detailed data on parts manufacturing can reveal cost-saving opportunities through optimized sourcing.
- Production Schedules: Aligning production with real-time demand helps minimize excess inventory costs.
- Energy Efficiency: Tracking energy consumption at various production stages allows for cost reductions and sustainability improvements.
- Logistics Optimization: Data-driven logistic strategies ensure timely delivery of materials and finished products.
- Supply Chain Stability: Monitoring supply chain disruptions helps anticipate bottlenecks, thus securing input flows.
Conclusion
The analysis of diverse data types has become indispensable for modern businesses looking to uncover the intricate dynamics of input costs and finished product sensitivities. From harnessing AI-driven insights in financial data to leveraging technology datasets to optimize operations, these resources offer sharp competitive edges. Each dataset delivers an array of perspectives, enabling firms to strategize toward sustainable growth and profitability.
In an increasingly data-driven world, blending multiple data streams elevates corporate understanding, offering a fuller image of economic and market trends. The importance of types of data cannot be overstated, as maintaining a responsive and adaptive business strategy is interconnected with the continuous evolution of our digital framework.
The potential for data monetization manifests as organizations realize they sit on valuable datasets accrued over years, now ripe with possibilities. Businesses are looking outward to collaborate and share their data for broader economic benefit while pursuing innovation to stay ahead.
Future advancements hold the promise of introducing entirely new datasets to market analysts and strategists. Areas such as granular material cost data and real-time production condition analytics will likely emerge, delivering further insights into cost dynamics and production efficiencies.
The advantages of data-centered decision-making surpass simple optimization. They extend into sustainable practices, corporate accountability, and enhanced customer satisfaction, underscoring the irreplaceable role of quality data insights in every company's blueprint for achievement.
As the technological landscape continues to accelerate, so too will the demand for smarter, faster, and more precise datasets, inevitably driving a new era of data empowerment and discovery.
Appendix
The wealth of insights generated from data is a boon across various industries, paving the way for more informed decision-making. Roles that can benefit significantly from data insights on input costs and product sensitivity range from investors to consultants, each using this data to approach their specific challenges.
Investors, for instance, depend on holistic datasets to scrutinize companies' cost structures, informing asset allocation decisions and market positions. They leverage trends seen in data to identify potential investment opportunities and risks.${" "}
Consultants wield data-driven insights to advise clients on strategic planning and process optimization, utilizing diverse categories of information to sculpt strategies that offset cost increases or capitalize on valuable input cost trends.
The insurance industry uses such data to assess risk exposure linked with production costs, customizing policies that accurately reflect market volatilities. Their predictive models ensure risk mitigation strategies are aligned with current financial realities.
Market researchers harness datasets to analyze emerging trends in production technologies, supply chain efficiencies, and changing material costs. These findings offer clients a roadmap to navigate complex markets and guide product management plans.
The future promises an even deeper role for AI in unlocking data's latent potential. AI may wield the capability to mine historical documents and modern filings for unprecedented insights, translating raw data into coherent narratives and predictive models that boost decision accuracy.
As these fields grow more attuned to the inherent value residing in data, the potential untapped insights it might offer are boundless. Organizations acquainted with these possibilities stand to not only understand their industries but shape them for a dynamic future.