Leveraging Labeled Image Data for Enhanced Burn Treatment Insights
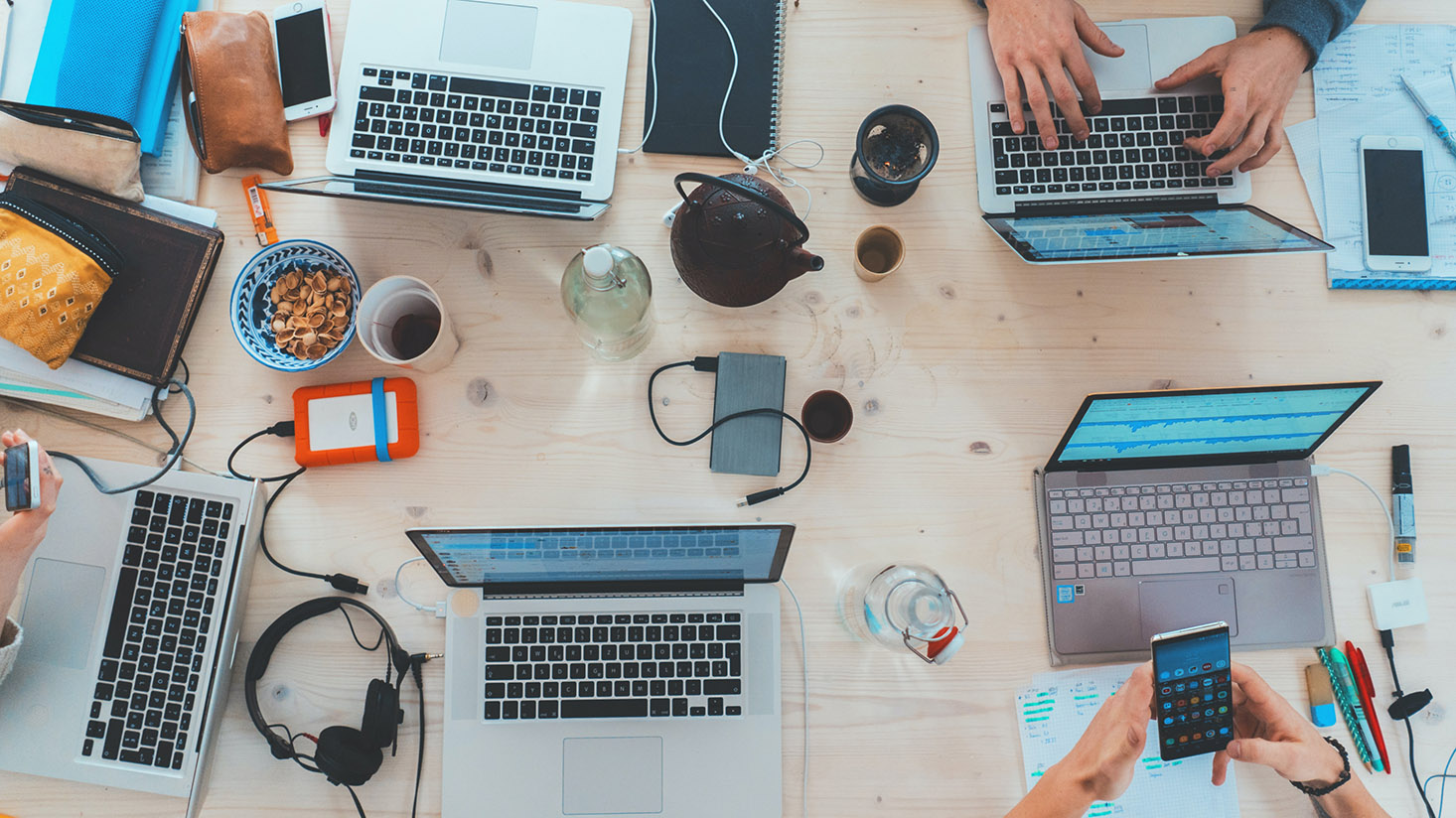
Introduction
The quest to gather actionable insights from labeled images of burn patients has historically been fraught with challenges. In the past, medical professionals had to rely heavily on manual assessments and anecdotal evidence to deduce treatment outcomes. This was an era where data, as we know it today, was either scarce or non-existent. Physicians often relied on word-of-mouth wisdom, which, while invaluable in its own right, was devoid of objective measurement and comprehensive data analysis potential.
Before the proliferation of sophisticated technological tools, data used in burn treatment was limited to basic patient history and rudimentary photographic documentation. It wasn't uncommon for physicians in different geographical areas to have wildly different approaches due to the lack of shared data points or centralized records. This led to delays in understanding the efficacy of treatments, with weeks or even months passing before a conclusive understanding could be reached regarding a particular method.
Enter the digital revolution, bringing sensors, internet connectivity, and a multitude of connected devices to the forefront. The medical field has amply benefited from this technological surge. Software began permeating various layers of healthcare administration and clinical operations, enabling the storage and analysis of vast amounts of data.
The importance of data in modern healthcare, especially in the treatment and analysis of burn wounds, cannot be overstated. Without it, healthcare providers were in a reactive mode, responding to complications after they had arisen instead of proactively preventing them. The introduction of real-time data collection and analysis has transformed this paradigm. Now, interventions can be crafted and fine-tuned based on immediate feedback derived from sophisticated data analysis tools.
Today's healthcare environment is data-rich, enabling professionals to harness real-time monitoring and analytics to refine treatment methods continuously. Immediate access to patient demographics, comorbid conditions, and sequential images of burn wounds across the treatment timeline significantly amplifies decision-making standards and treatment outcomes.
Data's significance in illuminating the pathway to superior patient outcomes is undeniable. Modern datasets not only capture extraordinary levels of detail but also present opportunities for machine learning models to predict outcomes with unprecedented accuracy. These advancements herald a new age where data not only informs but actively guides healthcare practices.
AI Training Data
The category of AI Training Data has emerged as a transformative force in the collection and modeling of medical datasets. AI-powered tools are built to digest vast quantities of image data, extracting patterns and correlations that might elude human observers. The history of AI training data, particularly within healthcare, traces back to initial machine learning algorithms tailored for speech and text recognition. The subsequent advancements allowed AI to leap into image processing, which has been crucial for clinical imaging.
A prime function of AI in the context of burn treatment is the ability to analyze labeled images accurately and rapidly. This not only speeds up diagnosis but also provides insights into the stages of healing and potential complications. Clinics globally, particularly those in resource-limited settings, have turned to AI to bridge the gap where human resources are stretched thin.
Advances in machine learning algorithms and computational power have accelerated the accumulation and processing of labeled image datasets. The healthcare industry, traditionally reliant on expert radiologists and dermatologists, increasingly leans on computer vision to enhance diagnostic precision. As data becomes more accessible, the role of AI becomes increasingly pivotal, simplifying complex datasets across the continuum of care.
The opportunities for utilizing AI training data in burn care are vast. Some examples include:
- Enhanced diagnostic accuracy: AI algorithms trained on labeled image data can discern minute visual differences that may be indicative of infection or poor healing.
- Prognostic modeling: Predictive analytics allow physicians to anticipate healing outcomes based on initial burn assessment data and treatment interventions.
- Customization of treatment plans: AI insights permit tailored interventions based on individual patient data, improving recovery rates.
- Improved clinician training: With access to comprehensive labeled datasets, medical professionals can refine their diagnostic skills based on AI-assisted learning modules.
- Research and development: Researchers can leverage large datasets to identify trends and innovations in burn treatment protocols, leading to novel therapeutic approaches.
Incorporating labeled image data into AI platforms empowers healthcare professionals to generate robust predictive models. These tools are invaluable in developing a nuanced understanding of treatment effects over time, ultimately contributing to improved patient outcomes.
Conclusion
In conclusion, the era of relying solely on subjective clinical evaluations is behind us. The integration of external data strategies, enhanced through AI training sets and comprehensive data analytics, is revolutionizing healthcare.
The accessibility to diverse datasets surrounding burn care, combined with the technological advancements in computational power, enables a deeper comprehension and more accurate treatment pathways than ever before. The culture of data-driven decision-making is swiftly becoming a staple in healthcare institutions worldwide, pressuring slow adapters to modernize their methodologies.
As organizations aim to become more data-focused, they recognize the value of exploring and capitalizing on valuable data assets. This spirit of data monetization finds grounding in the extensive datasets generated during patient care, treatment evaluations, and health records.
In the near future, we can expect emergent data types as organizations continuously extract and derive insights from diverse datasets. Imagine data that granulates patient interactions down to each detailed care episode, or data that predicts treatment outcomes based on genome sequencing.
Burn treatment remains a field of immense transformation potential through data insights. As healthcare professionals increasingly leverage seasoned and evolving datasets, they stand to significantly enhance patient care and operational efficiencies.
Appendix
Various roles and industries stand to benefit greatly from labeled image datasets pertaining to burn treatment. Investors, healthcare consultants, insurance firms, market researchers, and academic institutions represent some of these key stakeholders.
Investors often look for reliable data to foresee burgeoning technologies and potential process improvements in expanding industries. Labelled image datasets serve as a cornerstone for this analysis, highlighting gaps in present methodologies and opportunities for innovation.
Consultants advising healthcare facilities on efficient treatment protocols and enhanced outcomes wherein AI insights pave the way for practical, evidence-based recommendations also greatly benefit from these datasets.
Insurance companies, tasked with the financial oversight and risk assessment of medical procedures, depend heavily on clinical outcomes data. This influences policy limits, premium rates, and reimbursement tactics significantly.
Academic researchers are consistently at the helm of advancing healthcare treatment options. Access to granular image datasets equips them with the detailed information necessary for their studies and simulations.
The potential contributions of AI in processing and predicting from decades-old documents, or mnodern government filings, adds another layer to the utility of historical and current datasets. Future explorations could veer into new segments uncharted today, setting the stage for remarkable breakthroughs in burn treatment and other medical fields.