Leveraging Transaction Data for Insights into Consumer Debt and Delinquency
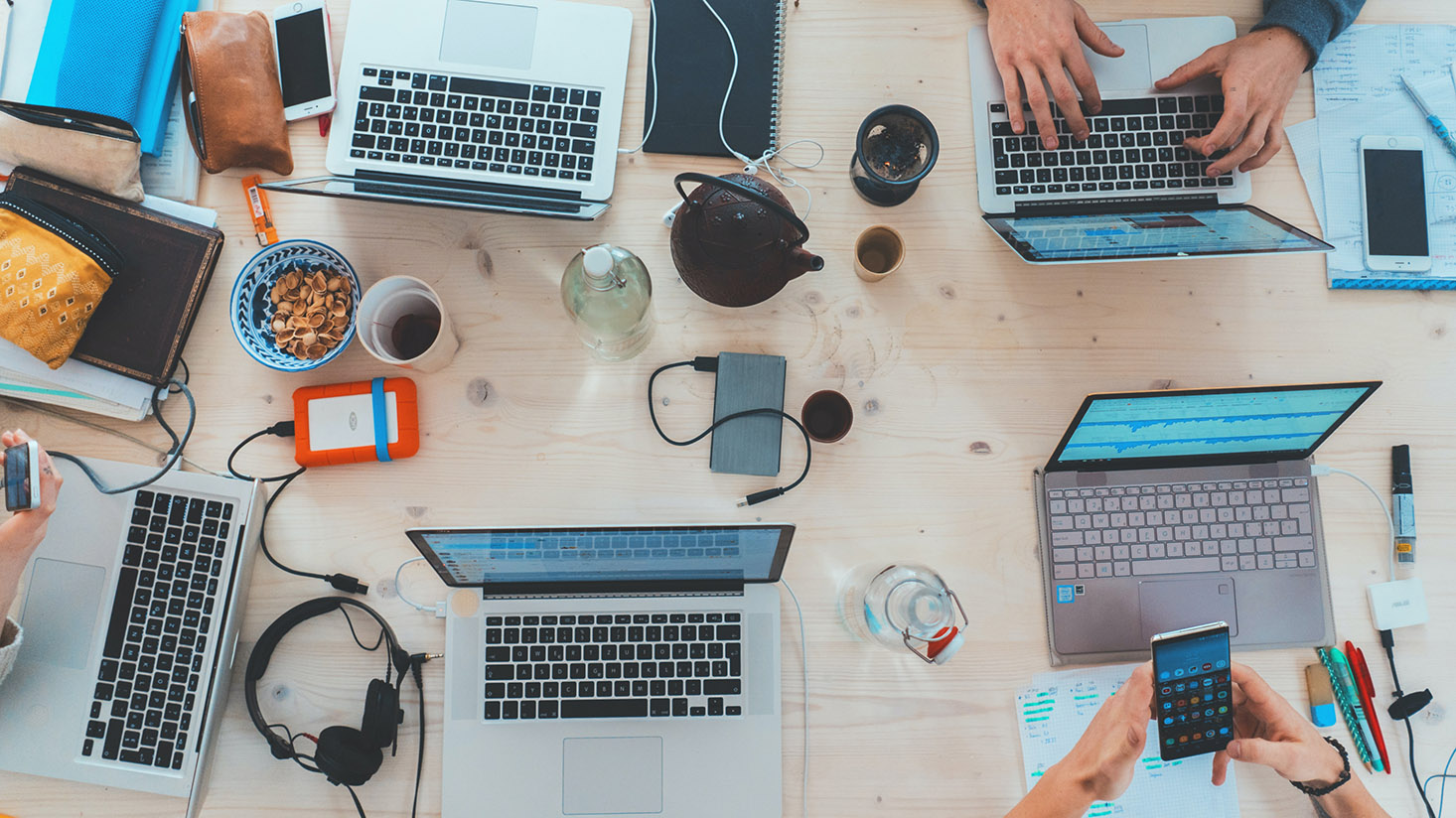
Introduction
Understanding consumer debt and delinquency has been a persistent challenge for financial institutions, policymakers, and businesses alike. Historically, acquiring insights into consumer financial behaviors required arduous and slow processes. Before the digital age, collecting data often meant depending on surveys, self-reported data, or aggregated reports from banks and financial institutions—methods that were often inaccurate and stale by the time they were compiled. In the absence of such data, businesses and analysts relied on anecdotal evidence or focused on macroeconomic indicators such as national debt levels or overall economic health to make informed decisions.
These antiquated methods left stakeholders in the dark for weeks or even months, waiting for official reports from credit bureaus and financial institutions to gauge changes in consumer credit behavior. This lack of timely data often led to missed opportunities, as firms remained unaware of emerging trends until it was too late to respond effectively. Decision-makers were unable to adjust strategies in real-time, often resulting in financial losses and suboptimal resource allocation.
The advent of technology marked a turning point in data collection and reporting. The proliferation of the internet, alongside advanced analytics software, introduced unprecedented levels of connectivity. This shift enabled the storage of vast amounts of information in databases and made it possible for real-time insights into consumer behavior. As companies raced to integrate these technologies, the landscape of consumer debt monitoring began evolving rapidly.
The rise of electronic banking, online transactions, and digital payment methods has revolutionized the way we access and interpret consumer financial data. Through bank transaction data, ACH transactions, and other digital footprints, businesses can now acquire timely insights into customer payment patterns and credit habits. The real-time data capture highlights the number of people paying late, missed payments, and varying debt levels, providing an immediate snapshot of financial health that was previously unavailable.
Today, the availability of massive datasets allows organizations to track consumer debt and delinquent patterns more accurately than ever before. This data, encompassing everything from auto loan payments to mortgage delinquencies, enables a more granular look at financial behaviors. Through data analytics, businesses no longer need to depend solely on past reports but can predict future trends and forecast potential market shifts.
Understanding these behaviors has become more critical than ever. With mounting global concerns on debt management, credit risk, and economic volatility, having insights into consumer debt levels is paramount. With the help of external data, businesses and policymakers can design better financial products, regulatory frameworks, and service delivery models, creating a more stable financial environment.
Transaction Data
One of the most vital data types for gaining insights into consumer debt and delinquency is transaction data. Initially used primarily by banks to track account activity and analyze spending habits, transaction data has evolved in both scope and utility. It encompasses data from various channels, including ACH transactions, credit card payments, and other digital financial activities.
The history of transaction data stretches back to the use of simple ledgers and account books where transactions were recorded manually. As technology advanced and banking systems became digital, transaction data started to be recorded electronically, creating a digital footprint for each account holder. This shift enabled banks and financial institutions to compile comprehensive datasets for internal use, which eventually became available for broader analysis by external entities.
Industries and roles that have historically used transaction data include financial analysts, credit risk managers, and market researchers. Initially, companies used this data to track spending patterns and predict market trends. As the technology matured, businesses began leveraging transaction data to develop personalized financial products, detect fraudulent activities, and improve customer service.
The technological advancement that spurred the scalability of transaction data was the widespread adoption of electronic transactions and digital banking. With millions of transactions occurring worldwide every second, the volume of data continues to grow exponentially. Modern analytics platforms have harnessed this data influx, using AI and machine learning to process, analyze, and derive actionable insights. Furthermore, with categories of data expanding, businesses can now obtain data mentioning detailed aspects of financial behavior such as borrowing tendencies, payment schedules, and even predisposition to delinquency.
The acceleration in data collection and processing capabilities has opened new applications for transaction data. Its relevance has only increased in addressing consumer debt analysis. By scrutinizing transaction patterns and behaviors, financial institutions can detect warning signs of debt distress long before official credit reports are released.
Utilizing Transaction Data for Consumer Debt Insights
- Tracking Payment Delinquencies: By monitoring ACH transactions, analysts can identify late or missed payments promptly. This immediate insight is invaluable for managing credit risk and customer communication strategies.
- Assessing Debt Levels: Analyzing transaction data provides a snapshot of consumer borrowing patterns, including auto loans, mortgages, and other financial obligations, highlighting potential trends in rising or falling debt volumes.
- Understanding Consumer Behavior: A detailed examination of spending habits assists in identifying shifts in financial priorities, helping to assess economic confidence and stability.
- Predicting Market Trends: Early detection of changes in consumer financial behavior can signal unanticipated trends, influencing strategic planning and product development.
- Fraud Detection: Irregular transaction patterns can indicate fraudulent activities or unauthorized access, allowing swift intervention before larger financial implications occur.
With vast datasets available, organizations can now make data-driven decisions that improve financial product designs and enhance customer engagement. As AI and machine learning continue to evolve, their role in processing and interpreting complex data sets will further unveil critical insights into consumer debt and delinquency.
Conclusion
The evolution of data collection and analysis methods provides unprecedented opportunities to understand consumer debt dynamics comprehensively. Access to diverse types of data enables stakeholders to gain insights that were previously unattainable. With ongoing digitization, businesses and policymakers are better equipped to forecast market trends, identify risk factors, and implement more effective financial strategies.
In a world rapidly becoming more data-centric, the ability to interpret and act on consumer financial data is becoming a cornerstone for businesses and governments to remain competitive. This transformation underscores the importance of becoming data-driven organizations and effectively leveraging the data available to serve strategic objectives better.
Moreover, as companies increasingly seek to monetize their data, there is an opportunity for the wider business ecosystem to draw on new data sources to enrich their understanding of consumer debt and financial behavior. This trend signifies a pivot to more nuanced, real-time insights.
Speculating on future data trends, the integration of augmented analytics, blockchain, and decentralized finance offers exciting possibilities for financial analysis. These technologies could lead to the unveiling of entirely new datasets that provide even deeper insights into consumer behavior.
While challenges such as data privacy and ethical considerations remain, organizations that navigate these issues effectively will likely stand at the forefront of financial analytics. Ensuring data is managed responsibly will be crucial to both its utility and acceptance in broader economic contexts.
Ultimately, the future hinges on the continuous evolution of technologies that employ data to transform not only our understanding of the financial landscape but also its very fabric. The potential for innovation in consumer debt monitoring and management is tremendous, driven by an ever-expanding universe of data.
Appendix
The data landscape offers tremendous opportunities across various roles and industries. For instance, financial analysts and credit risk managers can use consumer debt data to pinpoint areas of risk and adjust their strategies accordingly. Investors benefit significantly from understanding debt patterns, using insights to guide investment decisions and portfolio management.
Consultants and market researchers find data invaluable for advising clients on product development and market entry strategies. By harnessing early warning indicators of financial distress, they can proactively advise businesses on strategic pivots, gaining a competitive edge in adapting to market shifts.
Insurance companies also stand to benefit considerably from insights into consumer debt trends. Understanding which segments of the population are financially strained can help tailor insurance products and assess risk more accurately, leading to improved underwriting processes.
As the future unfolds, tapping into AI technologies offers exciting possibilities for unlocking latent value in existing datasets. As algorithms grow more advanced, their capacity to decode tangled data sets and extract actionable insights holds the promise of substantial industry transformation.
With advancements in training data methodologies, future breakthroughs could involve new algorithms that automatically detect consumer stress levels based on holistic spending analyses or identify demographic cohorts likely to face financial difficulties before they are recognized in traditional reporting metrics.
Overall, the continued integration of innovative data practices will redefine how businesses and industries perceive and act upon consumer behavior trends, fostering more resilient financial systems. The evolving nature of data and analytics will undoubtedly pave the way for substantial advancements in consumer debt understanding and management.