Loan Approval Trends Data
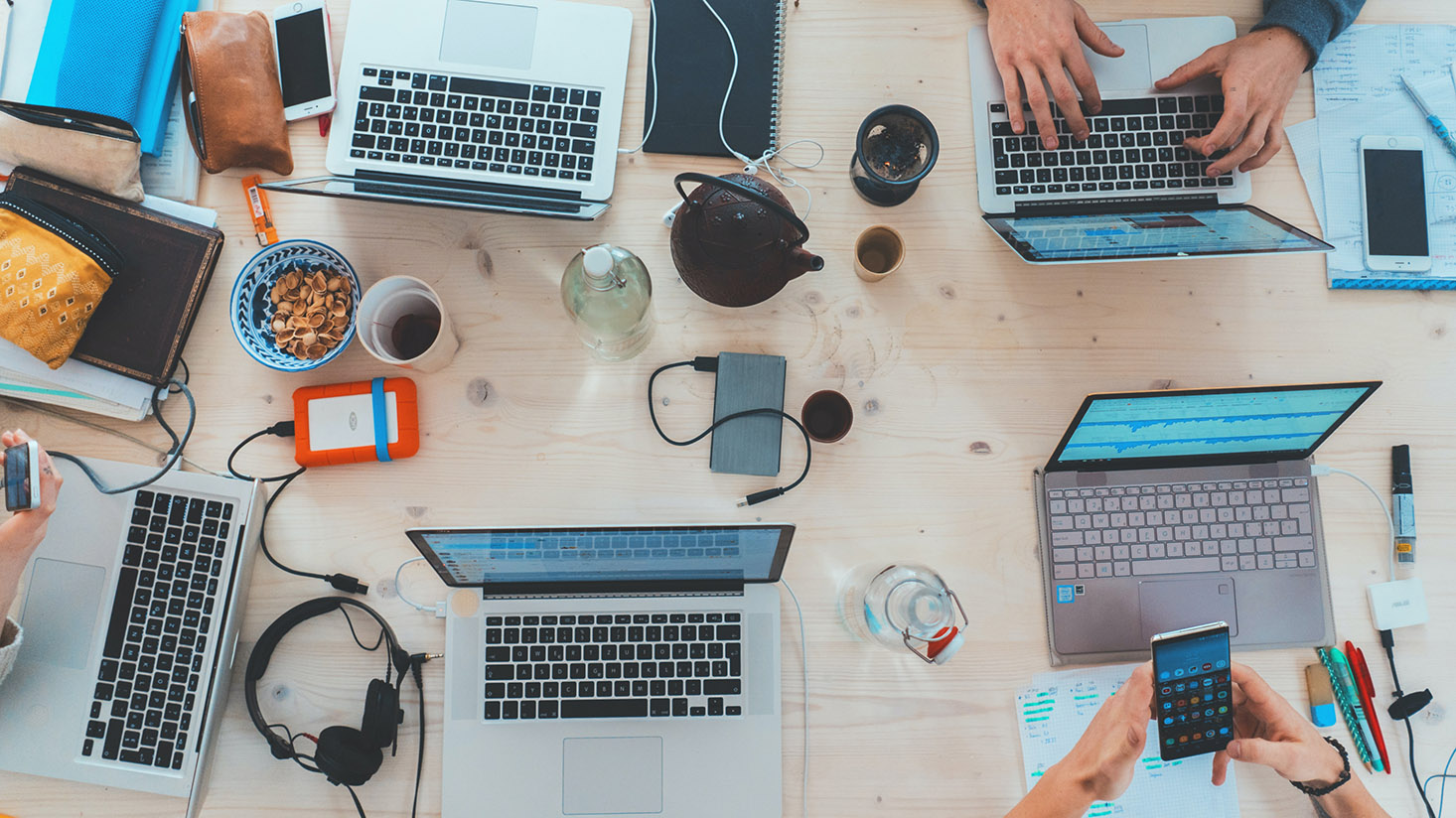
Introduction
Understanding the dynamics of loan approval and rejection rates has always been a critical aspect of financial analysis and risk management. Historically, gaining insights into these trends was a challenging endeavor. Before the digital age, financial institutions and analysts relied on manual record-keeping and anecdotal evidence to gauge the health of loan portfolios and predict future trends. This often meant sifting through mountains of paper applications, decision letters, and financial statements. Before any structured data collection, insights were based on personal experience and market hearsay, making it difficult to draw accurate or timely conclusions.
The advent of sensors, the internet, and connected devices, alongside the proliferation of software into many financial processes, has revolutionized how data on loan approval and rejection rates is collected and analyzed. The move towards digital applications and decision-making processes has meant that every application, approval, rejection, and the rationale behind each decision is now stored in databases, making real-time analysis possible. This shift has not only improved the accuracy of insights but has also significantly reduced the time it takes to understand changes in loan approval trends.
The importance of data in understanding loan approval and rejection rates cannot be overstated. In the past, financial analysts and institutions were in the dark, waiting weeks or months to understand changes in the lending landscape. Now, with access to real-time data, they can understand changes as they happen, allowing for more informed decision-making and risk management.
One of the key advancements in this area has been the development of consumer behavior data platforms. These platforms aggregate vast amounts of loan origination data, including critical factors such as credit scores, income levels, geographical location, and the existence of other loans. This data provides a comprehensive view of consumer credit behavior, which is essential for analyzing loan approval and rejection trends.
Consumer Behavior Data
History and Evolution
The collection and analysis of consumer behavior data have evolved significantly over the years. Initially, financial institutions relied on basic demographic information and simple financial metrics to make lending decisions. However, the technology advances in data collection and analytics have enabled a more nuanced understanding of consumer behavior. The introduction of credit scoring models in the mid-20th century was a significant milestone, providing a standardized way to assess credit risk. Over time, the amount of data collected has expanded to include more detailed consumer credit identifiers, such as income, geographical location, and the existence of other loans.
Today, the amount of consumer behavior data available is accelerating at an unprecedented rate. This is largely due to the digitalization of financial services and the increased use of online platforms for loan applications. Financial institutions and data providers now have access to a wealth of information that can be used to analyze trends in loan approval and rejection rates in real-time.
Types of Data and Usage
Consumer behavior data encompasses a wide range of information, including:
- Loan origination data: Information on approved loans, broken down by credit score, loan type (e.g., HE, HELOC, personal loans), and other credit identifiers.
- Credit score data: Detailed breakdowns of applicants' credit scores, providing insights into the creditworthiness of potential borrowers.
- Income and geographical data: Information on applicants' income levels and geographical location, which can influence loan approval rates.
- Existence of other loans: Data on applicants' existing loans, both past and present, which can impact their ability to secure new loans.
This data can be used to gain insights into consumer credit behavior, identify trends in loan approval and rejection rates, and make more informed lending decisions. For example, analyzing loan origination data by credit score can help financial institutions understand the risk profile of their loan portfolio and adjust their lending criteria accordingly.
Conclusion
The importance of data in understanding loan approval and rejection rates cannot be overstated. With access to detailed consumer behavior data, financial institutions and analysts can gain real-time insights into lending trends, enabling more informed decision-making and risk management. The evolution of data collection and analysis technologies has transformed the lending landscape, making it possible to analyze trends in loan approval and rejection rates with unprecedented accuracy and speed.
As organizations become more data-driven, the ability to discover and analyze relevant data will be critical to their success. The monetization of useful data that has been collected for decades is a growing trend, and the field of loan approval and rejection rates is no exception. In the future, we can expect to see the development of new types of data that can provide additional insights into consumer credit behavior and lending trends.
Appendix
The analysis of loan approval and rejection rates is relevant to a wide range of roles and industries, including investors, consultants, insurance companies, and market researchers. These professionals rely on accurate and timely data to make informed decisions and assess risk. The transformation of the lending landscape through data has enabled these professionals to gain deeper insights into consumer behavior and lending trends, improving their ability to predict future movements in the market.
Looking ahead, the potential for AI to unlock the value hidden in decades-old documents or modern government filings is immense. As technology continues to evolve, the ability to analyze vast datasets and extract meaningful insights will become increasingly important, driving further innovation in the field of loan approval and rejection rates.