Maximizing M&A Success with Financial and NLP Data Insights
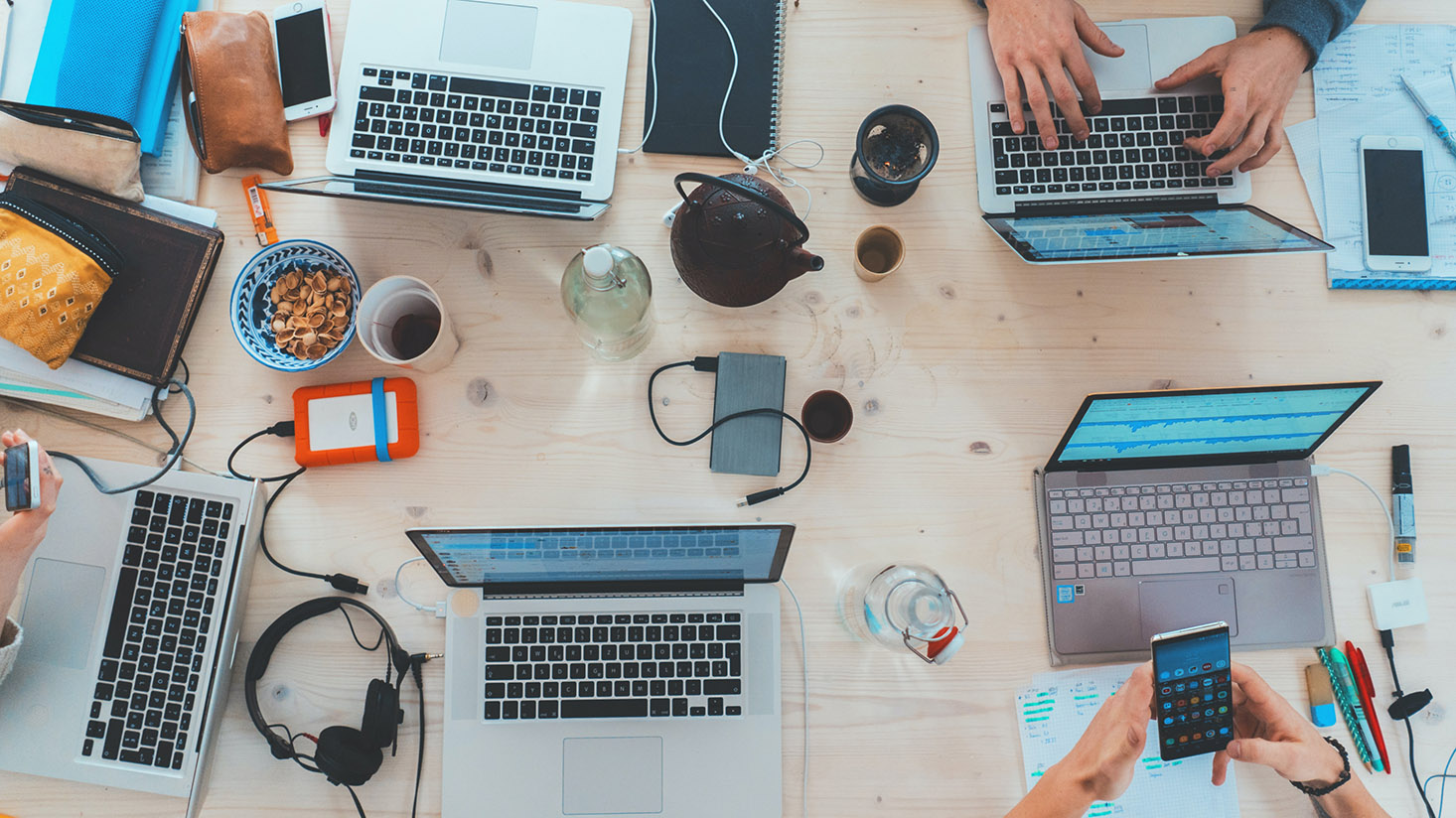
Introduction
The world of mergers and acquisitions (M&A) has always been a complex arena, veiled in ambiguity and uncertainty. Historically, gaining insights into M&A transactions required a mix of intuition, experience, and a painstaking slog through disparate data sources and manual processes. Before the digital age sparked the rise of data-driven decision-making, professionals relied heavily on network-driven intelligence, anecdotal evidence, and time-consuming research to gather information on potential deals. It wasn’t unheard of for decision-makers to act on gut feel, banking on their years of industry experience, crossed fingers, and a bit of trust in luck.
Early attempts at gathering M&A data involved studying public company announcements, printed financial reports, and laborious financial reconciliations, often leading to weeks or months of delay in understanding market trends. The intensive, manual labor required made the analysis prone to inconsistencies and errors. With the introduction of external data, companies started to keep meticulous records of transactions, providing a more tangible and structured glimpse into the market. However, merging these multifaceted datasets into holistic insights remained challenging.
The advent of the digital age, heralded by the proliferation of sophisticated communication networks, sensors, and databases, marked a turning point. With every click and transaction digitized, the instant availability of data opened new avenues for understanding the nuances of market fluctuations. This shift to digitization has redefined how M&A data is perceived, analyzed, and utilized today. Technologies like artificial intelligence, machine learning, and natural language processing (NLP) have further accelerated this transformation, enabling unprecedented real-time insights and deep analytical capabilities.
Data’s role in transforming our understanding of M&A cannot be overstated. Instead of waiting interminable periods to react post-facto, decision-makers can now adjust their strategies in real-time to better align with market conditions. Today’s business landscape mandates a proactive approach to data-driven strategy, and no realm benefits as directly from data insights as M&A.
As we usher into an era dominated by data, organizations are realizing the irrefutable power of a comprehensive understanding of transactional dynamics. The data-driven landscape not only democratizes insights but also provides a competitive edge to organizations that leverage the right kinds of data. Hence, the quest for a richer, alternative source of M&A transaction data becomes imperative.
This article explores how leveraging distinct categories of data can significantly enhance insights within the M&A domain, casting new light on historical patterns and paving the way for strategic innovation.
NLP Data
The application of Natural Language Processing (NLP) in the realm of M&A data has dramatically reshaped how information is processed and utilized. Originally developed to give computers the ability to understand, interpret, and respond to human language, NLP has become a robust tool to glean insights from large volumes of unstructured text data.
Consider the time when M&A analysts would sift through endless press releases, financial disclosures, and annual reports to infer the potential driving forces behind a market move. These texts, rich with financial jargon and context, often held valuable insights that needed precise extraction and interpretation. Historically, deciphering these insights was both an art and a science, demanding a human’s innate ability to parse nuance from dense language.
NLP has revolutionized this task by automating the analysis of vast arrays of textual data, thereby imbuing speed and accuracy into the analytical process. Today, machines can recognize and interpret complex patterns and sentiments in news articles or financial commentary that might point to merger interest, competitive pressures, or evolving market conditions. NLP enables the systematic quantification of qualitative data, making it an invaluable asset in decision-making.
The surge in available text data has been meteoric, with news sites, financial blogs, and social media channels contributing to the ever-growing dataset. NLP techniques, such as sentiment analysis and topic modeling, are now employed to obtain insights that aid in anticipating market trends and identifying potential acquisition targets.
By harnessing NLP data, analysts can:
- Identify emerging trends by monitoring sentiment shifts in industry news and reports.
- Automatically tag and categorize transactions for streamlined analysis.
- Provide real-time alerts on significant developments that could impact deal dynamics.
- Enhance due diligence processes with comprehensive sentiment and risk analysis.
- Refine predictive models by drawing from past transaction reports and estimating future M&A activity.
Financial Data
At the core of M&A analysis lies financial data, integral to evaluating transactions, measuring company performance, and forecasting future market conditions. Long before data democratization, financial insights were predominantly reserved for those with access to high-level proprietary databases or detailed public documentation.
Traditional M&A analysts routinely engaged in a tedious cycle of revisiting printed balance sheets, earnings reports, and industry analyses to ascertain market direction. Budget committees and boards of directors leaned on historical financials, awaiting quarterly or annual updates to make strategic decisions, which frequently relegated companies to playing catch-up in rapidly evolving industries.
The evolution of digital databases has transformed the availability and depth of financial data, providing a holistic view of financial performance metrics across public and private companies. Data providers amalgamate vast swathes of financial data, offering granular details on mergers, acquisitions, spin-offs, and divestitures globally. From Bloomberg to emerging fintech platforms, the richness and accessibility of financial data have set a new standard.
Some technological advancements that facilitated exponential growth in financial data include API integrations and cloud-based data solutions. Today, organizations leverage these technological innovations to harness financial data with ease and agility.
Financial data helps businesses tackle M&A with precision by facilitating:
- Comprehensive analysis of deal terms to understand the underlying strategic rationale.
- Assessment of asset performance and risk factors essential to due diligence.
- Valuation modeling to predict future operational performance and investment potential.
- Benchmarking through historical deal comparisons to align with industry standards.
- Scenario analysis to evaluate potential outcomes and contingencies.
Conclusion
As elucidated, the broad tapestry of types of data available today has fundamentally changed how businesses engage with M&A transactions. From the intricate narratives drawn by NLP data to the empirical assessments generated by financial data, the fusion of these dynamic sources unearths unprecedented insights for business leaders and deal-makers.
The importance of becoming a truly data-driven organization is profoundly illustrated in the realm of M&As, where time-sensitive opportunities often delineate market leaders from followers. Embracing this evolution, companies are increasingly realizing the potential of monetizing their data, unlocking value streams that were once latent.
Looking forward, as data streams continue to evolve and expand, corporations must remain agile, adapting swiftly to incorporate emerging datasets into their workflows. This data-driven capability will be critical as organizations seek not just to understand the market today but to predict what it will be tomorrow.
The quest for deeper insights will propel innovation in the kinds of data sold and mined. Imagining data streams like comprehensive AI-driven predictive models or instantaneous global market feedback seems no longer far-fetched but inevitable. Companies that embrace data as their compass will be better poised to navigate the unpredictable, charting paths to success.
The continued fusion of data categories indicates an exciting frontier where strategic foresight is shaped at the junction of technology and data.
Appendix
The transformative power of data is being felt across various sectors, with M&A information serving as a pivot for industries eager to harness data-driven capabilities. These sectors are witnessing a profound metamorphosis, thanks to the availability and insights of novel data streams. Roles and industries for whom this transformation holds significance include:
Investors and Financial Analysts: Within the financial sphere, investors and analysts depend on data to generate fortune-altering insights about companies of interest. Investors leverage financial and textual data to inform their decisions, aiming to maximize returns on their investments through strategic acquisitions or mergers.
Consultants and Strategists: Consultancies involved in advising on M&A strategy find the integration of data instrumental in tailoring bespoke and viable strategies for their clients. By leveraging data to inform their consults, they optimize client offerings, driving transformational change.
Insurance Sectors: Insurers benefit by utilizing these categories of data to assess the risk profiles of newly merged entities, fine-tuning their risk assessments and premium calculations with greater confidence.
Market Researchers: For market research firms, vast data streams translate to deeper, more precise insights into industry landscapes. Analysts examine how mergers shift market dynamics, informing client recommendations and reinforcing service value.
The ultimate future of M&A intelligence aligns with technological trends exploring the possibilities of AI, which will continue to unlock predictive prowess, turning data’s ubiquitous nature into actionable foresight for a world replete with decision-makers eager to stay ahead.