Retail Demand Forecasting Data
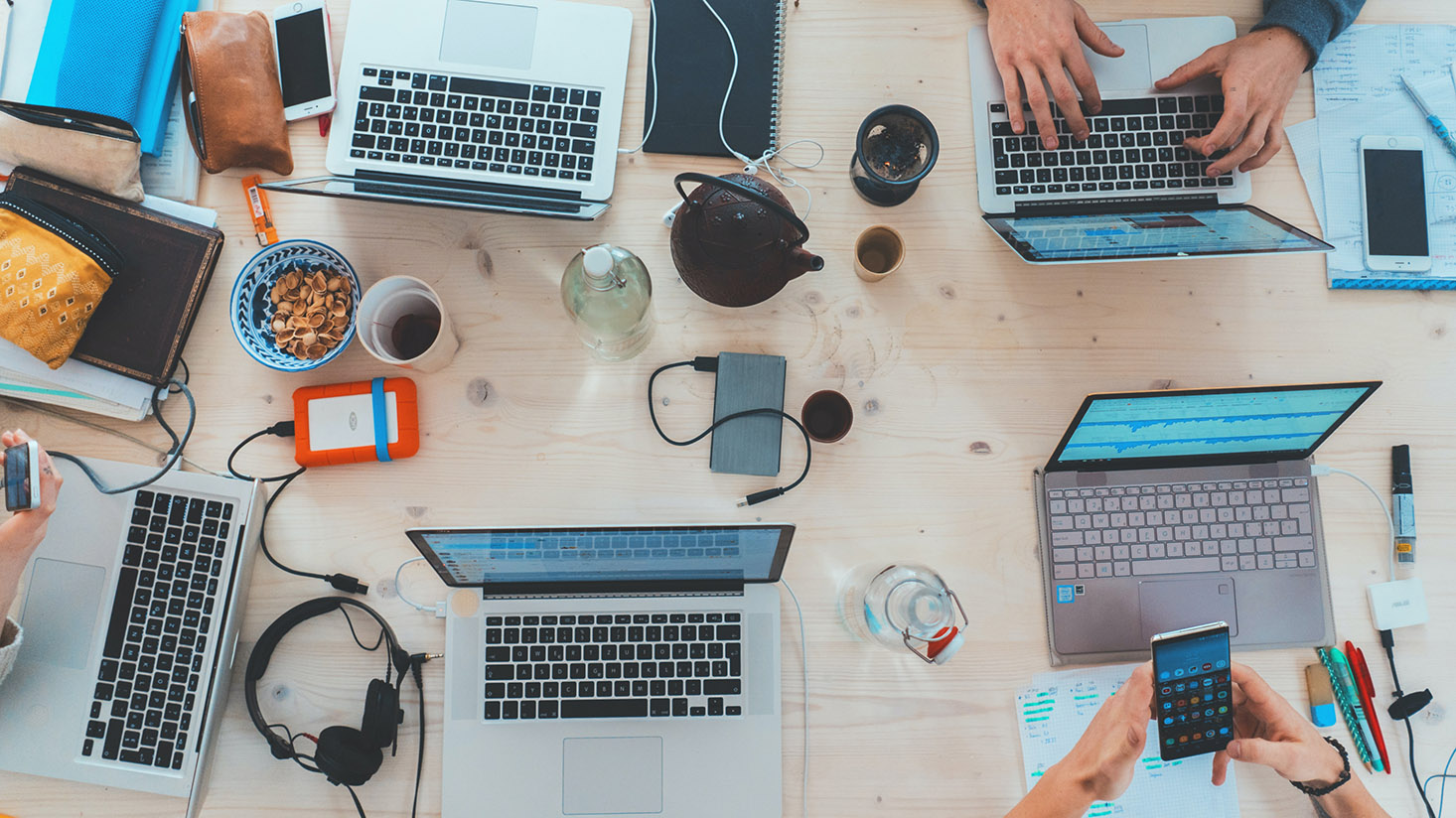
Introduction
Understanding and predicting retail demand has always been a cornerstone of successful business management. Historically, firms relied on rudimentary methods to gauge consumer interest and forecast demand. Before the digital revolution, businesses often had to wait for sales reports, customer feedback, and market trends to understand their performance, leading to delayed responses to market changes. Traditional data sources included manual customer surveys, sales data, and sometimes, educated guesses based on past experiences. Before the advent of sophisticated data collection methods, businesses were essentially navigating in the dark, making decisions based on limited and often outdated information.
The introduction of sensors, the internet, and connected devices has revolutionized the way businesses collect and analyze data. The proliferation of software and the digital storage of events have made it possible to track consumer behavior in real-time. This technological advancement has provided businesses with the ability to understand changes as they happen, significantly reducing the time between data collection and decision-making.
Data has become an invaluable asset in understanding retail demand. With the right datasets, businesses can now predict trends, understand consumer behavior, and make informed decisions swiftly. This shift from traditional methods to data-driven strategies has transformed the landscape of retail management, offering a competitive edge to those who effectively leverage data.
The importance of data in understanding retail demand cannot be overstated. In the past, businesses were often left guessing about consumer preferences and market trends. Now, with access to real-time data, companies can anticipate changes, adapt strategies, and meet consumer needs more effectively. This transition to a data-driven approach has not only improved decision-making but also enhanced the overall customer experience.
For businesses looking to understand and predict retail demand, especially for specific services like Stitch Fix, accessing the right types of data is crucial. This article will explore how various datasets can provide insights into retail demand forecasting, focusing on the importance of mobile app data and transaction data in predicting trends and understanding consumer behavior.
As we delve into the specifics of these data types, it's important to remember that the goal is not just to collect data but to derive actionable insights that can drive business success. The ability to accurately forecast retail demand can significantly impact a company's bottom line, making the investment in quality data an essential part of modern business strategy.
Mobile App Data
Mobile app data has emerged as a powerful tool in understanding and predicting retail demand. With millions of apps available across various platforms, tracking the performance of specific apps can provide valuable insights into consumer behavior and preferences. For businesses like Stitch Fix, which rely heavily on their mobile presence, analyzing app data can be particularly beneficial.
Key data points such as downloads, in-app purchase revenue, daily active users, monthly active users, total app sessions, and total time spent in the app offer a comprehensive view of app performance. This data, broken down by country or region, can reveal trends and patterns that are instrumental in forecasting demand.
The technology behind mobile app data collection has evolved significantly, allowing for real-time analysis with minimal lag. This immediacy means businesses can quickly adapt to changes in consumer behavior, optimizing their offerings to meet current demands. The historical data collected through apps also provides a rich source for predictive analytics, enabling companies to anticipate future trends.
Industries and roles that benefit from mobile app data include market researchers, product managers, and financial analysts. These professionals use app performance metrics to gauge consumer interest, track competitive performance, and make informed decisions about product development and marketing strategies.
The amount of data generated by mobile apps is accelerating, offering deeper insights into consumer behavior. As technology advances, the potential for mobile app data to inform business decisions continues to grow. For companies looking to understand and predict retail demand, leveraging mobile app data can provide a significant advantage.
Examples of how mobile app data can be used include:
- Tracking user growth: Analyzing the increase in monthly active users can indicate rising interest in a service.
- Understanding consumer engagement: Total time spent in the app and total app sessions can reveal how engaged users are with the service.
- Forecasting sales: In-app purchase revenue can serve as a leading indicator of financial performance.
Transaction Data
Transaction data provides a direct window into consumer spending behavior, offering insights that are critical for forecasting retail demand. This type of data encompasses a wide range of financial activities, including credit card, debit card, and bank transactions. For businesses aiming to predict demand trends, transaction data can reveal valuable information about consumer spending patterns, transaction volumes, and user growth.
The collection and analysis of transaction data have been revolutionized by advancements in fintech and data analytics. With near-real-time access to transaction information, businesses can now track consumer spending trends with unprecedented accuracy. This immediacy allows for agile decision-making, enabling companies to respond swiftly to shifts in consumer behavior.
Transaction data is particularly useful for understanding the financial health and consumer interest in specific services. By analyzing transaction counts, average ticket size, and spend per customer, businesses can gain insights into consumer preferences and spending habits. This data, often segmented by geographic and income demographics, can inform targeted marketing strategies and product offerings.
Industries that benefit from transaction data include retail, financial services, and e-commerce. Roles such as financial analysts, marketing professionals, and business strategists use this data to inform their decisions, driving revenue growth and enhancing customer satisfaction.
The volume of transaction data is growing, fueled by the increasing digitization of financial activities. This growth presents an opportunity for businesses to harness the power of transaction data to better understand and predict retail demand. As technology continues to evolve, the potential for transaction data to impact business strategy expands, making it an essential tool for companies looking to stay ahead in the competitive retail landscape.
Examples of how transaction data can be used include:
- Identifying spending trends: Tracking consumer spending trends can help businesses anticipate demand for specific products or services.
- Understanding customer demographics: Analyzing transaction data by geographic and income demographics can inform targeted marketing efforts.
- Forecasting sales: Transaction volumes and average ticket size can serve as indicators of financial performance and consumer interest.
Conclusion
The importance of data in understanding and predicting retail demand cannot be overstated. In today's fast-paced business environment, having access to the right types of data can provide businesses with the insights needed to make informed decisions. Mobile app data and transaction data are just two examples of the datasets that can offer valuable insights into consumer behavior and retail demand forecasting.
As organizations become more data-driven, the ability to effectively leverage data will be critical to success. Data discovery and analysis will play a key role in informing business strategies, enabling companies to adapt to changing market conditions and meet consumer needs. The potential for data to transform business operations is immense, and companies that invest in quality data will be well-positioned to thrive in the competitive retail landscape.
The future of data in retail demand forecasting is promising. With advancements in technology, new types of data will emerge, offering even deeper insights into consumer behavior and market trends. Companies that are proactive in exploring and integrating these new data sources will gain a competitive edge, driving innovation and growth.
Finally, the monetization of data presents an exciting opportunity for businesses. As companies recognize the value of the data they generate, the potential for data to become a revenue stream in its own right will increase. This shift towards data monetization will not only benefit individual companies but also contribute to the overall growth of the data economy, providing new opportunities for insights and innovation in retail demand forecasting.
Appendix
Industries and roles that could benefit from data on retail demand forecasting include investors, consultants, insurance companies, market researchers, and financial analysts. These professionals face challenges in understanding market trends, consumer behavior, and financial performance. Data has transformed these industries by providing insights that inform strategic decisions, enhance customer experiences, and drive revenue growth.
The future of data in these industries is bright, with AI and machine learning poised to unlock the value hidden in decades-old documents and modern government filings. As technology continues to advance, the potential for data to revolutionize industry practices and drive innovation is limitless. Companies that embrace data-driven strategies will be well-equipped to navigate the complexities of the modern business landscape, achieving success in an increasingly competitive environment.