Supply Chain Logistics Insights Using Geolocation and Transportation Data
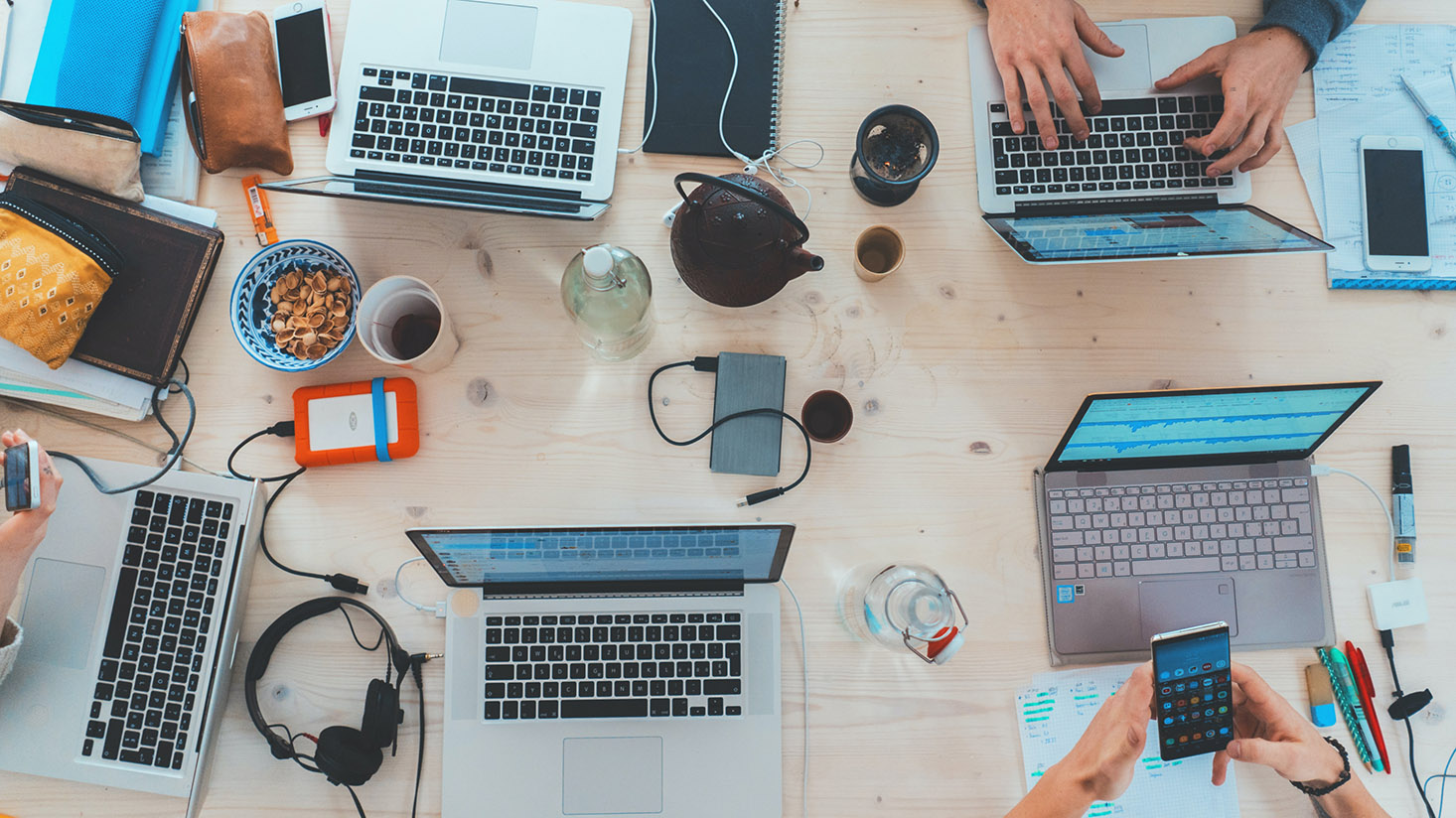
Introduction
The dynamic world of supply chain logistics has often seemed like a tangled web of intricate challenges. Traditionally, gaining insights into this complex topic was much akin to navigating through a labyrinth without a map. In days of yore, businesses were reliant on primitive methods and lacked the precise data and technology needed to demystify supply chain logistics, particularly in the realm of trucking and freight data for big-box retailers. Companies relied heavily on anecdotal evidence and rudimentary record-keeping that could only offer a fraction of the story.
Some of the older types of data that companies would rely upon included basic inventory records, manual count logs, and sporadic freight delivery receipts, which were often outdated by the time they were collected. Before the emergence of digitized data or even any data at all, businesses were largely in the dark, waiting weeks or sometimes months to uncover seasonal trends or supply issues. Decisions were more of a guessing game without the clarity and confidence that timely data provides.
Fortunately, with the advent of connected devices, the Internet, and the rise of comprehensive data storage solutions, the landscape has transformed dramatically. Sensors and data integration technologies have revolutionized the way supply chain logistics are managed, especially within the grocery and home goods categories of major retailers like Walmart, Target, and Home Depot.
These technologies have not just made data collection easier, but have redefined the very notion of logistics management. Businesses now benefit from real-time data tracks that illuminate trucking supply volumes and enable assessment of tenant health based on resupply activities. The paradigm shift from quarterly reports to real-time data has truly been groundbreaking.
The importance of data in understanding supply chain logistics cannot be overstated. Unlike in the past, companies no longer have to operate under a veil of uncertainty. They can actively gauge changes in supply and demand, emerging seasonal trends, and the complex timings of goods as they flow through the supply chain. This has immensely empowered decision-makers, leading to smarter and more efficient business processes.
Today, leveraging rich datasets in real time provides a strategic edge, guiding business professionals in making well-informed decisions that were once unimaginable. The reliance on outdated models has given way to a data-driven culture where insights are derived from solid numerical evidence, paving the road for more innovative and efficient logistics solutions.
Automotive Data
The shift towards a digital-first approach in supply chain logistics is partly heralded by the evolution of automotive data. This marks a radical departure from when rudimentary methods were used to track transportation metrics. Historical paths were filled with trial and error as there was scant means to capture and analyze such data comprehensively.
In contemporary practice, automotive data has found a significant foothold, especially when dealing with freight delivery and trucking routes. Today, sophisticated datasets organize trillions of data points from both private and commercial vehicles. This provides insights that are invaluable for reducing congestion and improving traffic flow—a crucial factor when dissecting logistics challenges.
Industries that have historically depended on automotive data include transportation agencies, logistics enterprises, and urban planners. The early technological advances that contributed to the current landscape included GPS-enabled tracking and advanced vehicle telematics, which opened up novel ways to collect and analyze data.
The amount of automotive data captured continues to accelerate, driven by advances in sensor technology and connectivity. This is particularly important for major retailers who seek insights into trucking routes and fleet management. By understanding these logistics, businesses can better predict and manage supply chain efficiencies.
Specific Uses of Automotive Data in Understanding Supply Chain Logistics:
- Fleet Tracking & Optimization: Utilize real-time data to monitor and redirect fleets based on traffic conditions.
- Fuel Efficiency: Access data on fuel consumption trends to optimize transportation costs.
- Predictive Maintenance: Use vehicle data to preemptively address potential mechanical failures, minimizing disruptions.
- Route Planning: Analyze historical and real-time data to devise the most efficient delivery routes.
- Customer Satisfaction: Improve delivery estimates by monitoring real-time vehicle locations and traffic conditions.
Ultimately, automotive data provides an expansive understanding of transportation logistics, offering a vision that can preempt bottlenecks and inefficiencies, thereby enhancing the overall supply chain process for major retailers.
Geolocation Data
Another cornerstone in the mosaic of supply chain logistics is geolocation data. Historically, businesses had limited visibility into the exact locations and movements of their freight operations. The narrative has changed dramatically with geolocation technology, which paints a vivid picture of supply routes and terminal points.
Geolocation data has been utilized in a variety of fields beyond logistics, including marketing, environmental science, and emergency response. Its core strength lies in tracking movement patterns and mapping connections between locations.
Technological advancements such as enhanced GPS systems, cellular triangulation, and satellite mapping have all contributed to the enriched quality of today's geolocation data. Businesses can now access an unprecedented range of information on mobility patterns, resulting in a comprehensive understanding of the entire supply chain's workability.
This type of data is not just expansive but also highly granular, providing invaluable insights into daily, hourly, and even quarter-hour traffic patterns around supply zones and shopping malls. The sheer richness of the data offers opportunities to analyze real-time shifts in supply volumes and seasonal trends more adeptly than ever before.
Specific Uses of Geolocation Data in Understanding Supply Chain Logistics:
- Trend Analysis: Analyze historical and real-time truck trips for logistical insights.
- Traffic Management: Use traffic and congestion data to optimize supply routes.
- Market Insights: Understand mobility patterns in relation to retail trends and tenant health.
- Demand Forecasting: Leverage geolocation data to predict shifts in customer demand based on supply chain movements.
- Resource Allocation: Determine areas where resources and focus should be allocated, based on detailed location data.
By understanding logistical flows and traffic trends, geolocation data serves as a vital tool that enhances the decision-making process, making the journey from supplier to retailer smoother and more efficient.
Conclusion
In summary, it is clear that the modern landscape of supply chain logistics has been significantly redefined by the profound role of data. Gaining insights into transportation volumes, seasonal supply shifts, and tenant health for big-box retailers has become less of an enigma and more of a calculated science. By harnessing these diverse data types, firms are empowered to make better, informed decisions that can streamline and optimize their supply chains.
With access to various predictive datasets, businesses within logistics can narrow their focus on what truly matters. Companies can preemptively solve problems previously difficult to navigate due to archaic record-keeping methods, which often lacked precision or timeliness.
Yet, for firms to stay ahead of the curve, they must continually embrace a data-driven culture. The value of discovery in this domain extends beyond mere operational efficiency and builds toward sustainable, long-term growth. This includes the integration of AI and machine learning algorithms that can transform raw data into actionable insights.
Moreover, organizations are actively seeking to monetize their data. Companies that have accumulated vast databases for decades are awakening to the potential goldmine they possess. This trend is not exempt from supply chain logistics, where data richness presents opportunities for both internal optimization and external value creation.
Looking ahead, we can anticipate new data sources that might become crucial as industries aspire to unravel even more accurate and specific logistics insights. Innovations will emerge with smarter ways to measure and predict logistics demands, leading to newfound efficiencies and practices.
Appendix
Supply chain logistics data find wide-ranging applicability across several industries, redefining roles and transforming long-standing challenges into manageable opportunities. Initially, investors can leverage these insights to pinpoint operational efficiency and assess retailer health—a prudent approach to capital allocation and risk management.
Consultants and analysts also stand to benefit significantly, offering expert advice and guidance backed by concrete data. By assimilating insights from trucking and logistics datasets, they provide actionable recommendations for enhancing supply chain performance and aligning these with strategic business goals.
Insurance companies, too, have a vested interest in logistics data, which can drastically affect risk assessments and policy evaluations. Geolocation and automotive data provide details essential for accurately measuring vehicle fleet risks and establishing sound underwriting practices.
Market researchers who focus on consumer behavior and retail trends are also in line to harness these insights. By understanding the ebb and flow of delivery patterns and changes in supply volumes, researchers can accurately assess retail health services and the broader shopping ecosystem.
The future holds even more promise as AI technologies make strides in unlocking hidden values buried in old datasets and modern government filings. From predicting logistics movements to assessing transportation turnovers, the possibilities with AI are vast.
In sum, supply chain logistics data not only reshapes traditional industry roles but also paves the way for limitless opportunities in refining strategies and practices across a global scale.