Transform Financial Risk Assessments with Advanced Fraud Loss Insights
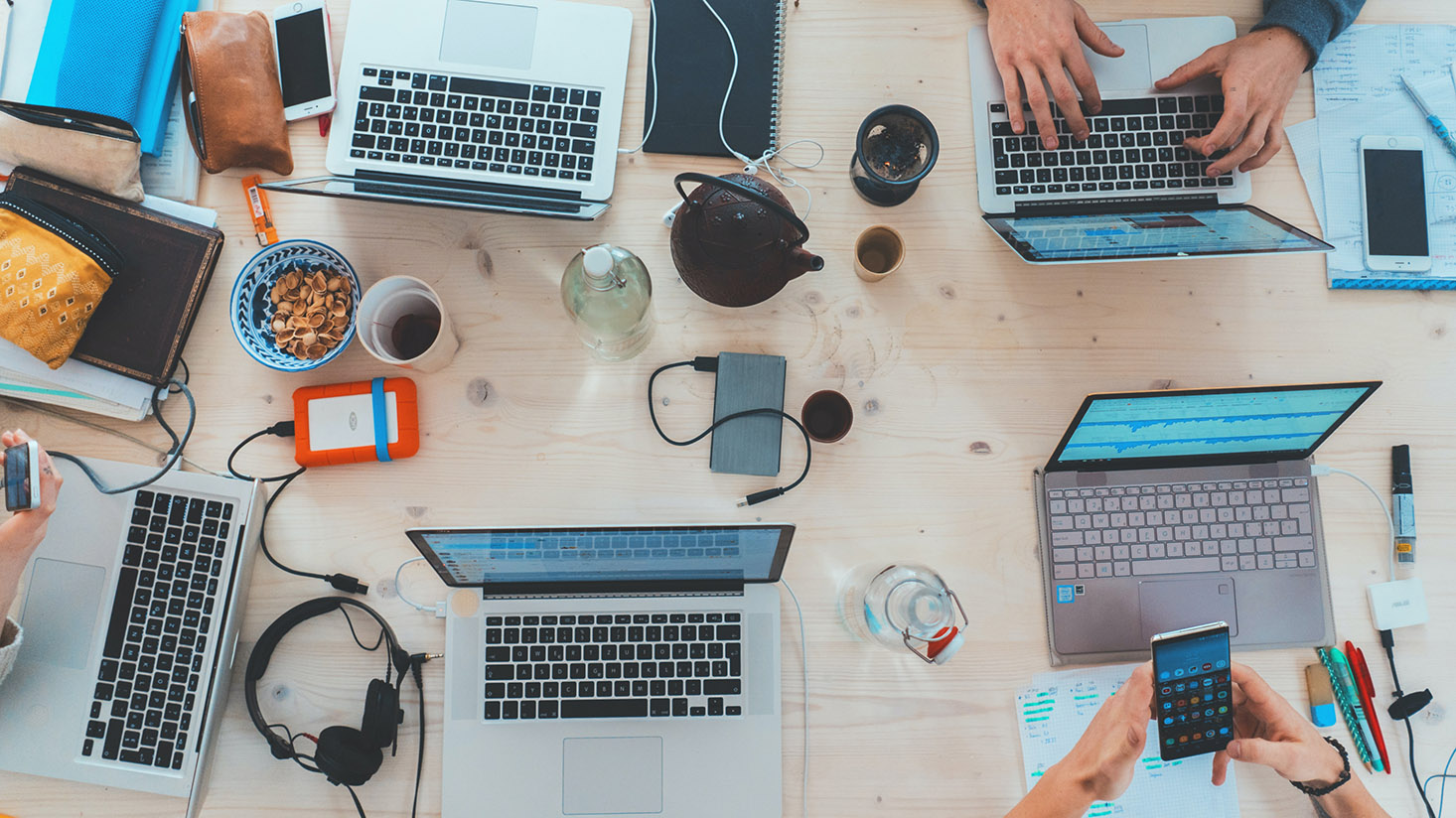
Transform Financial Risk Assessments with Advanced Fraud Loss Insights
Introduction
In the complex world of financial institutions, understanding fraud loss has historically been a formidable challenge. Before the era of big data and technological advancements, banks primarily relied on outdated methods such as manual record-keeping and basic financial audits to estimate potential losses due to fraudulent activities. These early techniques often missed detailed insights and led to protracted decision-making processes.
Before data analytics, banks were often in the dark, making assumptions based on past experiences rather than real-time intelligence. Banks once had to wait for weeks or months to compile and analyze reports, often relying on anecdotal evidence and rough estimations, which could lead to significant strategic missteps.
Historically, the absence of granular data meant that fraud detection and prevention were more reactive than proactive. Financial institutes could only respond to incidents post-occurrence, rather than forestalling them. The lack of timely insights left banks vulnerable to escalating financial risks and a diminished ability to shield their profits effectively.
However, the digital evolution ushered in by the proliferation of categories of data, such as Consumer Behavior Data, has revolutionized this domain. With the internet, connected devices, and sophisticated sensors becoming commonplace, banks are no longer waiting helplessly. Instead, they leverage real-time data to identify trends and mitigate risks proactively.
The infusion of advanced data analytics into the banking sector has transformed risk assessments from reactive to resilient. Now, banks don't just report on fraud; they anticipate it, utilizing data insights to write the new rules in fraud detection and prevention.
Data has become imperative in understanding the nuances of fraud loss. Real-time data access means that financial analysts can monitor threats dynamically, enabling them to execute rapid responses to any signs of fraudulent activities, protecting their assets more vigilantly than ever before.
Consumer Behavior Data
The advent of Consumer Behavior Data has dramatically altered fraud loss inspections in the banking industry. Initially, banks made use of rudimentary data like transaction histories or basic credit score checks, which lacked depth and specificity. Today, with intricate Consumer Behavior Data, they receive a comprehensive view of consumer activities and interactions.
Over the decades, this data type has evolved extensively. Sources like demographic data, purchase patterns, and behavioral analytics have provided banks with new layers of understanding consumer behavior, shifting from surface-level knowledge to profound insights that can predict potentially fraudulent actions.
Industries such as insurance, finance, and retail heavily utilize Consumer Behavior Data. Within the banking sector, fraud analytics specialists and compliance officers can interpret this data to discern patterns that may indicate fraudulent behaviors, leading to preventive measures rather than corrective ones.
The rapidity and volume of consumer data accumulation accelerate daily, thanks to technology advancements like mobile banking apps and e-commerce platforms. With the meteoric rise of online transactions and payments, banks have an expansive dataset at their fingertips to devise more precise fraud detection frameworks.
Specific ways in which Consumer Behavior Data supports banks in fraud detection are:
- Analyzing spending patterns: Helps to identify anomalies and predict suspicious transactions.
- Demographic profiling: Provides insights into consumer payment behaviors based on age, income, location, etc.
- Transactional history: Confirms authenticity by comparing current transactions with historical patterns.
- Lifestyle and preference data: Enhances understanding of consumer intent, helping to refine fraud filters.
- Dynamic scoring algorithms: Builds risk scores in real-time as new consumer interactions occur.
By harnessing Consumer Insights from industry leaders in fraud prevention, financial institutions can bolster their defenses against potential threats with greater precision and foresight.
Fraud Analytics Data
Fraud Analytics Data, consistently refined through advancements in statistical analysis and machine learning, serves as another critical component in mitigating fraud losses. In the early days, organizations faced banks relied on slow-moving audits and batch processing data to get indications of fraud risks. These methods were neither timely nor comprehensive.
The growth of computational power and data storage capabilities has allowed organizations to evolve their efforts from traditional inquiry to state-of-the-art fraud analytics. Software solutions enable the synthesis of vast amounts of transactional data into actionable insights, allowing institutions to bypass inefficiencies and adopt preemptive strategies.
Numerous technological advances have paved the way for fraud analytics, including the development of real-time processing, big data platforms, and predictive analytics. As these technologies proliferate, the volume of useful data grows, enabling analysts to enhance their detection capabilities.
Fraud Analytics Data empowers institutions to formulate precise fraud risk strategies. It can identify fraudulent activities through:
- Correlation Analysis: Detects correlations between disparate data points signaling potential fraud.
- Pattern Recognition: Identifies commonalities in fraudulent behavior across transaction types and consumer profiles.
- Risk Scoring Algorithms: Assigns risk levels to transactions based on anomaly detection and profile matching.
- Network Analysis: Connects dots across different transactional data to discover hidden fraud rings.
- Artificial Intelligence Models: Leverages AI to reveal non-obvious fraud avenues through self-learning models.
The power of modern data search engines has revolutionized Fraud Analytics Data application, providing businesses with the agility needed to stay one step ahead of financial threats.
Conclusion
In summary, the infusion of advanced data analytics into the investigation of fraud loss offers unparalleled insights and protection for financial institutions. By transitioning from outdated methodologies to real-time data-driven approaches, banks are better equipped to anticipate and mitigate risks, fostering an environment of secured financial management.
Understanding and utilizing diverse types of data are crucial for becoming a truly data-driven organization. Data discovery and monetization will play pivotal roles, empowering institutions to unlock hidden value in financial insights and drive informed decision-making processes.
As organizations strive to become more data-centric, exploring innovatory data opportunities will become indispensable. The potential future sale of newer types of data could further transform how financial fraud loss is handled, creating even deeper layers of analysis and protection.
The shift towards data monetization is increasing, with institutions beginning to capitalize on the wealth of data they've accumulated over decades. Financial fraud insights, when commercialized, can offer a competitive edge unheard of in traditional risk assessment methodologies.
The future beckons with intriguing possibilities, where sectors can tap into unexplored data reservoirs and develop cutting-edge fraud detection mechanisms that are both robust and resilient in the face of dynamic fraud landscapes.
Appendix
Industries such as investment, consulting, insurance, and market research stand to benefit immensely from these data insights. Financial regulation and compliance roles within these sectors increasingly depend on robust fraud detection and prevention methodologies, transformed by the data revolution.
Traditionally burdened with lengthy audits and manual processes, these sectors face challenges such as dynamic fraud schemes and regulatory scrutiny. But with the transformative power of data, these difficulties are swiftly evolving into opportunities for innovation and growth.
By embracing data-driven risk analysis, compliance officers, data analysts, and consultants are empowered to make quicker, more accurate decisions, ultimately bolstering the financial health of their organizations.
The future holds exciting prospects, particularly through AI applications, which will further reveal the value encrypted in decade-old documents or government filings related to fraud. With advanced training data discovery practices, they can unlock new rationales and develop sophisticated fraud mitigation strategies.
The gambling of fraud losses, underpinned by the power of data and analytics, is set to revolutionize industry-wide risk practices, making us rethink traditional concepts that once defined financial fraud reasoning.
The move towards monetizing data is a revolutionary step forward, as the intersection of legacy systems and modern technology promises a future where fraud loss insights are not just accurate, but also a crucial differentiator in a rapidly changing financial landscape.