Transform Healthcare Insights with Blood Cancer Diagnostic Data
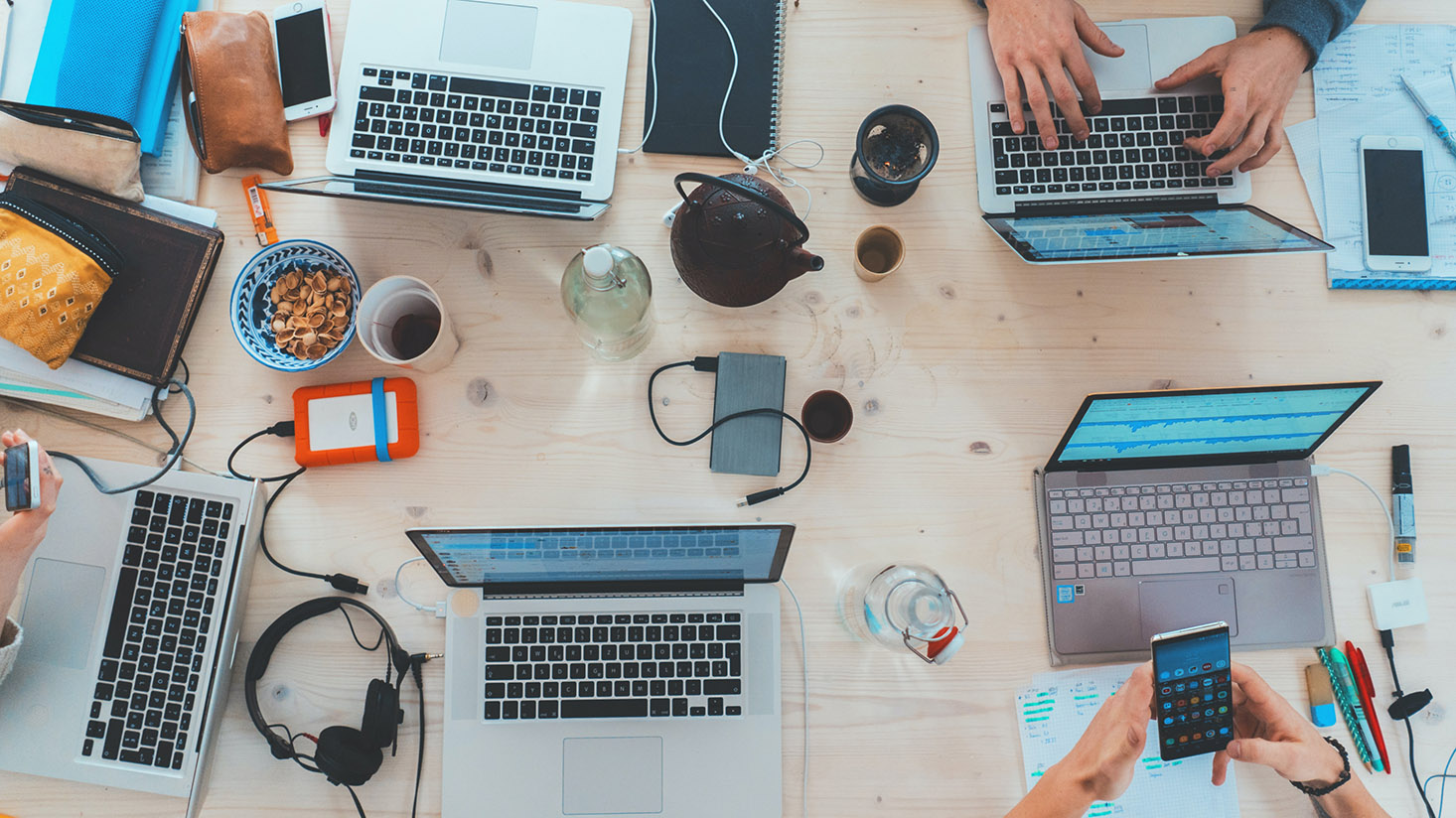
Introduction
Understanding blood cancer diagnostics through comprehensive data analysis offers a transformative approach to healthcare insights. Historically, gaining a clear perspective on this critical area was fraught with challenges. In the past, before data protocols and intricate record-keeping, medical professionals relied heavily on anecdotal evidence and individual experience. This resulted in a tapestry of fragmented insights, often leading to generalized assumptions that could not fully account for the complexity of blood cancer diagnoses. The evolution from reliance on sparse data like handwritten hospital logs to the vast databases of today has significantly enhanced our understanding.
The emergence of the internet and connected devices revolutionized data gathering, delivering detailed insights at unprecedented speed. Previously, understanding trends and changes in blood cancer diagnoses could take weeks or even months. Now, with the integration of real-time data collection mechanisms, stakeholders can access up-to-the-minute information, enabling quicker responses to emerging trends and needs, thus reducing the time lag between diagnosis and intervention.
The advent of electronic medical records (EMR) systems and healthcare informatics has further expanded the horizon for medical data management, providing comprehensive datasets that include individual patient histories, treatments, and outcomes. This transition from manual record-keeping to digital data warehousing is a landmark evolution in healthcare analytics, playing a pivotal role in fostering an environment where detailed data-driven insights can be promptly obtained and utilized.
Data, therefore, is not merely a static record but a dynamic component that drives effective decision-making processes in healthcare. It has become indispensable for ensuring optimal outcomes in blood cancer treatment and research. Timely access to data allows practitioners to identify trends, draw correlations, and develop predictive models that were previously unimaginable. The scope for data-led initiatives will only expand as healthcare systems continue to digitize their operations.
In this article, we will delve into key categories of data relevant to understanding blood cancer diagnoses and explore how these types of data can illuminate insights into unmet needs for bone marrow transplants across various demographics. By uncovering data trends—including age, race, comorbidities, and geographic location—healthcare professionals can comprehend the broader landscape of blood cancer diagnoses and better target resources.
The potential for data-driven insights is enormous, where once there was guesswork and delay. Modern datasets allow for accurate, real-time diagnostics, offering an unparalleled depth of understanding that can save lives and optimize the care journey from diagnosis to treatment.
Healthcare Data
Background and Historical Context
Healthcare data encompasses a wide range of information gleaned from individual patient encounters, aggregated diagnostic codes, treatment outcomes, and more. Historically, this type of data was preserved in physical formats such as patient charts, handwritten notes, and administrative paperwork. It was collected painstakingly over time and represented a significant administrative burden on healthcare providers. Categories of data like these were often siloed, inaccessible to those who needed them most, and prone to inaccuracies or loss.
The digital revolution in healthcare saw the rise of electronic health records (EHRs) in the 1990s and 2000s. With the advance of software solutions for health management, these records became integral to patient care, allowing for easier exchange of health information across systems. Contemporary healthcare data includes a vast array of fields and access to real-time patient monitoring through connected devices, offering granular insights into patient health.
Accelerating Data Utilization
Healthcare data has traditionally been used by epidemiologists, researchers, policy makers, and clinicians. This expansive use base has only grown over time as more sophisticated analysis tools emerged. Innovations such as machine learning and artificial intelligence use vast datasets to anticipate disease trends and recommend treatment plans, fundamentally shifting how healthcare providers interact with patient information. Organizations are often found utilizing external data to complement their own records, revealing unperceived insights and advancing scientific research.
The scale and speed at which healthcare data is produced are breathtaking. With millions of diagnoses and billions of claims processed annually, the volume of information available continues to grow. This surge is driven by advances in digital health, such as wearable technology and remote health monitoring. These innovations provide additional data streams, further enriching the landscape of healthcare analytics.
Applications to Blood Cancer Diagnostics
Comprehensive healthcare data provides a solid foundation for analyzing blood cancer diagnostic patterns. Specifically, data can be segmented and utilized to address various facets of the diagnostic process, leading to clearer trends and actionables:
- Age Distribution: Analyzing age-specific data helps identify at-risk demographics, allowing for strategic intervention planning.
- Diagnosis Timestamp: Evaluating diagnosis timelines ensures timely referrals and appropriate treatment initiation.
- Comorbidity Data: Understanding associated health conditions enables holistic patient care approaches, identifying potential barriers to successful treatment.
- Hospital Analysis: Identifying which institutions are diagnosing more effectively aids in resource allocation and benchmarking successful protocols.
- Variable by Race and Location: Disaggregating data by race and geographic location sheds light on disparities in diagnostic rates and treatment accessibility.
The impact of healthcare data within the realm of blood cancer diagnostics is profound, equipping clinicians and researchers alike with actionable insights to innovate and improve upon existing diagnostic processes.
Patient Demographic Data
Historical Use and Collection
Patient demographic data provides a snapshot of the social and personal attributes of individuals in healthcare settings. Traditionally gathered through patient registration and survey instruments, these data points included essential details such as age, gender, ethnicity, and economic status. Historically, demographics were recorded on physical forms that restricted data aggregation and analysis capabilities.
With digital transformation, patient demographic data is now collected instantly and maintained within expansive health information networks. These datasets are more comprehensive and easier to manage than ever before, and are curated to reveal essential healthcare trends, patient risk predictions, and program effectiveness comparisons.
Modern Applications and Analysis
Patient demographic data is vital to diverse stakeholders, from healthcare providers to policy makers. These roles leverage demographic insights to strategize business plans, allocate resources, and innovate patient care pathways. As the health data landscape evolves, more intricate models and analyses are emerging, supporting proactive rather than reactive health management.
Technological advances enhance data acquisition, permitting intricate detail and continuous updates as demographic shifts occur both regionally and internationally. These data points serve as the basis for extensive qualitative and quantitative research, establishing patterns and forecasting future needs for various healthcare services.
Impact on Understanding Blood Cancer
The integration of patient demographic data in understanding blood cancer diagnostics offers multiple benefits:
- Ethnic and Cultural Insights: Diverse populations can experience varied incidences of blood cancer. Demographic data helps identify these trends.
- Geographic Concentrations: Locating high-incidence zones aids in focusing diagnostic and treatment efforts.
- Socio-economic Impacts: Recognizing socio-economic factors in cancer prognosis enhances access reform and equity in care.
- Age-Specific Interventions: Aging demographics require unique diagnostic pathways and treatment options.
- Resource Distribution: Understanding demographic clusters helps institutionalize resource allocation.
This category of data underscores the critical role of understanding AI and smart analytics in tackling age-old healthcare issues through targeted insights.
Conclusion
Engaging with multifaceted data types is pivotal in transforming healthcare perspectives, particularly in enhancing the understanding of blood cancer diagnostics. The correlation of vast datasets ranging from healthcare and patient demographics to treatment outcomes illuminates the path from provisional assessments to strategic healthcare solutions.
Access to diverse data streams enables a holistic approach to recognizing and addressing unmet healthcare needs globally. This increased data literacy fosters a significant shift towards data-driven strategies that harmonize interventions with patient-specific needs, exemplifying the ongoing evolution towards more efficient healthcare delivery systems.
Corporations are capitalizing on this dynamic by seeking to monetize their data, potentially unlocking decades' worth of valuable insights. Firms that were once focused solely on operational improvements are now recognizing the expansive possibilities of data as a commercial asset in the evolving healthcare landscape.
Predictive analytics is paving the way for new types of data to emerge, such as genomic sequences or real-time biochemical markers in diagnosing diseases. This data may allow researchers to delve deeper, uncovering insights that might redefine our understanding and treatment of blood cancer and other complex conditions in the future.
As the healthcare industry embraces this ongoing data renaissance, businesses are better positioned to enhance patient outcomes, streamline operations, and explore new avenues of discovery. The acceleration of data insights marks a new chapter in healthcare, where innovation and data amalgamate to transform patient experiences.
Ultimately, the alignment of technology and healthcare data can catalyze sustained improvements across the healthcare spectrum, enabling a brighter and healthier future for all.
Appendix: Industries and Roles Benefiting from Data
The transformative potential of healthcare data is not restricted to a singular domain but spans multiple industries and roles, creating ripples of change across sectors.
Consultants and Market Researchers
Consultants serve a critical role in harnessing data insights to advise healthcare providers on operational efficiencies and strategic growth. They interpret datasets from healthcare facilities to offer customized, data-centric solutions that optimize patient care pathways and business augments.
Market researchers compile and analyze external data, including diverse patient demographics, disease prevalence, and healthcare accessibility, to identify emerging trends and potential markets for innovation. Their insights foster investment and development in both traditional and nascent healthcare streams.
Insurance Companies
Insurance companies utilize healthcare data to forecast potential risks, develop tailored coverage plans, and implement value-based care models. Through advanced analytics, they assess health claims data, offering personalized insurance solutions aligned with patient-specific health risks.
As predictive algorithms become more robust, insurance companies will continue to personalize offerings, ensuring appropriate risk mitigation strategies and pricing models, thereby enhancing overall health policy formulation and consumer satisfaction.
Investors
Investors recognize the burgeoning potential of healthcare data to identify lucrative opportunities within the healthcare sector. They employ data-driven approaches to assess the growth potential of innovations, ranging from telemedicine to AI-enhanced diagnostic tools, often leveraging training data sources. For more information on discovering training data, visit this resource.
Investors predict technological disruption within the healthcare industry and align investments accordingly to maximize returns while fueling advancements that cater to unmet patient needs.
Artificial Intelligence and Its Implications
The incorporation of AI in healthcare is unlocking new dimensions in data interpretation. With its ability to process large volumes of data rapidly, AI helps uncover hidden patterns, predictions, and insights that might remain unnoticed with traditional methods, significantly optimizing diagnostic and treatment processes.
The future sees AI being central to synthesizing information from various sources, paving the way for smarter, more informed healthcare decisions.
The continued integration of AI capabilities, alongside expanding datasets, is likely to redefine fundamental aspects of diagnosis, treatment planning, and resource management in healthcare, with AI potentially unearthing deep insights that could transform patient outcomes.