Uncover Consumer Financing Trends with Innovative Dataset Insights
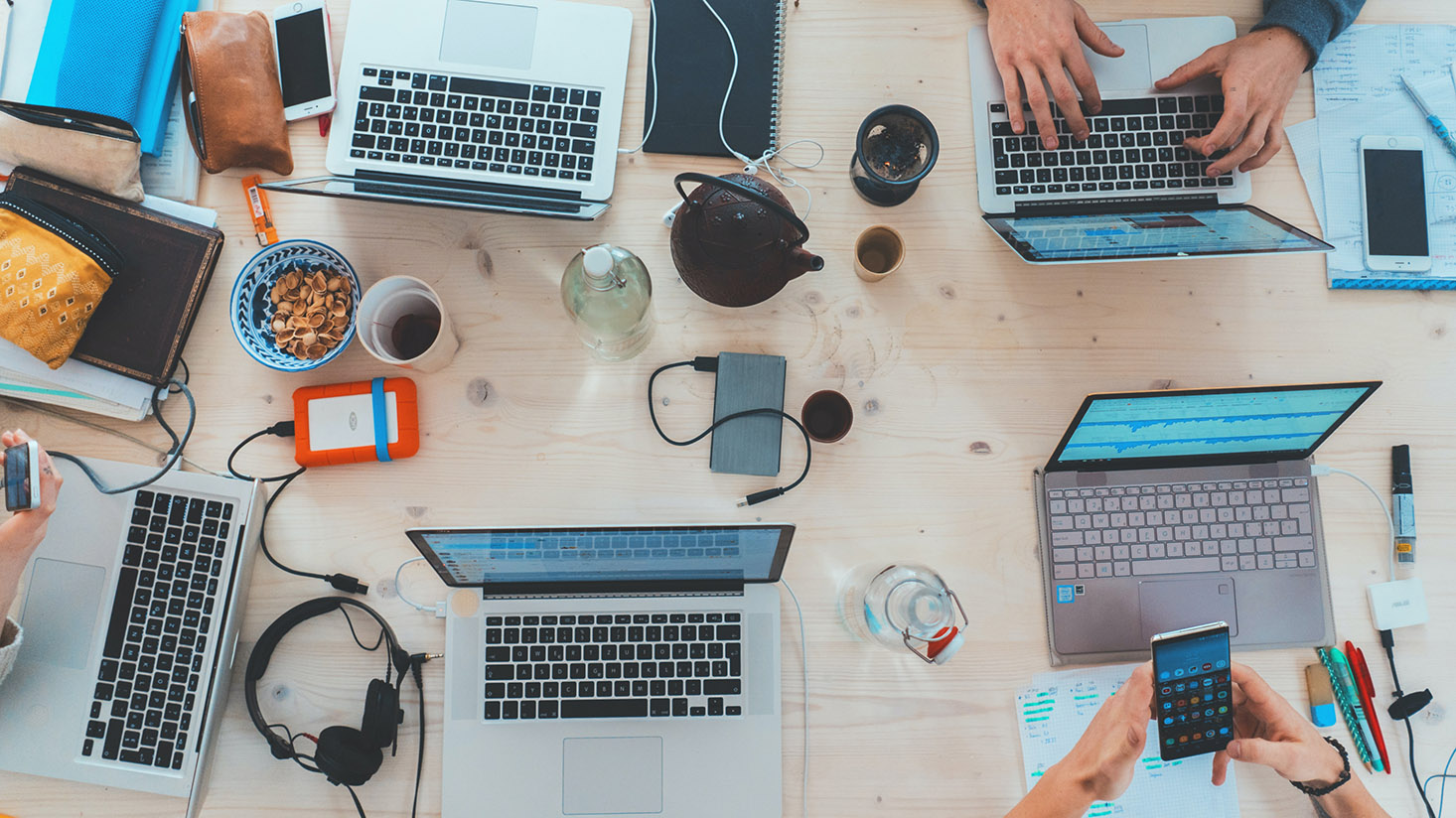
Introduction
Understanding the intricate dynamics of consumer financing has always been a challenging endeavor. Historically, businesses seeking to comprehend these trends relied heavily on minimal institutional reports, rudimentary surveys, and anecdotal evidence. Before the age of comprehensive datasets, companies faced a murky horizon, often left questioning crucial financial patterns without swift and actionable answers. The absence of real-time data meant that by the time updates reached decision-makers, the market landscape had already transformed. This lag turned analysis into a game of guesswork, rather than informed strategy.
In earlier days, lacking modern data infrastructure, businesses would rely on small-scale consumer surveys or sporadic financial reports from banks and other lending institutions. These methods, although useful to an extent, painted only part of the picture. It wasn’t uncommon for firms to wait weeks or even months for records of consumer trends, by which time the data was often outdated, and the competitive advantage—lost.
However, the digital revolution has orchestrated a paradigm shift in data acquisition. The advent of advanced sensors, the internet, and connected devices has brought about a deluge of accessible insights. This transformation, catalyzed by the proliferation of sophisticated software and databases, enables businesses to track every transaction or financing step as it happens in real time. Suddenly, historical financing behavior became a domain rich with instant insights.
The proliferation of e-commerce platforms and the adoption of digital financing methods like 'Buy Now, Pay Later' further highlight the need for immediate, accurate data. Today, understanding how many products are purchased via financing options, like a fitness bike and its associated app, involves more than just sales figures. It requires the dissection of consumer behavior, transaction patterns, and financing terms.
Such insights are no longer obscured by guesswork but are illuminated by diverse datasets encompassing consumer behavior and transaction data, among others. With the right external data, businesses can unlock unprecedented access to consumer financing trends. Discovering these patterns is no longer about prior assumptions but about empirical evidence derived directly from consumer activities and preferences.
As we delve into the various types of data that illuminate these consumer financing trends, the emphasis will be on understanding how these datasets not only answer primary questions but also offer layers of nuanced insights that can drive strategic business growth and adaptation.
Consumer Behavior Data
In exploring consumer financing trends, one foundational data type stands out—consumer behavior data. Historically, this data category has been pivotal for industries ranging from marketing to retail, enabling businesses to tailor their approaches based on consumer inclinations and activities. This data type emerges from various sources, including surveys, tracking of mobile application usage, and direct consumer feedback mechanisms.
Originally, understanding consumer behavior was limited to focus groups and manual surveys. Advances in technology have since redefined this landscape. The continuous development of targeted applications and online platforms has permitted the collection of extensive user activity data, proliferating at a remarkable rate. Devices capture and transmit vast amounts of information, transcending simple purchase histories to encapsulate detailed behavioral patterns.
Today's consumer behavior datasets provide invaluable insights into financing trends. For example, a data provider might use device feed tracking to identify which applications consumers frequently engage with and ascertain if fitness apps or financing providers are being employed. By linking transaction data with user surveys within specific ecosystems, illuminating questions—like whether a fitness product is purchased via a financing option—can be directly addressed.
Specific applications of consumer behavior data in understanding financing include:
- Application Usage Tracking: Understanding which finance-related applications are being used, and matching their usage to purchases.
- User Surveys: Deploying targeted questions within user ecosystems to capture data on whether products are purchased through credit or financing models.
- Data Linking: Correlating transactional and behavioral data to make informed predictions about the use of financing options.
- Trend Analysis: Using accumulated consumer behavior data to predict future financing trends and strategies.
- Cross-Platform Insights: Identifying how consumers interact across different platforms, shedding light on their financing preferences.
This data type's potential lies not merely in pinpointing purchase methods but in revealing the underlying motivations and habits, paving the way for deeper understanding and strategic foresight.
Transaction Data
Another powerful data type to illuminate consumer financing trends is transaction data. By nature, transaction data chronicles every financial interaction a consumer undertakes, offering a detailed audit trail of monetary behavior. In the world of commerce, understanding transaction trends is equivalent to holding the keys to the kingdom.
Transaction data traces its roots to bank ledgers and generic reports. With digital transformation steering financial transactions toward plastic cards and online processes, transaction datasets have rapidly grown in volume and detail. Modern transaction panels aggregate debit and credit transactions across myriad financial institutions, providing a comprehensive view of consumer spending and financing habits.
Businesses leveraging transaction data can decipher numerous financing mysteries. For instance, identifying when a particular product like a fitness bike is financed through 'Buy Now, Pay Later' models becomes pragmatic. Despite the absence of SKU-level specifics, sophisticated data solutions can align transaction timestamps and amounts to derive informed assumptions about financing methods.
Here are some ways transaction data provides rich insights into financing trends:
- Purchase Pattern Recognition: By examining transactions linked to both fitness providers and financing applications, patterns indicating financed purchases can be tracked.
- Cross-Referencing Applications: Connecting transaction timings with software application activations to infer financing starts and modalities.
- Comparative Analysis: Conducting comparative studies of transaction data across different financiers to optimize lending strategy.
- Recurring Payment Tracking: Identifying recurring payments linked to specific products indicates ongoing financing arrangements.
- Market Segmentation: Determining consumer financing behavior across demographic segments for targeted market strategies.
Transaction data’s strength is its ability to comprehensively present the monetary flow, crucial for dissecting complex financing patterns across industries.
Web Traffic Data
In today's connected world, the digital footprint left by users navigating the web forms an emerging category of data with immense potential—web traffic data. As consumers browse online retailers and select financing options, web traffic datasets can reveal much about how users interact with financing services.
The evolution of web traffic data originates from simple server logs containing basic traffic data. Contemporary technologies, backed by cookies, browser tracking, and sophisticated referral analytics, have catapulted web traffic data to a new echelon of detail and utility. These datasets highlight how users navigate, what sites they visit, and, importantly, how they engage with financing platforms embedded within e-commerce sites.
Businesses leverage web traffic data to gain macro and micro insights into financing preferences. For instance, whenever a consumer selects a financing option at checkout, their interaction with the financing provider's website, like logging into Affirm, is a revelatory data point.
Specific applications of web traffic data to discover financing trends include:
- Referral Tracking: Monitoring outbound traffic to financing platforms during an e-commerce transaction to discern financing uptake.
- Checkout Process Analysis: Analyzing the checkout steps taken during purchases to understand the financing adoption rate.
- Consumer Pathway Insight: Tracing the consumer journey from product selection through to financing finalization.
- Cross-Site Interactions: Observing interactions across multiple e-commerce sites and financing platforms for an integrated view.
- Trend Prediction: Utilizing traffic flow data to forecast shifts in financing preferences.
Web traffic data opens vistas for understanding the crossover between traditional commerce and new-age financing, presenting both challenges and opportunities navigable with data-driven insights.
Conclusion
The role of diverse datasets is undeniable in unraveling the complexities of consumer financing trends. As we have explored, each type of data—ranging from consumer behavior to transaction to web traffic—brings unique perspectives and value to the table. These datasets empower businesses to not only answer specific questions about consumer financing methods but also to develop comprehensive strategies for growth and adaptation.
The increasing trend towards a data-driven approach in businesses underscores the necessity of integrating these various data types. By unlocking the true potential of these datasets, companies can transform the way they perceive consumer behavior, enabling more responsive and dynamic adaptation to market changes.
Moreover, the idea of data monetization continues to revolutionize the landscape. Organizations are identifying the intrinsic value embedded within their proprietary data, and many are taking steps to monetize insights that have long been dormant. Consumer financing trends are no exception, as data-driven insights provide rich avenues for gaining competitive advantage.
Looking to the future, one might speculate on the new data types that could emerge in the market. The digital world continues to generate vast swathes of information, and future datasets may harness unprecedented insights from AI-analyzed social media trends, voice-activated purchasing patterns, or even blockchain-based financial transactions.
As industries strive to become more agile and informed, the integration of varied datasets into strategic initiatives could distinguish thriving businesses from their peers. The understanding and application of data to illuminate consumer financing patterns are poised to transform industry practices, ushering in a new era of strategic intelligence and enhanced decision-making.
Appendix: Benefiting Industries and Roles
Countless roles and industries stand to benefit significantly from insights delivered by rich datasets. Among them, investors, consultants, market researchers, financial analysts, and product developers find datasets invaluable in their quests for answers and trends concerning consumer finances.
For investors, data-driven insights aid in making educated decisions about market predictions and investment opportunities. They can draw on transaction trends and consumer behavior analysis to make informed financial forecasts and portfolio decisions.
Consultants leverage such data to craft tailored solutions for businesses, providing grounded strategies built on empirical insights rather than assumptions. Market researchers find significant value in consumer finance datasets, enabling them to foresee trends and drive market innovations.
Industries such as insurance find that datasets can revolutionize risk assessment. When coupled with AI algorithms, insurance companies can analyze consumer transactions and spending habits to tailor premiums and coverage more effectively.
The future promises fascinating possibilities in data application. As AI evolves, the potential for unlocking insights from decades-old documents and modern government filings becomes tantalizingly possible. These tools could enable individuals and businesses to unearth valuable trends and hidden opportunities within existing data landscapes.
Ultimately, the journey towards data enlightenment has only begun, and as these datasets continue to expand and evolve, the beneficiaries will be those proactive enough to embrace their potential in orchestrating strategic change and achieving sustainable growth.