Uncover Market Patterns with Historical Futures Trading Margin Data
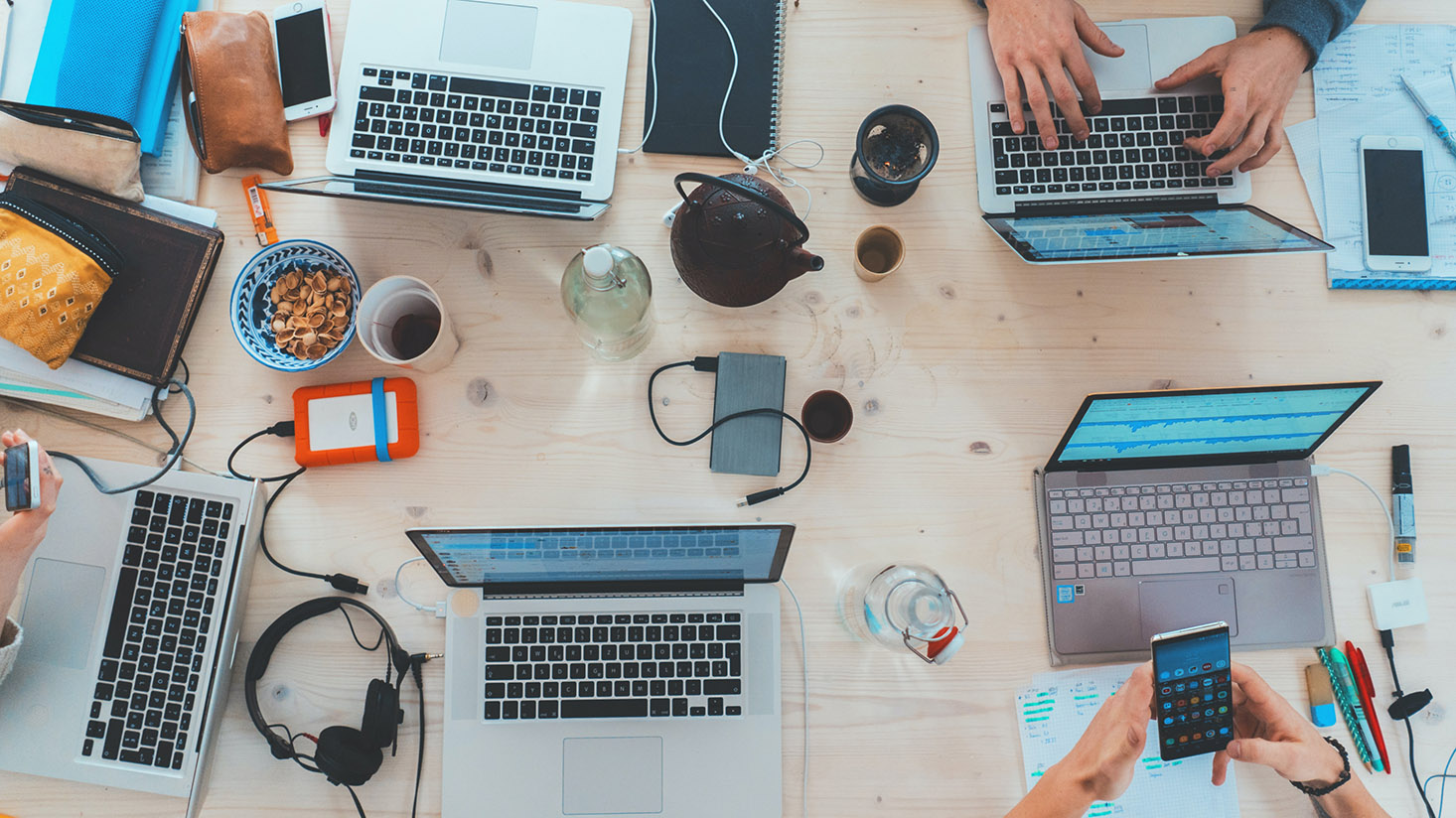
Introduction
Understanding the complexities of global futures markets has always been a strenuous task, fraught with challenges and requiring nuanced insight into various market signals. Historically, obtaining clear insights into trading margin requirements for futures was akin to navigating a labyrinth without a map. Before we had the luxury of purchasing comprehensive datasets, market participants relied on antiquated methods that were often cumbersome and rife with delays.
In the nascent days of futures trading, insights were primarily based on rudimentary paper records and manual calculations, lacking the finesse and accuracy we now take for granted. Back then, traders and analysts depended on reports that were weeks, if not months, out of date. Simply put, the path to understanding margin requirements was marred by time lags and inefficiencies, leaving little room for rapid decision-making that the fast-paced futures market demands.
The introduction of the internet, along with the proliferation of connected devices, brought forth a seismic shift in how data was collected and used. It catalyzed the creation of expansive databases that could store rich troves of information, offering unprecedented visibility into market operations. This was further enhanced by technological advances in sensors and computational capacities, which transformed data collection from a slow, manual process into one that could happen in real-time.
The ability to access real-time data has revolutionized the futures market, turning guesswork into informed strategies. Where once traders waited on pins and needles for sporadic insights, today they can analyze shifts in trading margins almost instantaneously. This transformation underscores the critical role that data plays in understanding not only market dynamics but also in crafting strategies that are proactive and resilient.
Moreover, firms are now realizing the importance of leveraging historical trading margin data to refine their strategies over the long term. Rather than reacting to market volatility as it arises, they can now back-test their strategies using historical datasets, allowing them to build robust, future-proof economic models. This leap from reactive to predictive and proactive management illustrates how integral data is in the continual evolution of market strategy.
The transition towards a data-driven market landscape has set the stage for unprecedented opportunities. Not only does it provide transparency and efficiency, but it also drives competitive edge, allowing those who harness it to navigate and excel in the intricate corridors of global futures trading.
Financial Markets Data
Historical Trading Margin Data
The journey of Financial Markets Data begins at the confluence of necessity and possibility. Historically, this type of data was not readily accessible, leaving traders dependent on piecemeal updates from exchanges. Fast forward to today, the narrative has dramatically shifted. Companies now provide comprehensive datasets covering up to 95% of major global futures exchanges, encapsulating information from initial to maintenance margin data crucial for market participation.
Originally, the pursuit of compiling such expansive datasets was challenging, inhibited by technological limitations. The subsequent tech advancements, like categories of data APIs and CSV data deliverance, have catalyzed the democratization of this data, allowing traders unrivaled access for over a decade, spanning as far back as 2014.
The importance of this data in the financial sector cannot be overstated. Financial analysts and traders employ it to assess market risks, craft hedging strategies, and back-test trading strategies against historical market conditions. The dynamic nature of external data catapults it to a league of its own, a robust resource for predictive analytics. This has fundamentally blurred the lines between speculation and informed decision-making.
Local exchanges worldwide enrich datasets with nuanced, market-specific insights, transforming generic global data landscapes into hyper-localized economic narratives. This provides substantial insight into regulatory shifts and evolving market trends, invaluable for financial strategists.
How Historical Financial Markets Data Can Be Used: This powerful dataset can help professionals realize objectives with precision and foresight.
- Risk Assessment: Historical margin data helps identify potential points of vulnerability within trading portfolios.
- Historical Comparison: Enables traders to draw comparisons between current and past market dynamics for better future predictions.
- Investment Strategy Development: Market data facilitates the development of forward-looking investment strategies tailored to mitigate risks while optimizing returns.
- Regulatory Compliance: Assists firms in adhering to market regulations by maintaining a comprehensive historical record of trading insights.
- Market Correction Predictions: Allows market analysts to forecast potential corrections based on past trends, preventing major economic setbacks.
Integration with Modern Market Mechanisms
The evolution of markets, paralleled by the accessibility of historical data, plays a crucial role in today’s hyper-competitive environment. By harnessing historical data, market operators create enhanced predictability in trading actions. Through backtesting strategies, firms enhance resilience amidst market fluctuations.
Individuals and entities alike are beneficiaries of this evolving data trajectory. From policymakers drafting fiscal strategies to traders fine-tuning market entries, historical data has unequivocally metamorphosed from simple informational undercurrent to a potent market force.
The sheer volume and velocity of data available today means strategic insights are now only a query away—reliable, instant, transformative.
Financial Data Provider
Margin and Settlement Prices Data
Financial Data Providers supplement market understanding by offering insights beyond traditional trading margins. These providers enrich datasets with related metrics like settlement prices, being of paramount importance to traders, analysts, and economic policymakers.
Diversification of data—ranging from market futures settlement prices in the US to Montreal, Euronext, and ICE Europe futures—provides investors with a wealth of information to make better-informed decisions. Historically, this kind of data was disparate and fragmented, but it now converges into a consolidated format, thanks to advancement in data technologies and the capabilities of modern financial platforms.
The advances in AI and machine learning have further catalyzed the transformation of how data is utilized. Algorithms sift through massive datasets to identify trends, anomalies, and opportunities, turning raw data into valuable financial intelligence. As seen in resources discussing the importance of AI, it’s not only about the data quantity but also the quality and analytical frameworks applied.
How Financial Data Providers Transform Market Cognition:
- Strategic Planning: Financial data is crucial for formulating long-term strategic plans and objectives.
- Market Entry Decisions: Firms rely on market data to guide cost-effective and timely market entries.
- Price Setting and Negotiations: Settlement price data plays a pivotal role in price negotiation strategies.
- Competitive Analysis: Highlights areas of competitive edges and gaps, guiding business realignments.
- Real-time Updates: Enables rapid response to market shifts with instant access to updated datasets.
Quantitative Analysis Advancements
Modern financial markets rely heavily on quantitative analysis to sift through the extensive datasets made available by financial data providers. Historical margin and settlement price data have fueled these analyses, paving the way for quantitative researchers globally to dissect market behavior with mathematical rigor, ultimately refining and validating financial models.
Quantitative metrics derived from these datasets are utilized across various sectors, including hedge funds, market research firms, and regulatory bodies, ensuring consistent, forward-moving development within the financial ecosystems.
Conclusion
The reliance on trading margin data and related financial metrics has firmly gripped the economic landscape, transitioning traditional methods into forward-facing, predictive analyses with real-world applicability. The marriage between historical data availability and technological innovation empowers firms to bridge gaps that once seemed insurmountable.
By fostering a data-driven culture, businesses have not only enhanced operational efficiencies but also constructed adaptive strategies capable of preemptively maneuvering through market volatilities. The advent of data monetization is further enriching this cultural shift, enabling entities to extract and monetize insights from underutilized datasets, driving the upward tide of innovation.
Future exploration into market behaviors may even unveil newfound external data niches, providing richer, untapped potential insights into manifesting trading advantages. The expansion of AI to unlock these potentials within both modern and historical contexts remains a keen area of exploration.
Holistically, the importance of historical data in forging robust economic strategies cannot be overstated. It acts as both a shield and a compass—providing necessary protection against uncertainties while guiding towards opportunities facilitated through strategic foresight.
As the trajectory of data collection aligns with emergent market needs, the importance of data discovery and dataset diversification becomes clear. The integration of training data methodologies further enhances this trend, reinforcing the collaborative potential between traditional datasets and advanced analytics methodologies.
Appendix
Industry and Role Transformations
The financial landscape has greatly benefited from the integration of extensive datasets, impacting various sectors and roles. For instance, investors now possess tools to make more accurate predictions about market performance, driven by data-driven insights. Subsequently, this offers a competitive edge in strategic planning and real-time response capabilities.
The world of consultancy also harnesses these datasets to provide clients with data-backed insights, significantly enhancing advisory services with measurable, evidence-based strategies that align with client objectives.
Insurance companies, capitalizing on historical financial trends, are better positioned to assess risks and price premiums more accurately, contributing to the insurance sector’s innovation and consumer satisfaction.
Market researchers analyze these historical datasets to glean trends and trajectories within financial landscapes, applying insights to various industries, driving targeted growth and strategic market flips.
In speculating future data trends, the rise of AI will undoubtedly play a transformative role. Its ability to process and analyze vast datasets reveals hidden potentials in historical contexts and modern market filings, streamlining operations and unlocking value previously inaccessible to firms.
The outlook for historical futures trading margin data is replete with promise. Its application across diverse sectors illustrates not just a revolution in understanding markets, but a foundational shift in strategic planning, capable of heralding a more informed, resilient future.