Uncover Retail Trends with US Consumer Transaction Insights
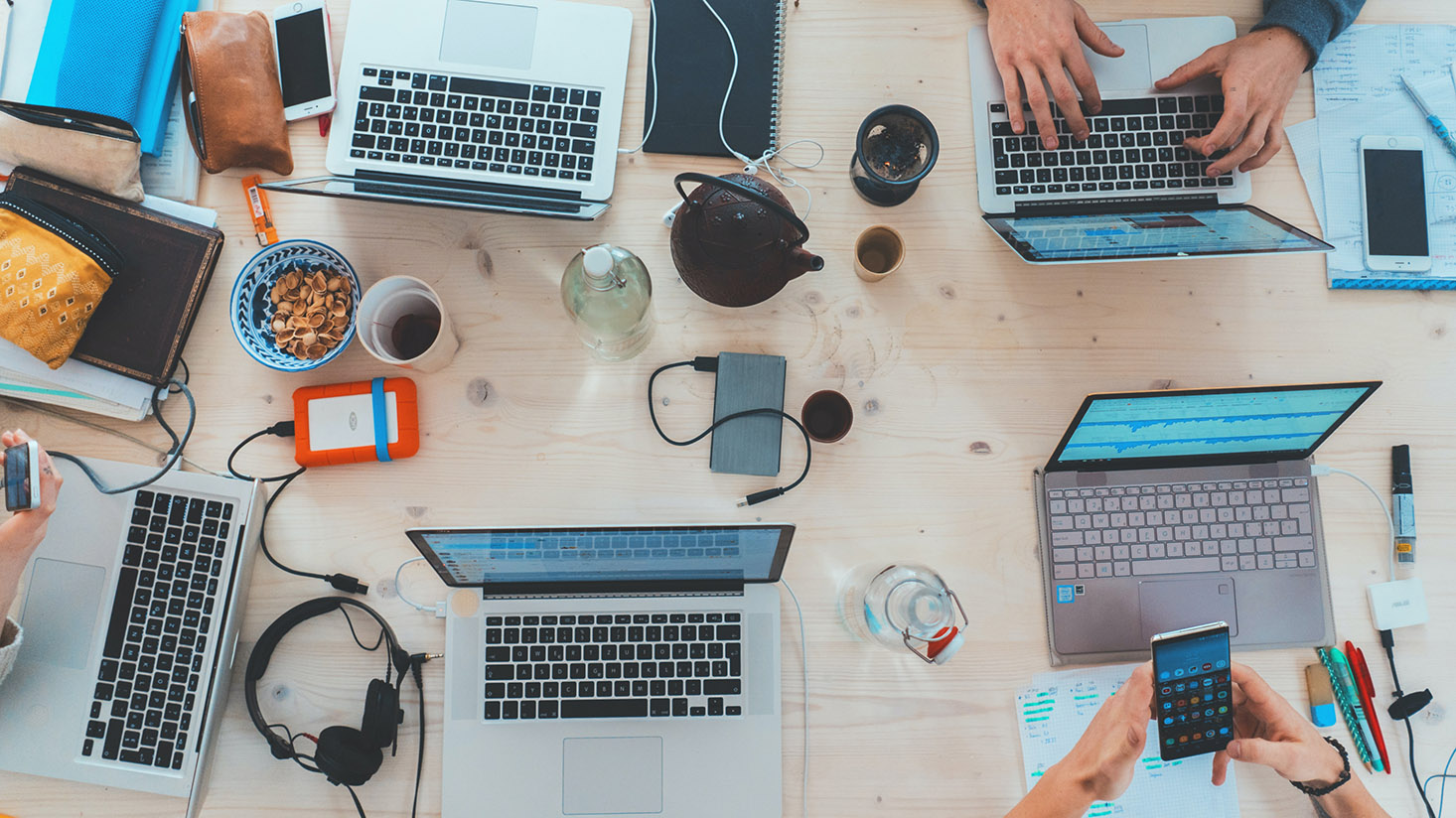
Uncover Retail Trends with US Consumer Transaction Insights
Introduction
In today's fast-paced marketplace, understanding consumer transaction behaviors is crucial for businesses looking to gain a competitive edge. Historically, capturing granular insights into consumer spending patterns was a challenging endeavor, often riddled with delays and inefficiencies. Before the digital age, companies relied heavily on traditional methods such as surveys, manual audits, and limited anecdotal evidence. These approaches offered fragmented insights at best and often missed the broader picture.
As retail shopping shifted from local stores to sprawling malls and eventually online platforms, the need for comprehensive data intensified. Before the proliferation of transactional data, businesses were left in the dark, making decisions based on outdated quarterly reports and annual analyses. This often meant waiting weeks or even months to understand market shifts and consumer preferences.
The transformation came with the advent of digitalization and connected devices. The internet became a reservoir of information, enabling real-time data collection through online payments, e-commerce platforms, and sophisticated point-of-sale systems. With connected digital tools, smart analytics, and automated transaction logs, businesses could finally obtain detailed and reliable consumer insights.
The importance of data in understanding consumer behavior cannot be overstated. What was once an obscure process, filled with uncertainties, is now an integral component of strategic planning. Retailers and manufacturers are no longer guessing; they are methodically tracking consumer preferences, purchase frequencies, and spending volumes with precision.
The digital transformation allows for a broader reach, encompassing transactions across both online and offline platforms. This amalgamation of technology and commerce has birthed a variety of transaction datasets, which offer unparalleled insight into consumer behavior. With this data, companies can respond promptly to changing market dynamics, optimize their strategies, and enhance customer experiences.
In this article, we will delve into the different categories of data integral to gaining insights into US consumer transactions. These data types are instrumental in painting a detailed picture of consumer spending, enabling firms to make informed decisions swiftly and efficiently.
Transaction Data
Transaction data has become a cornerstone in the analysis of consumer behavior, offering a goldmine of information about where and how consumers spend their money. Historically, transaction data was primarily extracted from credit card networks and bank statements, providing only a high-level look at consumer spending patterns. It was limited by geography and often lacked the depth needed for strategic insights.
Today, the scope and depth of transaction data have evolved exponentially. With the rise of e-commerce and digital payment platforms, there’s a continuous surge in the volume and variety of transaction data available. Modern transaction data encompasses cashless payments, mobile transactions, online purchases, and more, offering a picture of consumer behavior that is both comprehensive and detailed.
Various industries, including retail, finance, and marketing have historically leveraged transaction data to drive decision-making. Retailers use it to personalize marketing campaigns, finance professionals employ it for consumer credit assessments, and marketers turn to it to understand brand loyalty and consumer preferences.
The acceleration of technology, such as AI and machine learning, has further transformed the way transaction data is utilized. By analyzing patterns and detecting anomalies, businesses can predict future spending behaviors and craft strategies that resonate with their audience. Transaction data is essential in real-time tracking of shifts in consumer preferences, allowing for agile business adaptations.
Examples of Transaction Data Usage
- Retail Sales Analysis: By examining detailed patterns of purchases across retailers, businesses can identify top-selling products and adjust inventory strategies accordingly.
- Market Trend Predictors: Transaction data helps in forecasting market trends by identifying shifts in consumer spending.
- Competitive Benchmarking: Understanding where consumers are making their purchases helps businesses benchmark against competitors.
- Customer Segmentation: Detailed transaction data supports creating unique consumer profiles, aiding in targeted and effective marketing strategies.
- Fraud Detection: Anomalies in transaction patterns can quickly flag potential fraudulent activities, adding a layer of security.
Email Receipt Data
Email receipt data represents another rich data source for understanding consumer spending habits. With the rise of online shopping, email receipts have become a digital breadcrumb trail, offering insights into purchase patterns and customer preferences.
Historically, email receipts were mere confirmations of online orders. Yet, their potential as a data source has expanded significantly. By aggregating and analyzing email receipt data, companies can track consumer behavior with remarkable accuracy. They can understand spending patterns across various platforms, identify purchase frequencies, and even discern brand loyalty.
Retailers and e-commerce businesses have leveraged this data type to optimize their customer engagement strategies. They can offer personalized recommendations, tailor promotional offers, and predict future purchase behaviors more effectively.
Technological advancements in data processing and storage have amplified the usefulness of email receipt data. Machine learning algorithms now allow businesses to sift through vast amounts of data efficiently, extracting actionable insights that drive business growth.
Examples of Email Receipt Data Usage
- Customer Loyalty Programs: This data helps identify repeat buyers and subscribers, forming the basis for robust loyalty programs.
- Marketing Campaign Optimization: Insights into purchase patterns support the development of highly targeted marketing efforts.
- Price Sensitivity Analysis: By analyzing purchases and frequency, companies can gauge customer price sensitivity.
- Cross-Selling and Upselling Strategies: Understanding purchase history can lead to more effective cross-sell and upsell opportunities.
- Consumer Sentiment Analysis: Trends in email receipts can help businesses infer consumer sentiments towards certain brands or products.
Economic Data
Economic data offers a macroscopic view of consumer spending trends and patterns, supporting a deeper understanding of how broader economic factors influence individual purchasing behaviors. It provides aggregated information about spending by merchant categories, regions, and consumer segments.
Historically, economic data collected through government reports and industrial surveys was key to understanding consumer spending, albeit often outdated and limited in scope. However, with technological advancements, the accuracy, and timeliness of economic data have improved remarkably.
Industries ranging from retail to banking and beyond utilize economic data to craft strategies that align with consumer trends. This data is invaluable for understanding macroeconomic conditions, such as inflation and employment rates, that directly affect consumer spending.
Economic data's real-time accessibility now aids companies in making quick, informed decisions. With robust data analytics, businesses can pivot their strategies in line with economic changes, ensuring they remain competitive in evolving markets.
Examples of Economic Data Usage
- Market Entry Strategies: Businesses evaluate regional spending patterns to develop effective market entry strategies.
- Investment Analysis: Economic data helps in assessing the economic viability of new projects or investments.
- Risk Management: With insights into economic conditions, businesses can better manage financial risks.
- Supply Chain Optimization: Understanding regional economic conditions helps in optimizing supply chain decisions.
- Macroeconomic Trend Analysis: Companies leverage economic data to track macro trends and align their strategic plans accordingly.
Alternative Data
Alternative data has emerged as a transformative force in understanding consumer transactions and behaviors. Unlike traditional data sources, alternative data encompasses a range of non-conventional sources, including transactions, social media activity, and even satellite imagery.
The rise of big data and analytics has allowed for the collection and aggregation of alternative data at unprecedented scales. This data offers new dimensions into consumer habits that were previously elusive, providing a more holistic view of market dynamics.
Alternative data has been instrumental in influencing decision-making in sectors such as finance, marketing, and retail. Financial analysts use it to predict stock movements, marketers rely on it for sentiment analysis, and retailers dig into it to understand consumer preferences and improve customer experiences.
As the world continues to embrace digital and connected technologies, the scope and application of alternative data are set to grow exponentially, offering ever-richer insights into consumer transaction behavior.
Examples of Alternative Data Usage
- Consumer Segment Profiling: Alternative data aids in developing highly detailed consumer profiles for better personalization.
- Predictive Analytics: This data is key in building models that predict future consumer behavior and market trends.
- Sentiment Analysis: By analyzing social media and online reviews, businesses can gauge consumer sentiment towards products and services.
- Financial Forecasting: Alternative data supports more accurate financial forecasting by considering diverse metrics.
- Innovation Insights: Businesses can discover novel insights and opportunities by tapping into unconventional data perspectives.
Conclusion
In today's data-driven world, access to comprehensive insights into consumer shopping trends is a game-changer for businesses. Transaction data, alongside various categories of data, has become essential in understanding intricate spending patterns and preferences. The speed and precision offered by these data types enable companies to make informed decisions in real-time, steering strategies that align with consumer expectations and market needs.
Organizations increasingly recognize the value of adopting a data-driven approach to business. Transitioning to such a model requires embracing data discovery as a strategic asset, enabling more nuanced decision-making and fostering innovation in product development and customer engagement strategies.
As businesses continue to delve deeper into the monetization of their data, they uncover numerous opportunities to fortify their competitive standing. There is a shift toward recognizing the power of data not just as a resource but as a pivotal component of growth and profitability strategies.
The future promises new trends in data usage, with companies aiming to monetize newly recognized data streams that have been dormant for decades. This foresight is fueling a new wave of data monetization strategies, where businesses plan to offer valuable insights derived from innovative data points.
Looking ahead, the possibilities for new types of data are endless. From exploring IoT-generated consumer data to employing AI-enhanced analysis, organizations are poised to unlock unprecedented insights, driving productivity and relevance in the marketplace.
As new data types emerge, businesses that harness and monetize these insights will lead in shaping the future of commerce, transforming how consumer behaviors are leveraged to deliver value across industries.
Appendix
The insights gleaned from a comprehensive analysis of consumer transaction data are transformative across various industries and job roles. From retail and finance to consultancy and market research, the implications of such data extend far and wide, offering solutions to longstanding industry challenges.
Investors benefit significantly by identifying lucrative market trends and consumer shifts, allowing them to make informed decisions. Data feeds revealing transaction patterns enable them to better forecast financial growth potentials.
Consultants and analysts leverage consumer transaction data to provide clients with detailed market insights and business intelligence. Their ability to analyze spending patterns and consumer segments results in the development of strategies that improve client performance and competitive positioning.
The insurance industry also stands to gain considerable advantages by integrating transaction data into risk assessments, fraud detection, and tailored policy offerings. This enables insurers to offer products more closely aligned with customer needs and risk profiles.
Fortifying these benefits, modern advancements in AI and machine learning promise to unlock even greater value. By processing vast historical documents and contemporary government filings, AI can reveal latent insights, providing companies with information that was previously inaccessible.
The integration of AI with consumer transaction data will revolutionize how industries understand consumer behavior, paving the way for innovative solutions and more engaged customer interactions. The potential is enormous, propelling businesses into a future where data drives not just strategy but competitive advantage and enduring success.