Uncovering Insights with Comprehensive Free-Text Training Data
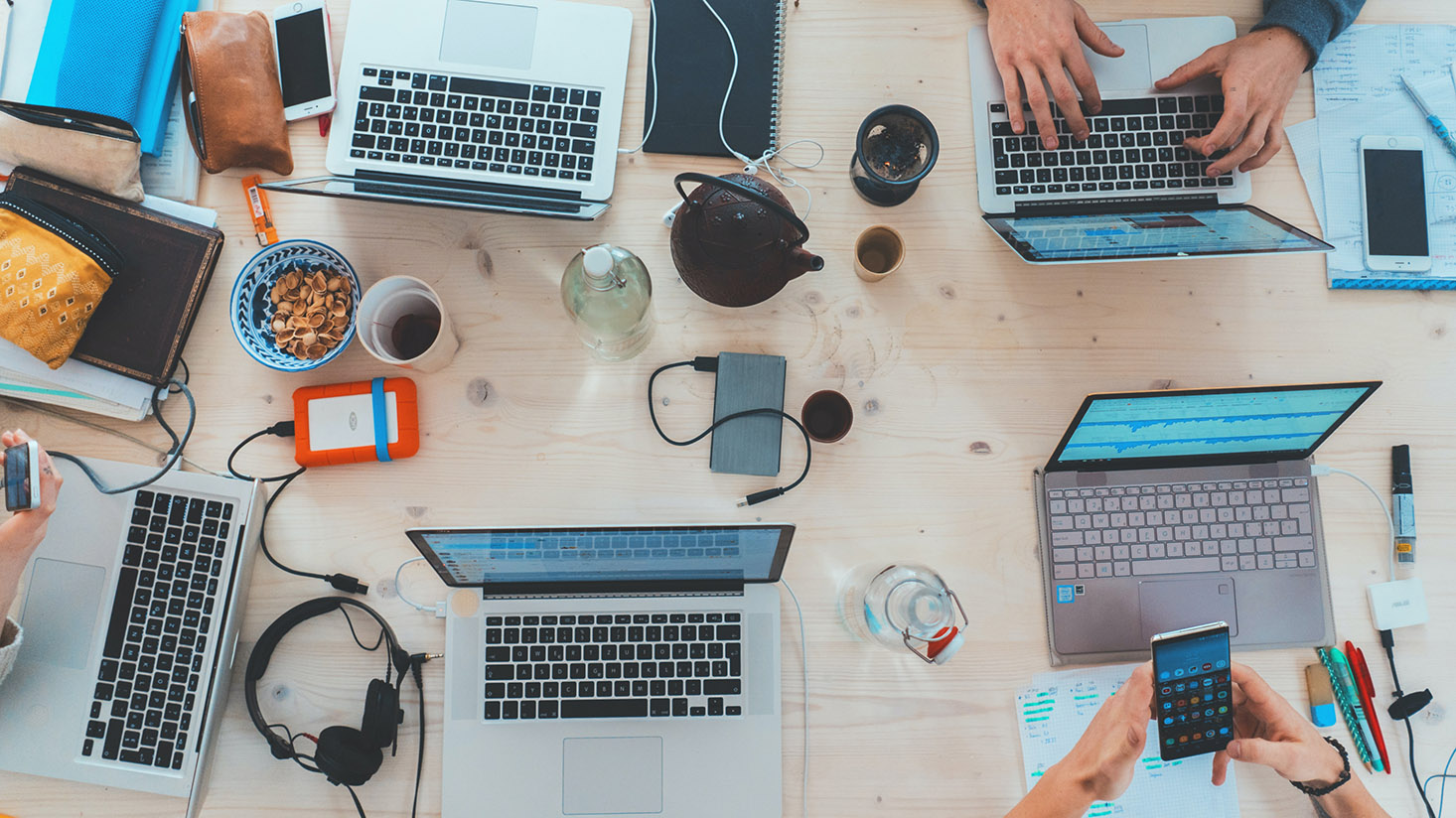
Introduction
In today's digital age, the potential to unlock powerful insights from data is immense. Historically, gaining insights from free-text data sources like customer support calls, medical notes, and legal contracts was challenging. The nuanced nature of such text made it difficult to extract actionable insights using older methodologies. Previously, without robust datasets, businesses relied heavily on direct observation or manual transcription, which often resulted in inaccuracies and delayed decision-making.
Before the advent of digital data acquisition, organizations gathered insights manually. Customer feedback, for instance, was primarily obtained through written or verbal surveys analyzed painstakingly through manual processes. Similarly, medical professionals relied on handwritten patient notes, often scribbled hastily and inconsistently, making it difficult to ensure precise interpretation and documentation.
With the emergence of digital sensors and a continuous connectivity through the internet, organizations began capturing data more seamlessly. The proliferation of databases and data storage solutions allowed for the systematic recording and retrieval of information. This evolution heralded a new era where data became a kingpin in decision-making processes, offering a significant advantage to firms that could harness its power.
In the absence of modern data mechanisms, businesses were in the dark, waiting weeks or months to understand trends or changes. However, today, with advances in data collection and analysis, insights can be garnered in near real time, offering a competitive edge. For instance, Natural Language Processing (NLP) and Machine Learning models can now parse and analyze large text data sets almost instantaneously, extracting meaningful patterns and trends that inform strategic decisions.
The sheer volume and variability of free-text data types have necessitated evolved methods for managing and analyzing data. Whether it's training an entity recognition model with customer service transcripts, analyzing medical notes for research purposes, or interpreting legal documents, the requirement for accurate and expansive datasets has been met with groundbreaking technological solutions. Data-driven methodologies offer unprecedented visibility, illuminating paths that were previously uncharted.
Given the criticality of insights derived from free-text data sources, it is clear that businesses must stay at the forefront of data utilization. Not only does this offer the potential to improve service quality and efficiency, but it also ensures enhanced compliance, better risk management, and, importantly, customer satisfaction. In this exploration, we will delve into different data types that are vital in enhancing insights from free-text data sources.
NLP Data
Natural Language Processing (NLP) data plays a pivotal role in unpacking insights from free-text sources. Historically, NLP was constrained by the computational capacities of the time and limited datasets. Early implementations struggled with context and semantics, resulting in less precise outcomes. However, with advances in computational power and the surge of big data, NLP has inexorably evolved, becoming an inseparable partner in the analysis of text data.
Today's NLP data sets encompass a vast range of domains, from detailed transcriptions of call center dialogues to sophisticated parsing of complex legal texts. The diversity of this data caters to various industries, supporting tasks like sentiment analysis, entity recognition, and topic modeling. This has been particularly transformative for AI research and applications, providing the foundational data necessary for training robust models.
Industries such as telecommunications, healthcare, and legal services have historically leaned heavily on NLP data. Telecom companies parse vast amounts of customer interaction data using NLP to improve customer service, while the healthcare industry uses similar techniques to handle voluminous medical notes, extracting insights critical to patient care and research. Legal services, similarly, utilize NLP to streamline the review of extensive legal documents, drastically reducing overhead and increasing accuracy.
A surge in the volume and complexity of NLP data can be attributed to advancements in cloud computing and storage, enabling organizations to handle larger datasets more efficiently. Furthermore, open-source contributions and collaborative platforms have democratized access, allowing more players to innovate and tailor solutions suited to their unique challenges.
Leveraging NLP data can significantly enhance understanding of free-text data by:
- Extracting Entities: Using NLP to accurately identify and categorize key entities, such as names, dates, and locations, within customer support transcripts or legal documents.
- Sentiment Analysis: Analyzing the tone and sentiment in customer interactions to improve service quality and address concerns proactively.
- Keyword Extraction: Identifying critical keywords and phrases in large bodies of text, aiding in trend analysis and sentiment mapping.
- Contextual Understanding: Analyzing text to understand context, enhancing AI applications in conversational interfaces.
- Topic Modeling: Uncovering themes and patterns across large deposits of text, providing insights into prevalent issues and areas of interest.
AI Training Data
AI training data is instrumental in educating machine learning models to understand and predict outcomes based on text data. The history of AI training data is relatively recent; however, its impact has been profound. In the past, the absence of vast, structured datasets limited the efficacy of machine learning applications, but as data storage became less of a constraint, vast repositories became possible.
AI training data for free-text sources is gathered from diverse pools like customer service interactions, medical notes, and legal agreements. It forms the backbone of machine learning training, providing the examples needed for algorithms to learn and make informed predictions. A crucial aspect of AI training data is its quality and coverage, which directly impacts the efficacy and accuracy of the resulting models.
Businesses across multiple sectors utilize AI training data for different applications, with tech companies being at the forefront due to their computational advantage. Healthcare providers and law firms increasingly rely on AI-powered tools trained with rich data sets to enhance their service offerings, streamline operations, and improve client outcomes.
The burgeoning growth of data has been driven by technological innovation, reducing costs and barriers to collection and analysis. This expanding trove of data not only accelerates AI development but also amplifies the need for more sophisticated training sets that cater to nuanced and evolving requirements.
Utilizing AI training data for free-text data insights includes:
- Enhanced Model Accuracy: Training models on diverse and accurate datasets improves their predictive outcomes.
- Automated Tagging: Automatically labeling sensitive information in legal and medical documents, ensuring privacy while maintaining data utility.
- Contextual Analysis: Developing models capable of understanding complex contextual relationships within textual data.
- Scalable Solutions: Deploying machine learning models that scale to handle large volumes of continuously accumulating data.
- Rich Data Representation: Structuring raw data into formats amenable to machine learning applications, enhancing utility and value.
Legal Data
Legal data encompasses a vast range of documents from court filings to contracts, forming a critical component of free-text data sources. Historically bound by laborious manual review, the legal industry has long grappled with the challenge of processing extensive text data efficiently. This challenge was compounded by regulatory constraints and the inherent complexity of legal language.
The shift towards digitization and technology adoption in the legal industry has been a relatively recent phenomenon, albeit transformative. The availability of large databases of legal documents has democratized access, providing diverse players with the tools to innovate and optimize how legal data is processed and analyzed. This transformation has been fundamental in evolving legal data into a rich resource for training AI models.
Legal firms, insurance companies, and governmental agencies utilize legal data for tasks such as contract review, compliance checks, and litigation support. Enhanced access to legal data has streamlined tedious processes, reduced human error, and improved response time to legal inquiries.
Legal data not only simplifies compliance but also complements various AI use cases. The modernization of this data arena has largely been fueled by increased digitization, regulatory pressures for transparency, and the growing complexity of legal frameworks worldwide.
Legal data can illuminate free-text engagements by:
- Risk Mitigation: Conducting real-time evaluations of legal risks embedded in contracts and agreements.
- Contract Analysis: Providing tools to review and analyze contractual obligations quickly and efficiently, ensuring compliance and risk management.
- Case Law Analysis: Innovating in the field of case law research with automation, saving time and resources for legal professionals.
- Regulatory Compliance: Ensuring businesses stay compliant by identifying and resolving potential legal issues before they escalate.
- Data Privacy: Implementing sophisticated tools for anonymizing sensitive information, upholding legal and ethical standards.
Healthcare Data
Healthcare data, especially clinical notes and medical records, represent another critical avenue for free-text data insights. Historically, healthcare professionals have maintained extensive patient records, but these were often siloed and inaccessible for broader analysis. The medical fraternity dealt with the dual challenge of managing voluminous data and ensuring confidentiality.
The transition to Electronic Health Records (EHR) and more advanced data documentation systems has expanded the utility of healthcare data. Medical notes, previously scattered and inconsistent, are now detailed, coded, and standardized. This shift has enabled researchers and policymakers to glean valuable insights from patient data, enhancing both clinical and operational outcomes.
Each day, hospitals, research institutions, and biotech firms leverage healthcare data for diverse purposes, ranging from patient diagnostics to the evaluation of treatment efficacy. As the volume of healthcare data continues to expand exponentially, its potential to transform patient care and drive medical advancements also grows significantly.
Technological progress has streamlined the collection, processing, and sharing of healthcare data. Consistent innovation in data anonymization and protection tools also means more utility for businesses that wish to leverage this data without compromising patient privacy.
Healthcare data can refine free-text data insights by:
- Clinical Decision Support: Leveraging data to offer diagnostic insights and treatment recommendations.
- Predictive Analytics: Identifying trends and predicting potential health outcomes based on historical data.
- Patient Management: Streamlining patient management processes to improve healthcare delivery.
- Research and Development: Accelerating discoveries through insights into treatment response and patient demographics.
- Data Anonymization: Developing protocols to safeguard patient identities while maintaining data integrity.
Conclusion
In conclusion, leveraging diverse categories of data for insights into free-text applications has revolutionized how businesses and industries operate. From Natural Language Processing to comprehensive legal and healthcare datasets, the dynamics of data have shifted to provide unprecedented transparency and efficiency. Crucially, organizations that embrace these data insights stand to gain significantly in terms of strategic decision-making and operational excellence.
Data-driven decision-making is no longer a luxury but a necessity. As businesses strive to become more data driven, access to diverse datasets is central to their analytical prowess and future success. Whether streamlining customer service functions, optimizing patient care, or refining legal processes, diverse data types offer innumerable benefits in understanding complex text insights.
As organizations increasingly look to data monetization, the pool of available data is foreseen to increase, fostering innovation and competitiveness. As a result, businesses should anticipate new data types emerging that will unlock even deeper insights, shaping the future's data landscape even further.
The evolving role of AI and machine learning, fueled by extensive and diverse datasets, is poised to redefine industries. The potential to unlock value from existing archives and build predictive, intelligent systems cannot be understated, ensuring businesses remain at the cutting edge of progress.
Looking ahead, it's likely that even more advanced use cases and external data integration opportunities will emerge, pushing the boundaries of data utilization. It will be exciting to see how future datasets impact the ability to extract increasingly complex insights from free-text data. Organizations that harness these opportunities stand to lead in innovation and foresight.
The race for digital transformation continues to accelerate, reinforcing the importance of data discovery efforts. As businesses explore further into uncharted data territories, the opportunities are boundless, and the prospects for enhancing their strategic capabilities remain profound.
Appendix
Various sectors and roles stand to benefit enormously from diverse data insights derived from free-text training data. Investors, consultants, market researchers, and policy developers can gain a better understanding of the nuanced layers embedded in this data type, enhancing both decision-making and strategy development.
Healthcare providers can leverage data insights to improve patient care and streamline processes. A wealth of healthcare data transformed how professionals diagnose, treat, and manage patients while supporting research innovations. As AI continues to unlock data insights efficiently, data-driven healthcare objectives can be achieved faster and more comprehensively.
Legal professionals rely on free-text insights to navigate complex legal frameworks and regulations more effectively. Automating document review and contract analysis, leveraging datasets like case law archives, helps mitigate risks and ensure compliance, thereby transforming the legal landscape.
Insurance companies can utilize free-text data to identify patterns in claims, providing a deeper understanding of client needs and improving risk assessment. Analyzing call center data with NLP can significantly enhance customer interactions and help streamline processes, delivering value across the insurance sector.
Looking to the future, the role of AI in unlocking hidden value from texts is bound to increase. Machine learning algorithms can parse data, unearth trends, and generate insights at phenomenal scales. Foreseeable advancements in AI and NLP will bring stronger analytical capabilities, further speeding the extraction of insights from vast data archives.
In conclusion, transformative data insights derived from comprehensive free-text data sources are shaping business strategies and redefining industry dynamics. As the demand for such data continues to grow, innovative applications across sectors will fast-track the integration of emerging technologies into business processes, ensuring a data-driven future.