Understanding Consumer Behavior with US Grocery Orders Data
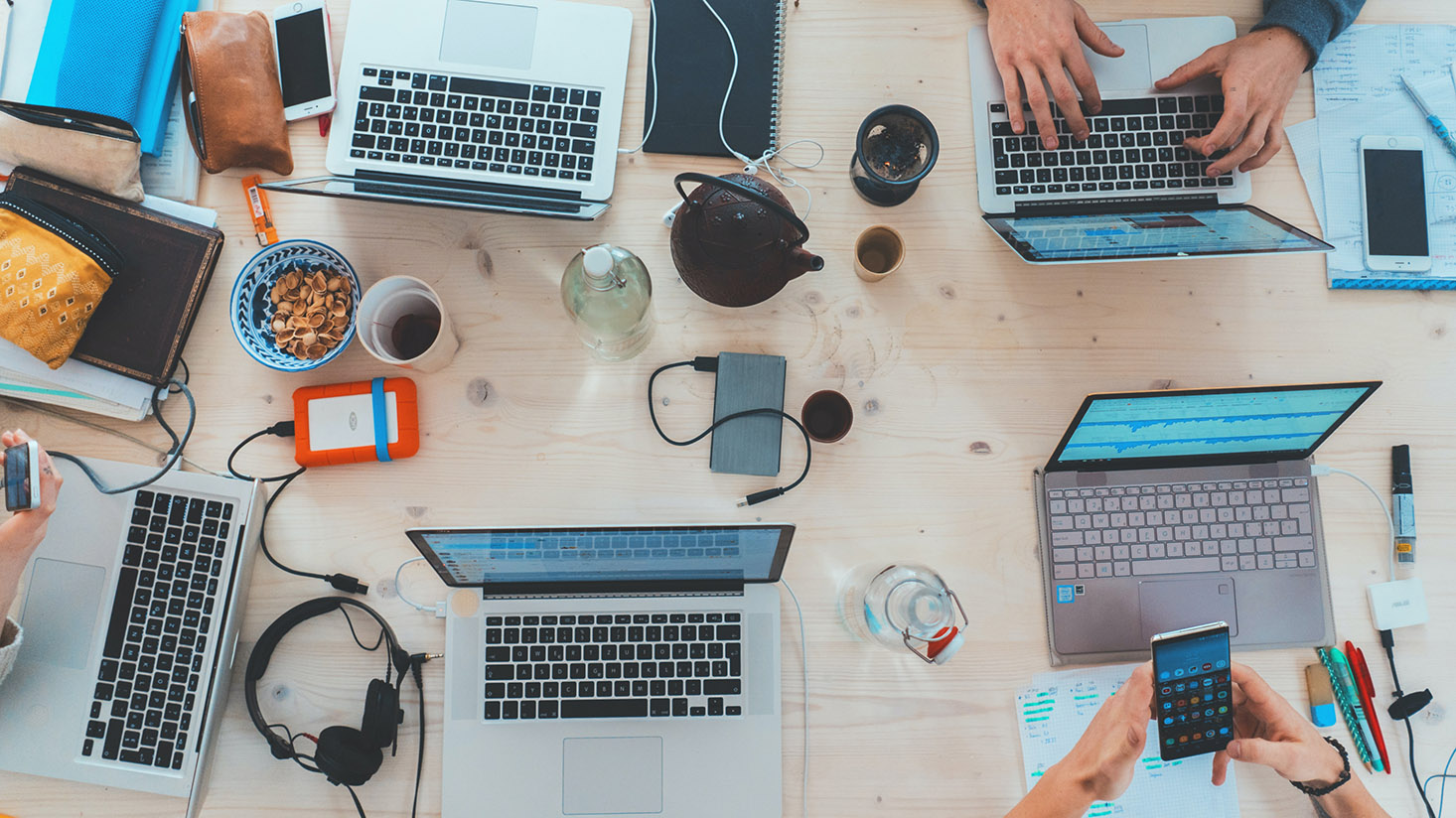
Introduction
In today's rapidly changing consumer landscape, understanding the nuances of grocery orders data, specifically related to plant-based milk products in the US, has become crucial for businesses seeking to optimize their strategies. Historically, gathering insights into consumer behavior and preferences was a daunting task. Before the age of technological advancement and data proliferation, businesses relied heavily on rudimentary methods to gain insights into consumption patterns. This included physical surveys, general sales reports, or even anecdotal evidence gathered from retail staff, which were often unreliable and lacked granularity.
Before the era of big data and fully integrated data systems, such insights were mostly inferred through indirect means. Companies relied on public sales figures, general economic indicators, or trends in similar product categories. This approach left stakeholders navigating in the dark, often making strategic decisions based on partial or outdated information. An accurate pulse on the market was often weeks or months out of reach.
The advent of the internet, connected devices, and advanced sensors marked a new dawn. These technologies facilitated the digitalization and storage of nearly every transaction and interaction. Suddenly, data became not just an artifact of operational systems but a strategic asset. The digital trail left by consumers provided a goldmine of insights, far richer and more immediate than ever before.
With the proliferation of smart technologies and software solutions in every business process, data capture and storage became an integral part of operations. This was especially evident in the retail sector, where point-of-sale systems evolved from simple cash registers to complex, data-rich systems capable of capturing detailed information about each transaction.
Today, leveraging high-quality data in real-time allows companies to respond proactively to market shifts. This capability has been further enhanced by platforms like Nomad Data, enabling businesses to access categories of data they may never have deemed possible.
Data-driven decision-making has become synonymous with success, providing a competitive advantage in a world where consumer preferences can shift overnight. As we delve deeper into how specific types of data can illuminate the complexities of grocery data, particularly focusing on the plant-based milk market, it becomes clear how indispensable these modern data tools are.
Point of Sale Data
Point of sale (POS) data represents one of the most foundational datasets in retail analytics, capturing the detailed information generated at the point of purchase. This type of data has been an essential component for understanding consumer behavior across various industries.
Historically, retail industries relied on basic cash registers, offering minimal opportunity to gather extensive transactional data. As technology evolved, so did the capacity for POS systems to provide insightful data points. POS data from systems today offers granularity down to SKU-level, capturing not just the product sold but its price, quantity, and discount details, among others.
Industries that have primarily benefited from this type of data have included retail, particularly grocery and convenience stores, which frequently analyze such data to optimize inventory and sales strategies. However, it is not limited to this sector alone. The hospitality industry, fashion retail, and even event ticketing rely on POS data to better understand customer preferences and transactional history.
The technological advances that brought POS data to the forefront were predominantly the introduction of digital transaction systems and automated checkout stations, allowing for real-time data aggregation across multiple retail locations.
Using POS data for our primary topic, grocery orders of plant-based milk products can result in significant insights. This data can track sales of brands like Oatly, Silk, and Califia Farms, providing information on how these items perform both regionally and nationally.
- Identify popular brands: Understand which plant-based milk brands are leading in sales across different segments and geographical regions.
- Promotional impact analysis: Assess how sales promotions and discounts affect the purchase volume of plant-based milk products.
- Trend spotting: Observe seasonal trends in plant-based milk consumption, helping foresee future demand.
- Inventory management: Use sales data to manage stock levels effectively, reducing the risk of overstock or stockouts.
- Price sensitivity: Analyze how price changes influence consumer purchasing behavior and brand loyalty.
With platforms like Nomad Data, companies can enhance their insights, ensuring they stay ahead of market dynamics.
Inventory and Purchasing Data
Inventory and purchasing data serves as a vital source for managing product availability and understanding purchasing trends across retail environments. This dataset provides critical insights into how inventory levels correlate with sales, thus influencing strategies for product replenishment and distribution logistics.
In earlier times, inventory management was largely based on historical sales data and manual tracking, often leading to inaccuracies and delays. However, with the digital revolution, obtaining real-time updates on stock levels and purchasing behaviors became possible. Electronic platforms and IoT devices within supply chains have played a significant role in making inventory data dynamic and highly actionable.
The retail sector, particularly grocery and food services, has been a significant adopter of inventory data. The necessity to maintain a balance between demand and supply while minimizing waste highlights the importance of this dataset. Moreover, manufacturers, warehouse logistics, and supply chain consultants rely on this data for efficient operations.
Significant technological advancements that have driven the importance of inventory data include RFID technology for tracking goods, advanced analytics for predicting stock needs, and AI-based solutions for demand forecasting.
When tied to plant-based milk products, inventory and purchasing data can provide valuable insights into the consumption patterns and supply chain efficiency. For instance, brands can understand the demand frequency and purchasing preferences.
- Demand forecasting: Predict future inventory needs based on historical sales patterns and seasonality.
- Product availability: Ensure that plant-based milk products are available when and where consumers desire them.
- Regional distribution: Analyze which geographic areas have higher consumption rates and adjust distribution accordingly.
- Supply chain optimization: Use purchasing data to streamline logistics and reduce transportation costs.
- Waste reduction: Align purchasing and stocking with actual demand to minimize product expirations and spoilage.
Inventory management combined with actionable purchasing data unlocks substantial competitive advantages in a crowded market.
Transaction Data
Transaction data encompasses detailed records of each financial transaction conducted, laying a foundation for insights into consumer spending patterns and purchase behaviors. Historically, transaction data was limited to basic financial records, but with the rise of digital payments, this dataset has become incredibly robust, offering a deep dive into consumer preferences.
Traditionally captured through financial systems, transaction data was predominantly used by the banking and financial services industry. However, its utility extends across multiple domains, including retail, where it assists in understanding customer life cycles and enhancing personalization strategies.
Technological innovations, particularly in digital payment methods, have transformed how transaction data is captured and analyzed. The integration of mobile payments, cryptocurrency, and blockchain technology has further enriched this dataset's granularity.
When applied to plant-based milk products, transaction data can reveal valuable insights about consumer engagement, loyalty, and price sensitivity. For example, transaction data enables the tracking of purchases across various channels, including traditional stores and online platforms.
- Customer loyalty: Identify repeat purchasers and monitor brand loyalty over time.
- Channel analysis: Understand consumer preferences for buying plant-based milk online versus in-store.
- Marketing effectiveness: Evaluate the success of marketing campaigns in driving sales conversions.
- Price elasticity: Analyze how different pricing strategies impact consumer purchasing decisions.
- Cross-selling opportunities: Discover purchasing correlations to suggest complementary products along with plant-based milk.
Incorporating transaction data into business analytics equips companies with the tools needed for a data-driven approach to understanding and catering to consumer needs.
Conclusion
The insights generated from analyzing grocery orders data within the US are extremely valuable for understanding consumer habits, especially concerning plant-based milk products. These insights empower businesses to create data-driven strategies, fostering more informed decision-making processes.
Retailers and manufacturers now have access to comprehensive and real-time data, allowing them to respond rapidly to changing market trends. The agility afforded by such data ensures that businesses stay relevant and competitive.
As we embrace the era of artificial intelligence and machine learning, the predictive power of these datasets will only intensify. Organizations must pivot to become more data-centric, employing sophisticated analytics to unlock the potential held within vast datasets.
The growing emphasis on data monetization presents an opportunity for businesses to leverage consumer insights for revenue. As companies increasingly seek to discover training data for AI models, unique datasets could provide an edge in understanding consumer trends.
Future opportunities are boundless. Companies might explore new datasets such as sentiment analysis from social media, consumer feedback loops, or even nutritional trend datasets to refine their market understanding.
Businesses stand on the brink of a data-driven revolution where external data can transform operations, sharpen competitive strategies, and uncover unprecedented consumer insights.
Appendix
The roles and industries poised to benefit significantly from grocery order data analytics are diverse. Within the investment community, analysts use such data to gauge the performance of consumer goods firms, guiding investment strategies in the food and retail sectors.
Consultants utilize these data insights to offer targeted recommendations to their clients, enhancing business operations and optimizing supply chains. Management consultants, in particular, leverage this data to craft solutions that improve profitability and efficiency.
Market researchers benefit by gaining a granular understanding of consumer behavior, helping them in the creation of tailored marketing campaigns. This level of insight often translates to heightened engagement and customer satisfaction, expanding brand reach and loyalty.
The insurance industry can also harness grocery data analytics, using spending patterns as predictors of health trends and risks. This allows for more accurate policy pricing and underwriting decisions.
As the future unfolds, the integration of AI to unlock historical and real-time data will be fundamental. AI can uncover insights from decades-old documents and even modern government filings, suggesting anomalies and deriving actionable intelligence.
The continued evolution of this data landscape ensures that those who adopt data-driven approaches will gain significant advantages, becoming leaders in their respective industries.