Understanding Loan Yield in Key Chinese Cities with Financial Data
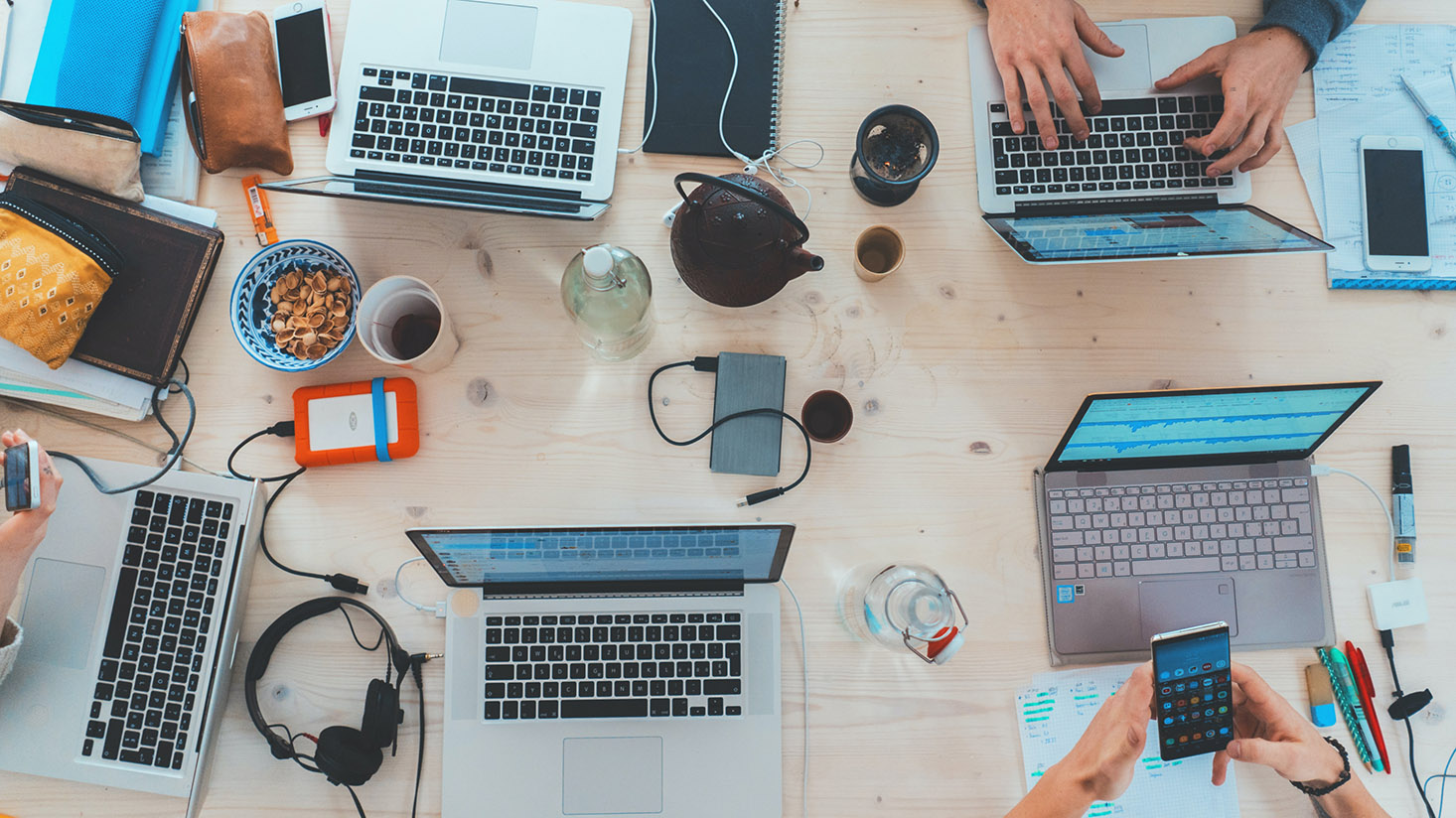
Introduction
The quest to understand loan yield data in China has been a challenging endeavor for business professionals and economists alike. Historically, gaining insights into this area involved a cumbersome process rife with manual collection methods, anecdotal evidence, and delayed reporting. The days before the proliferation of digital data were marked by reliance on documents, paperwork, and sometimes word-of-mouth, which were neither efficient nor timely.
Loan yield metrics, such as average mortgage or consumer finance loan yields, have always been of keen interest because they offer a glimpse into borrowing and lending dynamics. Before the digital age, firms depended on monthly or quarterly financial statements without the granularity needed for deep analysis. Such insights were more retrospective than predictive, often leaving professionals in the dark.
With the advent of modern technology, such as external data search systems and interconnected devices, obtaining precise and real-time loan yield data has become more streamlined. The digital revolution, spurred by the internet and big data analytics, allows for comprehensive datasets that not only reflect historical averages but also predict future trends.
The integration of sensors and connected devices in financial systems has also boosted data availability. Consider how mortgage applications are now processed online with real-time loan yield calculations, providing immediate feedback and helping stakeholders make informed decisions faster.
Today, businesses can leverage various data types to interpret shifts in loan yields accurately. This shift from outdated methods to modern data-driven approaches lets professionals respond to market changes almost instantaneously. Loan yield data, which used to take weeks to gather and analyze, can now be accessed with a few clicks, thanks to sophisticated financial datasets.
In this rapidly evolving landscape, understanding loan yield data is crucial for fostering economic growth, making intelligent investment decisions, and crafting effective financial strategies. The transformation from traditional processes to data-centric methodologies highlights the importance of data in comprehending the complexities of China's loan markets.
Mortgage Loan Data
Mortgage loan data has long been essential for understanding financial trends within China. Historically, data in this domain was manually gathered through records kept at local banks or government offices. Mortgage data would often trickle in from surveys or depersonalized monthly reports, which lacked the breadth and depth desired for strategic planning.
Over the years, this data type has evolved significantly with the penetration of advanced financial software that collects and analyzes vast quantities of mortgage-related information. Today, mortgage data is a robust and dynamic dataset that provides valuable insights into average yields, interest rates, and even borrower demographics in cities like Ningbo, Hangzhou, and Shanghai.
Historically, stakeholders such as financial analysts, real estate professionals, and government bodies heavily relied on mortgage data to guide their decisions. However, it wasn’t until the digitization of records that a real breakthrough occurred, allowing users to access real-time insights across various metropolitan areas.
The advancement of technology has transformed mortgage data from static historical records into real-time insights that empower better decision-making. This transformation is driven by financial platforms and databases that aggregate city-level data to reveal patterns and irregularities across regions.
Yet, the significance of mortgage loan data goes beyond just monitoring average yields. Here are some specific examples of its utility:
- Forecasting market trends: By analyzing historical yield data, businesses can predict future market movements.
- Risk assessment: Identifying credit risks associated with particular demographic groups or regions.
- Investment decisions: Informing real estate investments by understanding potential appreciation rates.
- Policy formation: Assisting governmental agencies in creating policies that regulate the housing market.
- Competitive benchmarking: Offering comparative insights to financial institutions to refine their offerings.
Meteoric advances in digitization continue to enhance the accuracy and applicability of mortgage data, making it a critical tool for various sectors aiming to optimize their strategies in China’s dynamic loan market.
SME Loan Data
Small and Medium Enterprise (SME) loan data serves as a critical gauge of economic health and entrepreneurial activity within a region. Historically, gathering comprehensive SME loan data was a daunting task, primarily because it entailed sifting through paper-based records from individual banks.
Today, however, SME loan data has transformed into an expansive dataset capturing an array of metrics, thanks to coordinated efforts in financial digitization. The proliferation of digital banking platforms has made it possible to gather data on SME loan yields as frequently as quarterly, providing up-to-date insights on business activity in cities such as Beijing, Nanjing, and Jiangsu.
Industries that have historically benefited from SME loan data include financial analytics companies, governmental economic bodies, and business incubators. By understanding where and how SMEs access funding, stakeholders can make informed predictions about regional economic growth and identify emerging business opportunities.
The expansion of SME loan data capabilities is enabled by digital financial tools that conduct real-time analyses on loans, offering instant assessments of enterprise health and lending trends. Below are various ways in which SME loan data can provide better insights into financial markets:
- Understanding regional business climates: Detailed loan data allows analysts to assess the economic vitality of specific cities or provinces.
- Monitoring creditworthiness: Evaluating the risk levels associated with lending to different industries or business sizes.
- Optimizing financial services: Fine-tuning financial products to meet the needs of local SMEs.
- Policy development: Informing public policy decisions to foster a supportive environment for SMEs.
- Investment diversification: Guiding portfolio managers interested in SME-oriented investment opportunities.
As data collection mechanisms become increasingly precise, SME loan data will continue to grow in importance, offering crucial insights that enable more strategic and proactive decision-making in the wider financial landscape.
Consumer Finance Loan Data
Consumer finance loan data plays a pivotal role in understanding personal borrowing behaviors and consumer confidence across China. Before the digital age, acquiring consumer loan data meant manually processing complex paperwork, often leading to delayed and incomplete insights.
With advancements in external data platforms, consumer loan data has evolved into a comprehensive, timely, and actionable asset. It provides financial experts a clear snapshot of consumer lending, average borrowing costs, and payment behaviors among urban populations.
Industries such as retail banking, consumer credit firms, and market research organizations have long depended on consumer finance loan data. However, the technology advancements that record every consumer interaction with financial institutions have vastly improved the accuracy and scope of this dataset.
Here are some ways consumer finance loan data can be leveraged for additional insights:
- Predictive analytics: Using historical loan data trends to project future consumer borrowing behaviors.
- Credit scoring enhancements: Refining algorithms that determine consumer creditworthiness.
- Product development: Creating tailored financial products based on current consumer needs and spending patterns.
- Market segmentation: Identifying high-value consumer segments and opportunities for cross-selling.
- Behavioral studies: Understanding the drivers behind consumer borrowing decisions.
The ongoing digitization of consumer finance data not only benefits financial service providers but also provides essential insights for crafting well-targeted marketing strategies and improving customer engagement.
Conclusion
The exploration of various types of financial data illuminates the critical role they play in understanding loan yield dynamics across China's major cities. With access to mortgage, SME, and consumer finance data, professionals are better equipped to navigate the financial landscape with confidence.
Data reliance has become integral to effective decision-making, offering insights that were previously inaccessible through traditional methods. It has become clear that integrating diverse types of datasets is not just beneficial but essential for future-proofing corporate strategies.
As a result, organizations are gradually incorporating a data-driven approach, catalyzing the need for advanced data analytics in evaluating market trends and insights. Many companies are also exploring ways to monetize their data or gain supplementary insights through outside partnerships.
Looking ahead, the nature of financial data itself is set to evolve. Imagining new forms of data—such as real-time economic metrics or consumer sentiment analysis—could unlock further potential and redefine how stakeholders engage with loan yield patterns.
Optimizing data collection and analysis strategies will undoubtedly remain a priority, corroborated by advancements in digital technology, AI, and machine learning. The financial world stands on the brink of exciting opportunities, guided by the insights that innovative and expansive datasets will continue to provide.
Appendix: Key Roles and Industries Benefiting from Financial Data
In the realm of finance, data-driven insights have transformed industries, paving the way for unprecedented growth and innovation. Several roles and sectors benefit significantly from the availability of detailed loan yield data. Among these, investors, market researchers, and financial consultants stand out.
For investors, loan yield data in Chinese cities presents a key area of interest. Investors seek to analyze this data not only to steer investment strategies but also to manage portfolio risk effectively. The ability to understand regional loan yield variations can serve as a crucial factor in asset allocation decisions.
Consultants leverage this data to advise clients on financial strategy and operational optimization, while market researchers utilize it to comprehend economic indicators and sector health. Together, this knowledge drives new investment avenues and boosts client confidence.
Insurance companies use detailed financial datasets to assess risk accurately, devising tailored policy structures that align with market conditions. As such, access to complete and timely data can reveal weaknesses in existing models or highlight new areas for development.
In the future, AI might further unlock hidden patterns in existing data, uncovering predictive insights from longstanding trends or even providing clarity in complex government financial statements.
The integration of machine learning with financial datasets will accelerate the ability to identify opportunities while automating routine analyses. By engaging in the discovery of rich and varied training datasets, such as those discussed in training data, businesses can refine their predictive models, staying competitive in a data-driven world.