Unlock Consumer Segment Insights with POS Transaction Data
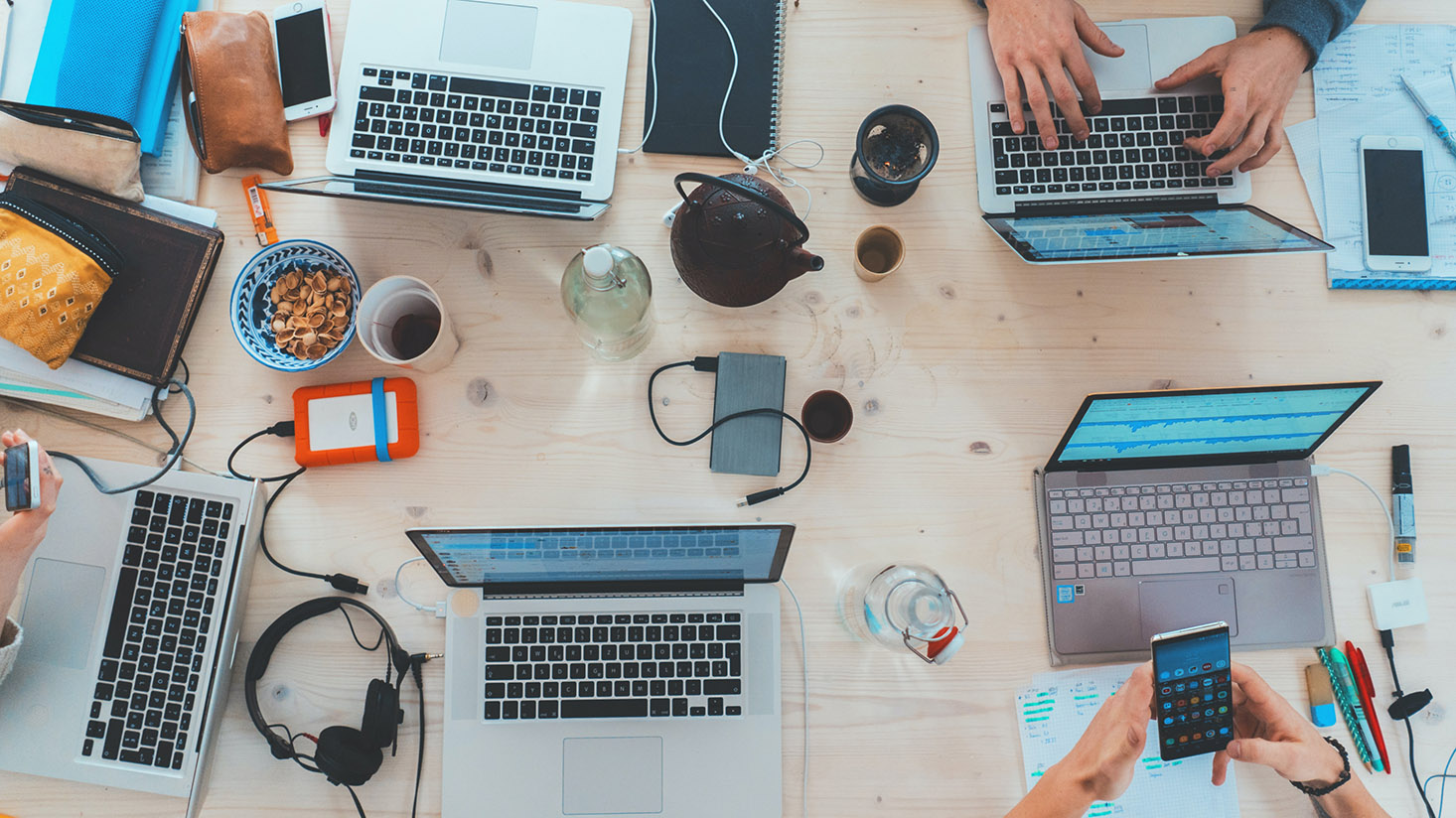
Introduction
The world of consumer segments, especially in the retail arena, has always been a complex labyrinth. Historically, gaining insights into these segments involved cumbersome, manual methods to gather and analyze data. Retailers and brands relied on consumer surveys, foot traffic analysis, and basic sales reporting—tools that were not only time-consuming but also limited in breadth and depth. It is fascinating to consider how businesses operated before the age of digital transformation, often with very little data to guide strategic decisions.
Before the advent of comprehensive external data sources, retailers might have relied on rudimentary data such as sales logs, occasional market research, and gut instinct to drive their strategies. Decision-making processes were slow, sometimes taking weeks or even months to adapt to market changes or consumer behaviors.
The dawn of the internet and connected devices ushered in a new era for data collection. Sensors embedded in point-of-sale systems, the proliferation of eCommerce, and comprehensive transaction tracking have revolutionized the way businesses understand consumer behavior. The rise of data-driven technologies allows companies to capture every transactional detail across platforms, whether brick-and-mortar or online.
Such transformation is vastly significant as it allows businesses to transition from reactive to proactive decision-making. Instead of being in the dark, waiting weeks to grasp changes in consumer behavior, companies can now receive real-time insights. This agility is invaluable in today's fast-paced market where consumer preferences can shift unexpectedly.
The importance of data in understanding consumer segments cannot be overstated. With the right data, organizations can discern patterns, understand purchasing trends, and even forecast future demands with remarkable accuracy. The ability to access granule data at a weekly product-retailer level with various metrics has bridged the gap from traditional broad-stroke strategies to finely-tuned, data-informed decision-making.
In this article, we delve into various categories of data that can provide insights into consumer segments through point-of-sale transactions. We will explore the roles of Financial Data and Transaction Data in unearthing these insights for different consumer segments like food & beverage, electronics, clothing, and more.
Financial Data
The concept of financial data has evolved dramatically over the years, and its role in understanding consumer segments has become increasingly pivotal. Historically, financial data was primarily used for analyzing broader economic trends and financial performance. Early adopters of financial data were often limited to large financial institutions and government agencies who used economic indicators and aggregated financial metrics to inform policy and investment decisions.
With advancements in technology, financial data has become much more detailed and accessible. Today, this type of data encompasses a diverse range of information, including SKU-level point-of-sale (POS) data from thousands of retailers. This granular level of data collection has enabled businesses across industries to understand consumer behavior in unprecedented detail.
One of the most significant technological advances in financial data collection has been the integration of POS systems in retail environments. These systems capture transaction data in real-time, providing comprehensive insights into consumer buying habits. Retailers, as well as other sectors such as consumer goods and service industries, have increasingly adopted these systems to gain a competitive advantage.
The acceleration of financial data volume is largely driven by the digitalization of retail and the growing number of connected devices. As each transaction is recorded, an expansive dataset is created, capturing detailed information on every sale, including product type, location, time, and even consumer demographics associated with loyalty programs.
Understanding consumer segments, particularly within different geographic markets such as Japan, has been greatly enhanced by financial data. The ability to access SKU-level data from hundreds of convenience stores and supermarkets offers invaluable insights into local consumer preferences and trends.
Five ways financial data can be utilized to gain insights into consumer segments include:
- Trend Analysis: Track changes in consumer preferences over time by analyzing historical sales data at the SKU level.
- Market Basket Analysis: Understand buying patterns by analyzing which products are commonly purchased together.
- Price Sensitivity: Evaluate consumer response to pricing changes and optimize pricing strategies accordingly.
- Regional Preferences: Compare sales data across different regions to identify local consumer trends.
- Customer Loyalty Programs: Leverage data from loyalty programs to identify and reward frequent shoppers, enhancing customer retention.
Transaction Data
Transaction data represents one of the most dynamic sources of consumer insights today. Initially, retailers relied on transactional data fundamentally for accounting and basic sales reporting. However, transaction data’s evolution has been remarkable, offering a more profound understanding of consumer segments across various industries.
Transaction data aggregates all consumer purchase activities, whether through physical receipts or e-receipts, capturing a comprehensive view of purchasing patterns. Over time, industries such as consumer electronics, home improvement, and clothing have turned to transaction data to identify specific consumer demands and preferences.
The technological advancements that have bolstered transaction data collection include the digitization of receipts and the use of machine learning algorithms to process and analyze vast amounts of data. This transformation allows for not just understanding the transaction value but dissecting each element of a purchase.
With the amount of transaction data available consistently accelerating, businesses can now offer personalized shopping experiences, tailoring their operations to meet nuanced consumer needs. Retailers, equipped with demographic data linked to transactions, can segment their consumer base based on behavior and preferences with precision.
The use of transaction data goes beyond traditional sales analytics. It provides businesses with the capability to explore consumer insights and drive strategic decisions effectively. By differentiating between physical and e-receipts, companies can optimize their online and offline strategies.
Five practical examples of how transaction data can be applied to understand consumer segments include:
- Consumer Behavior Segmentation: Categorize consumers based on their purchasing frequencies, preferences, and channels.
- Channel Performance: Differentiate between in-store and online purchase patterns to optimize resource allocation across channels.
- Product Lifecycle Analysis: Monitor product performance from launch to decline phase for strategic inventory and marketing adjustments.
- Predictive Consumer Insights: Use historical transaction data to forecast future demands and trends.
- Cross-Category Sales Monitoring: Explore how sales of one category might affect sales in another, leading to improved cross-selling strategies.
Conclusion
In summary, the insightful understanding of consumer segments hinges heavily on the strategic utilization of various data types, particularly financial and transaction data. These datasets provide critical insights into consumer behavior, facilitating informed decision-making processes for businesses globally. The ability to analyze consumer data at a granular level has transformed how companies operate, allowing for dynamic, evidence-based strategies.
As organizations embrace a more data-driven approach, integrating diverse data sources becomes indispensable. The emergence of platforms facilitating data monetization empowers companies to leverage untapped data assets, unlocking new revenue streams while enriching decision-support systems.
The evolving landscape suggests an imminent rise in the availability and types of data assets, further enhancing consumer insights. Companies are expected to innovative around emerging data types, offering more refined datasets that could extend beyond basic transactional information, encompassing broader consumer behaviors and lifestyle patterns.
Businesses must remain vigilant in adopting these changes, ensuring they possess the agility to adapt to rapidly evolving markets. The potential to enhance strategic value by coupling traditional and modern data resources cannot be overlooked.
Looking forward, the role of AI and analytics continues to play a predominant role in unlocking hidden value within abundant data reserves. The advancement in data technology and solutions will further engrain these practices into the core framework of organizations globally.
Ultimately, the future holds vast possibilities as new technologies and data accessibility converges. Industries that successfully adopt these advanced strategies will not only unlock greater consumer insights but also position themselves as leaders within an ever-competitive business environment.
Appendix
The rapid evolution of data collection and analytics has had a transformative impact on numerous roles and industries. Investors, consultants, insurance companies, market researchers, and a host of other professionals have seen significant benefits from harnessing the power of POS transaction data to glean insights into consumer segments.
Investors, for example, have relied on such data to predict market trends and make informed decisions regarding stock purchases in retail and consumer goods sectors. With the capability to track real-time sales and consumer patterns, investors are better equipped to assess a company's market position and growth potential.
Consultants leverage detailed consumer insights to advise retail and brand clients on optimizing product offerings and marketing strategies. By understanding demographics and purchasing behaviors, consultants can tailor strategies to match consumer preferences more closely.
Insurance companies utilize this valuable data to enhance their risk models, assessing consumer buying patterns as proxy indicators for risk prediction in various sectors. Such developments in data analytics have significantly improved the accuracy of risk assessments.
Market researchers are perhaps the most obvious beneficiaries, using transaction data to study consumer behavior nuances, identifying emerging trends, and developing comprehensive market reports. As training data becomes more available, researchers can build more sophisticated predictive models.
Looking to the future, AI stands poised to delve even deeper into transaction data, uncovering insights from decades-old documents and modern, large-scale datasets alike. AI’s potential to translate massive data points into actionable insights will revolutionize how industry professionals value and apply historical data, propelling the sector further into the future.