Unlock Health Insights with Activity and Sleep Data for Life Insurance
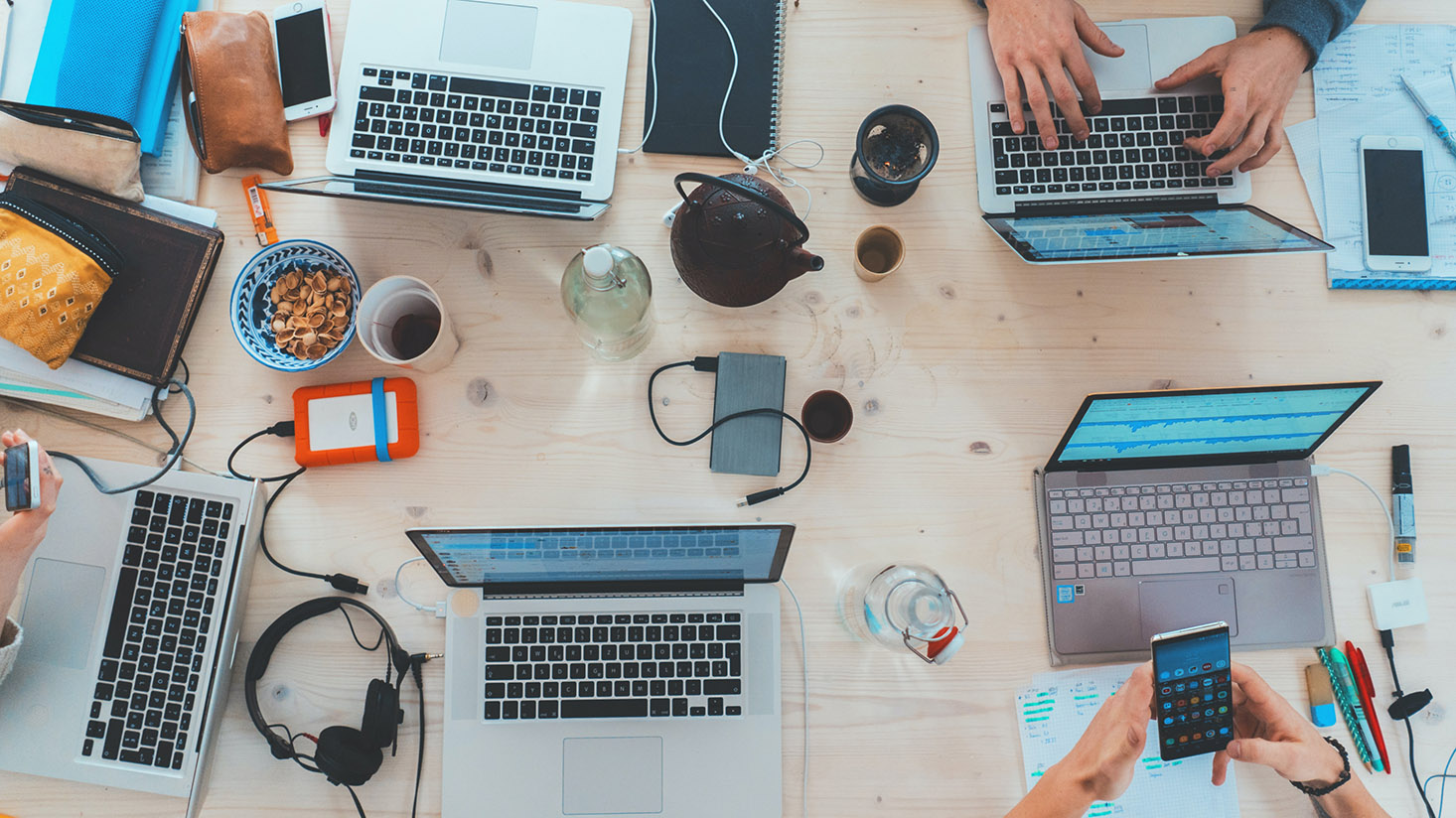
In today's data-driven world, comprehending human behavior is a puzzle that businesses and institutions strive to solve. One such puzzle is understanding the intricate relationship between physical activity levels, sleep patterns, and mortality rates among individuals. Historically, gleaning insights into this area has been challenging, with reliance primarily placed on anecdotal evidence or limited empirical data. Before the digital age, insurance companies and health researchers gathered rudimentary physical activity data through labor-intensive methods like surveys, self-reported logs, or indirect assessments.
For instance, individuals may recall that early research relied on paper-based surveys to track daily steps or activity levels. These surveys were often constrained by participant bias and memory lapses, as well as the inefficiency of manual data processing. Self-reported logs, while being somewhat of an improvement, still suffered from an inherent subjectivity and often made data accuracy questionable. Before sensors and connectivity, human observation was pivotal. Researchers painstakingly conducted studies with limited participants, often generating data that was not immediately actionable.
The surge of advancements in technology, specifically within the realms of sensors, the internet, and connected devices such as smartphones and wearable technology, has revolutionized how we gather and analyze data related to physical activity and health outcomes. The rise of these innovations ushered in an era of real-time data collection. Devices are now capable of continuously tracking movements, sleep patterns, and activity levels, providing a wealth of data that traditional methods could hardly replicate.
For businesses like those in the life insurance sector, these technological developments have turned the once-labyrinthine task of understanding mortality risk into a more understandable science. Granular data, available at the individual level or in aggregate, enables insurers to refine their risk models and pricing strategies significantly. The result is that organizations can transition from generalized assumptions to data-backed insights that directly inform decision-making and product offerings.
Modern datasets allow insurers to understand real-time changes in physical activity and health indicators, enabling a shift from reactive to proactive engagement with policyholders. No longer do businesses need to rely on quarterly or annual reports to identify trends; instead, they can anticipate needs and adjust their strategies dynamically.
Data holds the key to dissolving the mystery surrounding physical activity and its correlation to mortality. Furthermore, as highlighted in the importance of data in AI, leveraging artificial intelligence can transcend traditional analysis, thereby offering a nuanced understanding that guides better-informed decisions.
Geolocation Data
Geolocation data provides a compelling lens through which we can analyze human movement patterns and behaviors. Through the ubiquity of cellphones, this type of data captures the movement of individuals worldwide, offering a macro perspective traditionally inaccessible. Historically, location-based analyses were limited to manual tracking and point-in-time observations, yet today geolocation data reveals patterns on a micro scale, leading to more informed assessments than previously possible.
For the life insurance industry, geolocation insights are invaluable. For instance, they provide a dynamic understanding of how physical activity varies across different regions or demographics. This data type is beneficial across various roles and industries like financial, real estate, and insurance, offering benefits that include measuring community exercise levels, evaluating walkability of urban areas, and estimating local health risks.
The technological advances responsible for geolocation data's rise largely stem from advancements in mobile technology, GPS accuracy, and data processing, enabling unprecedented data collection and analysis. Such innovations have led to a soaring availability of movement data, transforming conventional understandings into continuous streams of fresh insights.
The various ways geolocation data can be applied to examining physical activity patterns and mortality risk include:
- Community Activity Mapping: Determining regions with high or low average daily step counts can offer predictive insights into community-wide health risks.
- Exercise Habits: Analyzing time and frequency of visits to gyms or parks enables better understanding of population health habits.
- Correlation with Health Outcomes: Identifying movement patterns correlated with low health outcomes can inform insurance risk models.
- Behavioral Variability: Understanding shifts in community activity patterns because of seasonal changes, offering insights into environmental health impacts.
- Regional Health Comparisons: Comparing activity levels in different urban vs. rural areas helps tailor health communications and policy initiatives.
Ultimately, geolocation data is the silent observer that compiles a narrative allowing insurance companies to craft data-backed policies that resonate with reality.
Sleep Research Data
Sleep data, sourced from institutions like the National Sleep Research Resource, takes a scientific perspective by focusing on sleep's impact on health - an area especially pertinent to life insurance models. Sleep studies have come a long way from their nascent days of portraying simple sleep cycles, evolving into comprehensive evaluations involving terabytes of data from varying cohorts and demographics. Historically, sleep data collection faced challenges due to the complexity of tracking physiological signals - a technologically demanding task that required dedicated environments and specialized equipment.
Today, polysomnographic (PSG) studies and actigraphy have come to the forefront, informing not only health researchers but also professionals across insurance, healthcare, and wellness industries. PSG records intricate physiological signals such as brain waves, oxygen levels, and bodily movements, creating a detailed dataset that allows insurers to explore correlations between sleep quality and incident risk.
Technological advances related to computerized measurement tools have produced an explosion in available sleep data. Innovations like accelerometers mean that wearable devices now gather sleep activity seamlessly, granting individuals and businesses alike insights that once required tailored studies.
Specific applications of sleep data for understanding health and mortality include:
- Mortality Risk Assessment: Categorizing sleep patterns that precede certain health outcomes aids in refining risk assessment models, significantly impacting policy pricing and terms.
- Sleep Disorders Evaluation: Evaluating correlations between poor sleep and chronic conditions allows insurers to predict long-term health care expenses.
- Product Development: Tailoring insurance products to cater to groups with atypical sleep behaviors that denote higher risk.
- Customer Engagement: Providing personalized feedback on sleep health encourages policyholder engagement with wellness programs.
- Predictive Health Insights: Understanding physiological markers in sleep studies informs risk factors and future health trajectories for specific demographics.
Incorporating sleep data is a forward-thinking strategy allowing life insurance professionals to react not only to existing health trends but to forecast impending shifts - a powerful asset for risk mitigation and policy refinement.
Conclusion
In conclusion, accessing different types of data, from geolocation insights to detailed sleep studies, provides an unmatched advantage for life insurance businesses seeking to understand the nuances of health and mortality. Embracing a multitude of data sources equips industry professionals to transcend traditional limitations, transitioning from a reactive mindset to a proactive, data-driven culture.
Organizations that lean into data discovery, as facilitated by platforms like Nomad Data's data search tools, will find themselves empowered to make informed decisions, fostering not only improved business outcomes but contributing to a healthier, more informed society. Data not only offers competitive edge, but also paves the way for smarter, more personalized interactions with policyholders.
Companies are increasingly looking to monetize their data, recognizing latent value hidden within historical datasets. The future promises a broadening spectrum of potential data sources that can enrich understanding of physical activity, sleep behaviors, and their implications on longevity and health outcomes.
Speculation suggests that forthcoming types of data for commercialization may include mental health metrics, cognitive function analysis, and behavioral health assessments—all of which hold potential to enrich health-related datasets further.
The evolving landscape demands that businesses adopt innovative ways of leveraging data, ensuring that they remain at the cusp of actionable insights and transformative discoveries in the domains of health, exercise, and longevity.
Appendix
The life insurance industry, among others, stands to gain immeasurably from incorporating diverse datasets into their decision-making processes. Roles such as investors, consultants, and market researchers frequently seek the insights data offers to unlock a deeper understanding of consumer health patterns and potential mortality risks.
For example, investors assess the risk profile of insurance companies by examining the granularity of their underwriting processes, which data directly influences. Consultants, on the other hand, assist clients in optimizing wellness programs based on data-driven insights into policyholder behaviors and health metrics.
Within insurance organizations, actuaries harness diverse datasets to refine premium pricing and risk models, ensuring they maintain a competitive and responsible approach to risk and customer engagement. Market researchers benefit from expansive data as it offers an unparalleled understanding of population health trends, enhancing their capability to predict future shifts in consumer behaviors and needs.
As businesses become more data-driven, they may increasingly look to systems like Nomad Data's platforms to discover and integrate training datasets into their analytical pipelines. The transformative potential of artificial intelligence and machine learning within this domain cannot be overstated as they unlock insights hidden within vast arrays of data.
AI holds the promise of deciphering complex patterns that were once shrouded in obscurity, offering unprecedented clarity and opportunities for actionable intelligence. As technology and methodologies continue to advance, the iterations of AI will only grow more sophisticated, positioned to offer a fresh perspective on longstanding questions regarding health and activity.
The road ahead for life insurance and related sectors is brightened by the wealth of data availability coupled with technological progress. With a commitment to data integration and a forward-thinking approach, organizations can look ahead to a future rich in insights and informed decision-making.