Unlock Retail Insights with Advanced Location Analytics Data
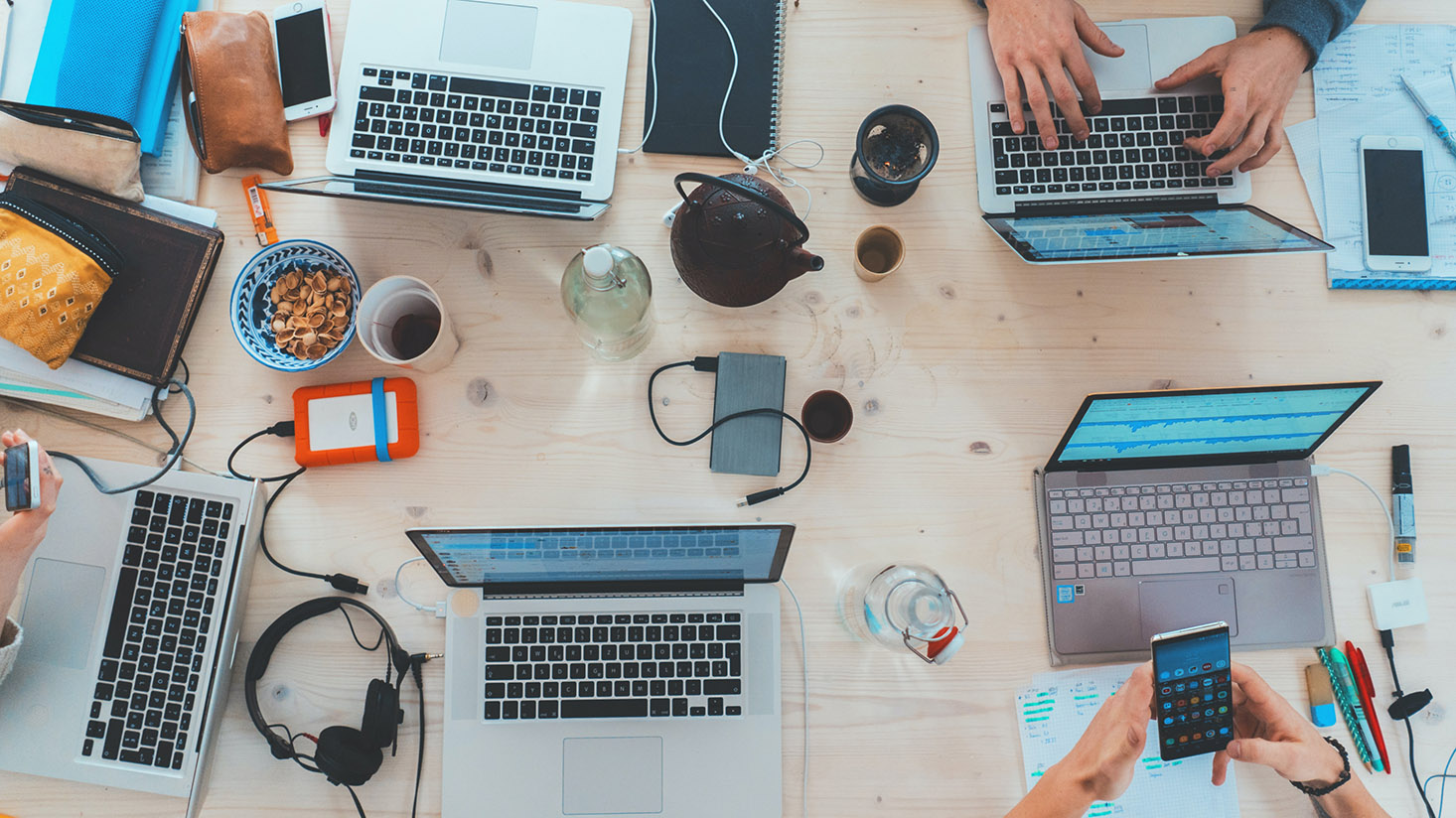
Unlock Retail Insights with Advanced Location Analytics Data
Introduction
In the retail industry, understanding consumer behavior and trends has always been pivotal for success. However, historically, gaining insights into footfall and shopping patterns was fraught with challenges. Retailers depended on traditional methods like manual traffic counters and customer surveys, which were both time-consuming and often inaccurate. Before firms had access to external data, these antiquated approaches provided incomplete pictures that required weeks or even months for analysis. This delay in understanding and adjusting to market conditions posed significant hurdles.
In an era before technological advancements, retailers were left to their own devices to estimate foot traffic or make educated guesses based on sales figures alone. The disconnect between action and information was glaring, leading to missed opportunities and inefficient business strategies. Simple consumer observation was insufficient to grasp the full spectrum of shopper activity across numerous categories such as grocery, restaurants, and apparel. The advent of sensors and the proliferation of software into business processes, however, signaled the dawn of a new era.
With the rise of the internet and connected devices, retailers suddenly found themselves with access to a wealth of data that could inform their business decisions in real-time. Modern technology advancements have made it easier to gather and analyze data from various touchpoints, offering detailed insights into location analytics. Data today can be collected seamlessly from customer interactions, sensor-driven technologies, and mobile applications, allowing businesses to adapt quickly to changing consumer preferences.
Location analytics has become a powerful tool, enabling the tracking of footfall and mapping customer journeys with unprecedented precision. Companies can now rank shopping centers in local areas by comparing foot traffic volumes against peers almost instantaneously. This capability allows firms to uncover cross-shopping patterns and develop strategies tailored to enhance the retail experience. The importance of these insights cannot be overstated in the rapidly evolving commercial landscape.
In today’s data-driven world, conversion from foot traffic to effective customer engagement relies heavily on timely and accurate external data. With data at their fingertips, businesses can analyze customer movements, identify peak traffic times, and adjust in-store strategies accordingly. Gone are the days of waiting for quarterly reports; now, real-time data provides the agility businesses need.
This article explores the crucial types of data that can illuminate different aspects of location analytics. Whether tracking footfall at retail centers or understanding customer journeys, the available datasets equip businesses with the insights needed to stay ahead. We will delve into various data categories, their historical context, technological advancements, and their impact on modern business strategies.
Geolocation Data
Geolocation data has emerged as a cornerstone in understanding footfall and customer behaviors across retail sectors. Historically, geographical information was confined to basic maps and manual customer check-ins, offering limited insights. Today, geolocation data offers a robust solution, harnessing GPS, Wi-Fi, and mobile device signals to deliver comprehensive analytics.
Example datasets in this category include mobile device location data covering entire regions. This training data reveals patterns in consumer movement and engagement, essential for businesses aiming to optimize retail operations. Industries such as investment and real estate have historically relied on these datasets to make informed decisions around property value and site viability.
Technological advancements facilitating the growth of geolocation data include the integration of smartphones and IoT devices, which provide more granular data than ever before. This progression allows businesses to leverage extensive datasets, providing a deeper analysis of consumer journeys. The accessibility and precision of this data type are accelerating, bringing new insights to bear on business strategies.
Applications of Geolocation Data in Retail
- Footfall analytics: Determine the volume and pattern of visitor journeys across different retail locations.
- Heat mapping: Identify areas within stores that attract the most attention, informing layout and marketing strategies.
- Peak time analysis: Align staffing and promotional efforts with customer influx patterns to maximize sales and customer satisfaction.
- Cross-shopping patterns: Analyze how customers transit between different stores or departments to optimize layout and sales strategies.
- Brand performance benchmarking: Compare foot traffic between competitors to enhance competitive analysis and positioning.
These applications showcase geolocation data's transformative potential, allowing companies to tailor offerings and marketing efforts to the unique dynamics of each venue.
Conclusion
In sum, the strategic use of data has revolutionized businesses in their effort to understand and engage with retail space dynamics and consumer behavior. From the rudimentary insights offered by antiquated methods to the refined and actionable intelligence enabled by modern data sources, retailers now navigate a landscape where they can decisively respond to trends and opportunities.
Data allows businesses to witness behavioral shifts and market changes in real-time, a capability that previously seemed out of reach. The multitude of AI applications continues to propel industries toward becoming more data-driven. Insights gleaned from data provide not only clarity in decision-making but also the agility needed for rapid adaptation and strategic foresight.
As organizations continue their quest to monetize their existing data, they will need to strategically evaluate which data types hold the most potential for commercial use. The retail sector, abundant with foot traffic and consumer data, is fertile ground for such efforts.
The increasing trend toward data monetization finds companies turning untapped data into a valuable resource. By effectively utilizing data, businesses stand prepared to seize new opportunities for growth and innovation within this digital era.
Future prospects in the realm of data for location analytics could include even deeper integration of consumer preferences and sentiment analysis to further enhance retail operations. As technology progresses, companies must remain attuned to novel data sources that will unlock new opportunities and enhance their strategic posture.
As companies embrace the monetization of data, the market for consumer insights will only expand, offering fresh opportunities for growth, differentiation, and competitive advantage.
Appendix
The harnessing of location analytics data unlocks new frontiers for a diverse range of roles and industries. Investors, consultants, insurance companies, market researchers, and policymakers have all begun to reap the rewards of insightful data analysis.
For investors, data-driven insights into retail footfall trends provide critical inputs for valuing properties or predicting sales trajectories. Location analytics can guide investment strategies, allowing decisions to be made based on observable consumer behaviors and not merely projections.
Consultants utilize datasets to help clients craft strategies that incorporate customer preferences and shopping patterns, thereby creating market differentiation. Insights gleaned from location analytics empower consultants to recommend the most effective adaptations in real estate positioning or marketing outreach initiatives.
Market researchers employ location analytics for a panoramic view of consumer journeys, analyzing the potential interactions and touchpoints between brands and consumers. This detailed data allows for segmentation and precision targeting like never before possible.
The insurance industry, although less intuitive, benefits from this data by predicting risk factors associated with particular locations, such as foot traffic or accident probability. This information allows for adjustment of premiums and the tailoring of insurance products.
The future of location analytics will likely see the integration of AI and machine learning capabilities, transforming raw data into strategic assets that deliver more personalized and predictive insights to enterprises of all kinds.