Unlock Retail Insights with Comprehensive Discounting Data
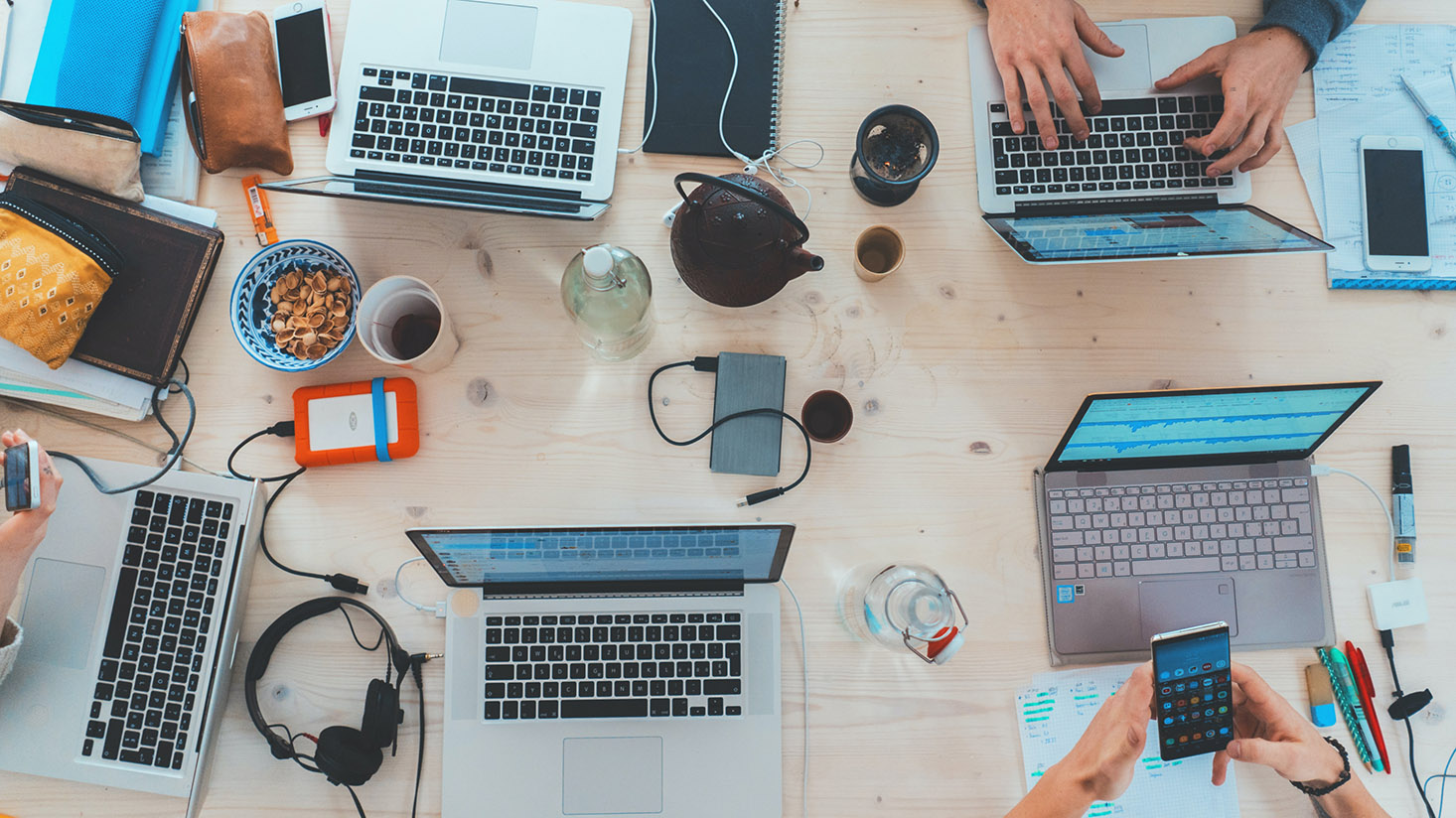
Introduction
Tracking the history of retail pricing and discounting has always been a complex and difficult endeavor. Over the decades, businesses have operated in the shadows, often resorting to guesswork when it came to understanding pricing trends. In the past, before the advent of modern data systems, retailers relied heavily on manual records and anecdotal information to keep track of pricing history. This often involved tedious processes of manually collecting and compiling data from sales receipts and ledgers, which was prone to errors and significant delays.
In ancient practices, bartering was a common method of exchange, and pricing was negotiated on a case-by-case basis with little standardized data on price history. With the proliferation of marketplaces and the formalization of currency, the ability to track pricing and discounting trends became more organized, yet still remained entrenched in manual processing. Entering the industrial era, businesses began employing basic accounting systems which provided some level of historical insights but were still heavily reliant on time-consuming processes.
As technology advanced, so did our methods of capturing and analyzing data. The transition to digital systems marked a turning point in data collection, making it easier to collate and analyze large volumes of data. The advent of the internet and connected devices, including external data platforms, has further transformed our capacity to gather real-time insights into pricing trends. Now, sensor data, online databases, and comprehensive software solutions capture every transaction and price change, which provides an unprecedented depth of understanding in retail discounting.
The importance of real-time data in understanding the topic of discounting cannot be overstated. Waiting weeks or months to analyze trends is no longer viable in today's fast-paced market. Immediate access to data allows retailers to quickly adjust strategies to optimize sales and profits. Without such instantaneous insights, businesses were forced to rely on outdated or incomplete data, leading to inefficiencies and missed opportunities.
As we delve into the specific categories of data relevant to understanding retail discount patterns, it becomes evident how crucial these datasets are. Modern businesses can leverage these insights to develop more sophisticated pricing strategies, understand competitive landscapes, and better cater to consumer demands in real-time.
Historically, firms had limited means to comprehensively track SKU-level price histories, relying on rudimentary methods that were costly and error-prone. But now, a cornucopia of data sources provides a holistic view that illuminates even the most subtle shifts in the market. With these advanced data capabilities, businesses have a powerful toolkit to make informed decisions, foster growth, and effectively manage their resources.
Sales and Pricing Data
History and Overview
Sales and pricing data has long been a cornerstone of retail analytics. Historically, this data was initially gathered through manual observations and simple accounting systems. The process required hand-written logs and registers that recorded each sale and its respective price, which required a significant amount of man-hours to maintain and analyze. As businesses expanded and consumer bases grew, the demand for more efficient, data-driven insights became apparent.
With technological advancements, sales data collection evolved significantly. The introduction of electronic cash registers initiated a digital transformation that propelled the collection of each transaction into structured databases. This evolution continued with the advent of enterprise resource planning (ERP) systems that facilitated the analysis of pricing over multiple channels.
Today, comprehensive datasets track discounting data for hundreds of retailers and numerous brands. These datasets include historical records of both list and selling prices, providing granular insights down to the SKU level. Retailers have access to information across different geographic markets, allowing for precise comparative analytics. For instance, tracking sales and pricing data within home improvement stores, whether in the US or UK, has become commonplace. This historical data serves as a pivotal tool for investment analysis and strategic decision-making.
Current Applications
- Investment Strategy: Investors use detailed pricing history to assess discounts and predict future revenue trends, striking a balance between price cuts and profitability.
- Competitive Analysis: Businesses analyze competitors’ discount patterns to refine their own pricing strategies and understand market positioning.
- Consumer Behavior Insights: By analyzing trends in sales and discounts, retailers can deduce consumer responses to price changes and seasonality effects.
- Product Lifecycle Management: SKU-level pricing data helps track product performance over time, indicating when to innovate or discontinue products.
- Dynamic Pricing Models: Robust datasets fuel algorithms that enable real-time pricing adjustments based on inventory levels, competitor pricing, and demand forecasts.
Accelerating with Technology
The integration of AI and machine learning with sales and pricing data continues to evolve the retail landscape. By leveraging AI, retailers can predict optimal pricing models and enhance automation in pricing adjustments, thus ensuring dynamic responses to market shifts. As the volume of available data grows, machine learning algorithms can refine their models to become increasingly accurate in forecasting and decision-making.
These technological advancements not only increase the accessibility of data but also enhance its granularity and accuracy, allowing for more robust analyses and strategic insights. As businesses strive to become more data-driven, effective utilization of sales and pricing data increasingly sets leading companies apart in the competitive retail market.
Point of Sale (POS) Data
Historical Context
Point of Sale (POS) systems have revolutionized the collection and application of sales data. Originally, POS systems simply referred to mechanical cash registers that recorded transactions one at a time. This process was manual and slow, limiting the potential insights derived from each sale. As computational technology improved, POS systems evolved into sophisticated digital platforms capable of capturing detailed transaction information efficiently.
With modern POS systems, every transaction is logged instantly, capturing not just the SKU and price, but also metadata such as time of purchase, location, and payment method used. The integration of these systems with online platforms further broadens their scope, encompassing e-commerce data alongside in-store transactions.
These systems have become integral to operations across retail sectors, providing retailers with real-time, actionable insights into stock levels, sales patterns, and consumer behavior.
Utilizing POS Data
- Inventory Management: POS data allows for precise tracking of stock levels, optimizing supply chain operations and minimizing overstock or stockouts.
- Sales Forecasting: Analyzing historical sales data facilitates more accurate forecasting of future sales trends, aiding in resource allocation and strategic planning.
- Promotional Effectiveness: By evaluating sales during promotion periods, retailers assess the success of marketing campaigns and adjust strategies accordingly.
- Consumer Experience Enhancement: Real-time data enables personalized customer interactions, fostering loyalty through targeted offers and recommendations.
- Data-driven Marketing: Insights gleaned from POS data guide marketing strategies, enhancing customer segmentation and target marketing initiatives.
Advancements and Opportunities
The burgeoning growth in POS data has opened new avenues for analytics and business transformation. Advanced analytics platforms paired with POS data provide unparalleled insights into consumer trends and market dynamics. Retailers who effectively harness these insights can sustainably enhance both profitability and consumer satisfaction.
As data from these systems becomes more entwined with other retail analytical tools, businesses can anticipate and react dynamically to marketplace fluctuations, harnessing the full potential of integrated data-driven strategies.
Conclusion
In an age where data reigns supreme, understanding the intricacies of retail discounting has never been more accessible. With an arsenal of data types at their disposal, business professionals can delve deeper into the dynamics of pricing, gaining a nuanced understanding of consumer behavior and market trends. Through strategic utilization of both sales and pricing data, along with external data sources, businesses are positioned to make informed decisions and evolve alongside a rapidly changing retail landscape.
Data-driven decision-making is vital for modern organizations, and the ability to discover and apply diverse datasets to retail strategies is a competitive advantage. The increased focus on data monetization underscores the importance of these insights. As organizations look to monetize and share their data, they contribute to a global ecosystem where data accessibility and utility continue to expand, transforming industries.
The retail sector is no exception, as companies continually find innovative ways to leverage existing data to drive growth. Looking to the future, we can expect retailers to explore new types of data that can offer fresh perspectives on consumer preferences and market opportunities.
Emerging technologies will further enrich these datasets, providing businesses with even finer-grained analyses. New data streams, possibly from unstructured sources or emerging technologies, will likely become critical components in retail analytics, augmenting current insights.
Thus, as businesses become more adept at unlocking these valuable insights, they will be able to preemptively navigate the complexities of the modern market, setting the stage for sustained innovation and growth.
Appendix
The wealth of data available today has implications far beyond retail, impacting a myriad of industries and roles. Investors, for instance, have leveraged discounting data to gauge market sentiment and identify lucrative investment opportunities. By understanding discount trends, they fine-tune their portfolios to reflect evolving consumer preferences and market shifts.
Consultants benefit from these datasets by advising clients on strategic approaches to pricing and discounting. Armed with detailed insights, they help businesses refine their competitive positioning, enhancing brand value and market share.
In the market research sector, access to robust datasets provides a foundation for understanding consumer behavior, guiding product development, and optimizing marketing campaigns. Utilizing sophisticated algorithms, researchers can glean insights from historical price data to forecast trends more accurately.
Moreover, insurance companies might find value in such data when evaluating the fiscal health of retail operations, thereby calibrating risk assessments and insurance offerings more effectively.
Looking forward, the integration of AI presents an exciting frontier for unlocking the potential hidden in voluminous datasets. AI has the capacity to automate trend identification and provide predictive insights, revolutionizing strategies across the board.
Whether it’s through uncovering insights from training data or extracting value from contemporary market sources, the future is poised to usher in a new era of data utilization. As industries adapt, those who effectively embrace the opportunities provided by data will thrive.