Unlocking Business Insights Through CAC and LTV Data
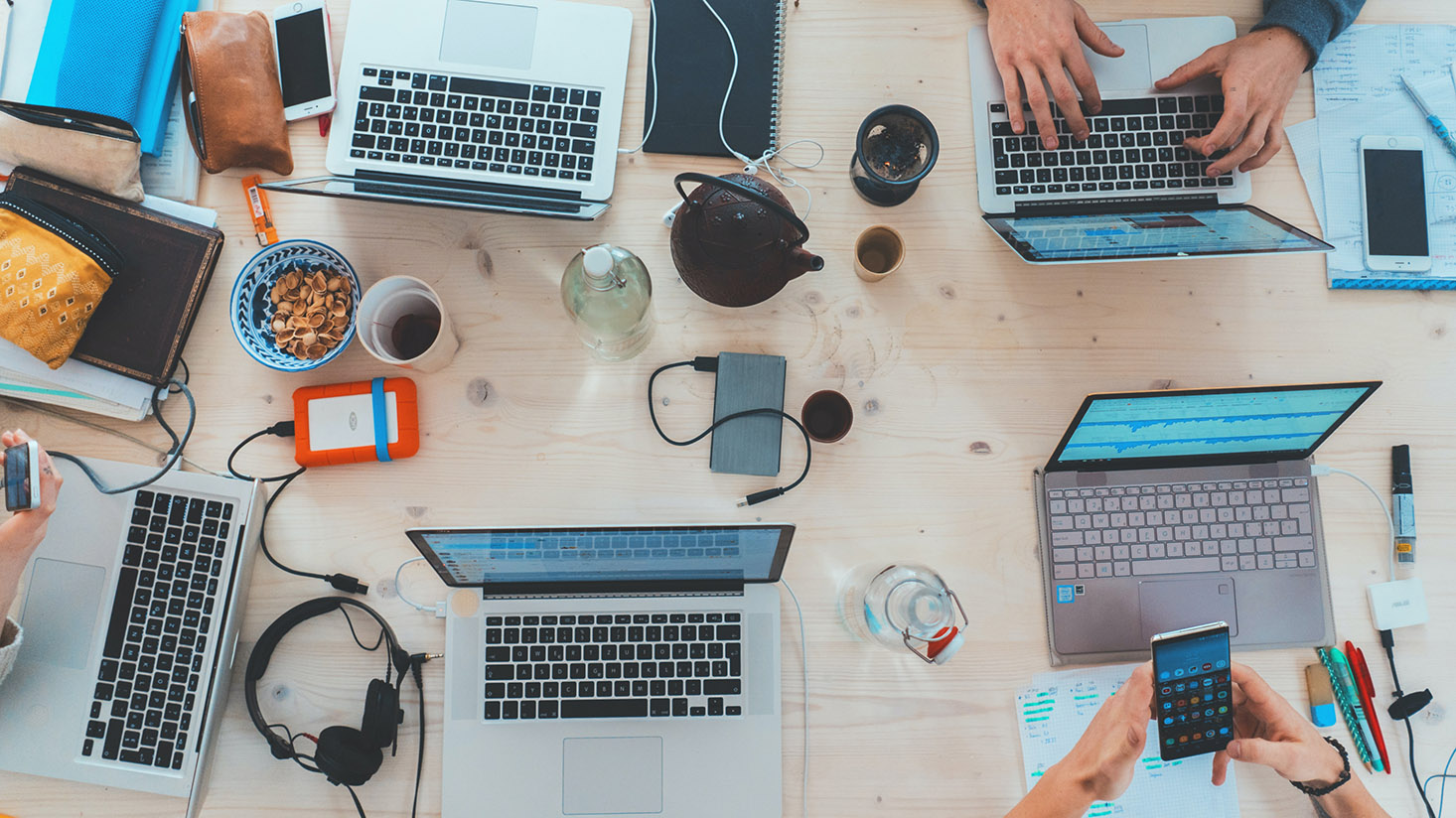
Unlocking Business Insights Through CAC and LTV Data
Introduction
In the dynamic landscape of digital health, understanding customer acquisition cost (CAC) and customer lifetime value (LTV) has become paramount for businesses aiming to enhance their strategic decisions. Historically, firms had limited means of gauging these critical metrics, relying on rudimentary methods that often painted an incomplete picture. Before the era of data monetization and sophisticated analytics, businesses relied on traditional metrics like sales reports or customer feedback collected through surveys and focus groups. These methods were labor-intensive and required long cycles of data gathering and processing, often leaving companies lagging in their response to market shifts.
Before the digitalization wave, many companies operated in the dark, lacking access to real-time information about customer interactions and behaviors. It took weeks, if not months, to compile data, analyze trends, and make informed decisions, which hampered businesses' agility in a competitive environment. As the digital age dawned, the proliferation of sensors, the internet, and connected devices offered a new realm of possibilities for data collection, making real-time tracking of customer behaviors possible.
With the advent of the internet and the expansion of software into numerous business processes, the storage and accessibility of data have witnessed a significant transformation. Companies can now capture every interaction and transaction, allowing for a comprehensive analysis of customer journeys. This transformation is particularly vital in the digital health sector, where understanding CAC and LTV provides insights into the efficiency of marketing channels and customer retention strategies.
Data has revolutionized how we understand and interact with market trends, offering clarity that was once elusive. In the past, businesses navigated with limited guidance, but today, with data, insights can be generated swiftly and accurately. This evolution has provided unparalleled opportunities for companies to engage meaningfully with their audience, tailor their offerings, and optimize marketing spend to enhance ROI.
The importance of data in unraveling customer behaviors and preferences cannot be overstated. Data empowers businesses to craft strategies informed by solid analytics rather than intuition or outdated practices. Real-time data tracking also enables swift course corrections in strategy based on immediate customer feedback, which is crucial in maintaining competitive advantage.
Ad Targeting Data
Understanding Ad Targeting Data is crucial for delineating effective strategies around CAC. Over the last decade, the evolution of this data type has been remarkable, driven largely by advances in internet infrastructure and analytics technology. Originally, ad targeting was limited to broad demographic categorizations. However, the emergence of refined datasets has allowed companies to hone in on target segments with increasing precision.
Ad Targeting Data has typically included an array of metrics such as audience demographics, behavior patterns, and engagement levels. This information is invaluable for marketers and advertising agencies as they strive to reach the right audiences in the most efficient manner possible. Such data enables businesses to reduce waste in advertising spend by focusing efforts on channels and audiences that promise the highest returns.
Industries ranging from retail to technology have historically harnessed such data to optimize ad spend and enhance targeting efficiency. The growing demand for personalization in marketing campaigns has further accelerated the refinement of this data, incorporating everything from browsing history to purchase intentions.
Technological advancements, particularly in AI and machine learning, have spurred the amount of Ad Targeting Data available for analysis. These technologies facilitate the creation of more nuanced audience segments and enable predictive analytics, helping marketers anticipate customer needs before they even manifest.
Using Ad Targeting Data for CAC and LTV Insights
- Comparative Analysis: By analyzing spending patterns across different channels, businesses can identify high-performing channels that contribute to reduced CAC.
- Behavioral Targeting: Insights drawn from user interaction data allow companies to tailor messaging that resonates with specific audiences, improving conversion rates and subsequent LTV.
- Channel Strategy Optimization: Understanding which channels yield the highest engagement informs the strategic allocation of marketing budgets.
- Creative Strategy Development: Insights into creative performance across platforms assist in crafting more effective advertising content.
- Real-Time Adjustments: Daily updated datasets enable immediate adjustments to campaigns, enhancing effectiveness and efficiency.
Transaction Data
The role of Transaction Data in discerning customer lifetime value and acquisition costs is pivotal. Transaction data offers granular insights into consumer spending habits, revealing not only the purchase value but also the frequency and modality of transactions.
This type of data has been a staple for sectors such as retail, financial services, and e-commerce. Traditionally gathered through credit card processing, loyalty programs, and point-of-sale systems, it provides a mirror to the client's financial interactions, illustrating spending trends and predicting future behaviors.
Applications in Digital Health
In the context of digital health, this data is indispensable for understanding how customers interact financially with digital health services:
- Customer Segmentation: By breaking down transaction data, businesses can identify distinct user groups and tailor their offerings accordingly.
- Retention Strategy Development: Recurring payments and frequency of transactions provide insights into customer loyalty and product satisfaction, critical for LTV calculations.
- Pricing Strategy Formulation: Analyzing average transaction values assists companies in optimizing pricing strategies across various services.
- Predictive Analytics: Historic transaction patterns aid in forecasting future revenues, crucial for planning marketing and operational strategies.
- Channel Performance Assay: Dissecting transaction data per channel offers transparency on the efficiency of each medium in customer acquisition and retention.
Conclusion
The journey of understanding Customer Acquisition Cost and Customer Lifetime Value in digital health has transitioned remarkably from opaque and dated methods to data-driven clarity. Different types of data like Ad Targeting Data and Transaction Data are transforming the sector by offering granular insights into spending behaviors, channel efficiencies, and customer retention metrics.
The attitude towards data is evolving rapidly, as organizations recognize the immense value data-driven decisions can provide. As more companies seek to monetize their data, the landscape becomes increasingly rich with opportunities for deeper insights and enhanced analytics.
Given the accelerating quantity and quality of available data, businesses are equipped to explore novel metrics and models to stay ahead of market trends. As the fusion of AI with data analytics becomes more prevalent, the predictive capabilities will burgeon, further enhancing understanding via historical and real-time data.
In this data-driven era, the importance of data discovery cannot be overstated. Venturing into uncharted data territories promises to unearth actionable insights that not only optimize marketing expenditures but also drive strategic growth.
Appendix: Industry Impact and Future Potential
The application of customer acquisition and lifetime value data is broad, impacting numerous roles and industries. Marketing strategists, data analysts, and business consultants across sectors leverage these insights to tailor their approaches, ensuring efficiency and efficacy.
Historically, industries such as digital health, e-commerce, and telecom have faced challenges in accurately projecting growth due to the volatile nature of customer behaviors and preferences. However, with data, past uncertainties are being untangled, leaving strategies more grounded in empirical evidence than ever before.
Looking forward, the banking and insurance sectors have exciting potential with this data, as they pivot towards personalized financial products and insurance offerings based on transactional and ad targeting insights.
The advent of Artificial Intelligence promises to revolutionize how businesses interact with aged documents and filings, extracting and synthesizing hidden value for modern applications.
Ultimately, as the synergy between data and AI grows, sectors will witness unprecedented opportunities to re-think their models, transforming raw data into predictive catalysts for business innovations.