Unlocking Commodities Pricing Insights with Comprehensive Data Analysis
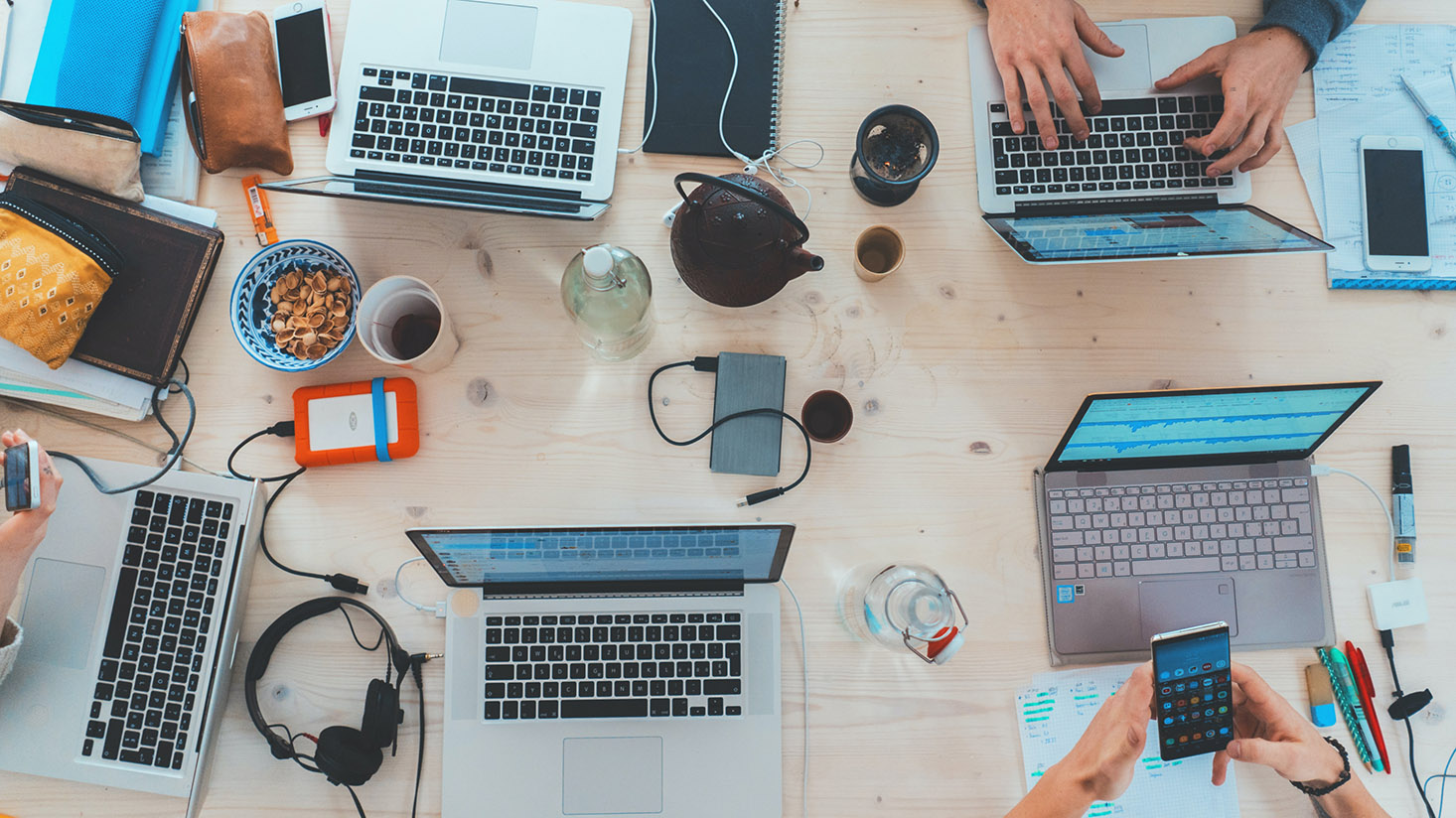
Unlocking Commodities Pricing Insights with Comprehensive Data Analysis
Introduction
In the ever-evolving world of commodities trading, obtaining precise insights into pricing has historically been a challenging endeavor. Financial markets have long depended on an amalgam of traditional techniques and tools to gauge commodities pricing. Before the digital era opened up new horizons in data acquisition, traders and investors primarily relied on antiquated methods which often left them grasping in the dark.
Traditional methods ranged from interpreting verbal reports and market speculations to manually assessing newspaper market listings. These tools did little to provide accurate or real-time insights. With limited connectivity in global markets, it was common for professionals to wait weeks, or even months, before they could gauge pricing trends and market shifts across critical commodities such as metals and polymers.
The advent of advanced technologies, including the internet and interconnected devices, has revolutionized this landscape. Today, with the help of state-of-the-art sensors and sophisticated software systems, data collection has become more granular and instantaneous. The omnipresence of digital recording tools ensures that every flicker in market activity is captured and stored in expansive databases.
This transformation underscores the critical role that data plays in understanding commodities pricing. Previously, professionals attempting to anticipate changes in the commodities market operated in relative obscurity. They now have access to data that provides real-time insights, a game-changer in rapidly making informed decisions.
For stakeholders, particularly institutional investors, the shift toward relying on external data sources has essentially eliminated the prolonged delays that once stifled timely market responses. Utilizing precise and up-to-date information enables investors to adapt quickly to market dynamics, mitigating risks and identifying potential opportunities.
With the rise in demand for real-time pricing data, understanding the right types of data that can assist in these challenging scenarios is imperative. Let's delve into several categories of data that can extend profound insights into commodities pricing and help transform strategic decision-making.
Commodities Data
The realm of commodities data has seen immense growth in recent years. Historically, commodities data encompassed basic trade records and sporadic pricing indicators. With the evolution of technology, this data category has transformed, offering extensive historical and real-time datasets, detailed price points, shipment records, and comprehensive trade flow analysis.
Key to industry professionals, such as equity investors and portfolio managers, commodities data provides the foundation needed for sound strategy building. Several technology advancements, including robust API integrations and data visualization tools, have made it simpler for industries to tap into these valuable insights.
Examples of Commodities Data Utilization:
- Understanding global trade flows: Analyze detailed records of commodities traded globally, offering clarity into market dynamics.
- Assessing supply and demand: Predict and evaluate changes in commodities supply chains and demand metrics.
- Real-time pricing data: Access spot and future price data to make informed trading decisions.
- Identifying geopolitical influences: Monitor how global events impact trade routes and pricing structures.
- Benchmarking market trends: Compare historical data to current trends for future forecasting.
The acceleration of data in the commodities sphere ensures stakeholders receive a fuller, more nuanced picture of the pricing landscape.
Sales and Pricing Data
The significance of sales and pricing data cannot be overstated when it comes to understanding fluctuations in commodities markets. Historically, this data was sparse and often inclusive of oversimplified market indexes. The data community is now witnessing a shift toward comprehensive datasets with intricate details on commodities like metals, paper products, and plastics, encapsulating current and historic prices.
Traditionally used by retail businesses to set competitive prices, sales and pricing data helps track trends across complex supply chains. This data can be leveraged by procurement specialists, financial analysts, and entrepreneurs keen on refining their market approach or optimizing supply costs.
Specific Applications of Sales and Pricing Data:
- Track material cost changes: Gain insights into how raw material pricing affects broader market strategies.
- Analyze competitive pricing strategies: Compare competitor pricing movements and make informed adjustments.
- Forecasting demand shifts: Utilize historic price trends to anticipate future demand changes in key commodities.
- Cost optimization: Identify pricing inefficiencies in production processes.
- Investment strategy refinement: Align financial investments better with expected market behaviors.
With accelerating access to historical and real-time sales and pricing data, businesses can better strategize and maintain competitiveness amid fluctuating commodities markets.
Conclusion
In conclusion, the importance of data in unraveling the complexities of commodities pricing is unequivocal. Having pertinent and robust datasets allows business professionals to fathom deeper market insights, directly influencing enhanced decision-making. In an era where being data-driven is no longer an option but a necessity, accessing diverse types of data is more integral than ever before.
Corporations are increasingly exploring data monetization opportunities, tapping into valuable datasets that they have amassed over decades. This conversion of dormant data into actionable intelligence represents a promising future. As businesses digitize their operations, the potential expansion of new data types will offer fresh insights into the commodities sector.
Whether through leveraging improved data analytics or harnessing advanced technologies like AI, the potential of data to influence the future of commodities pricing is boundless. Companies that champion this approach will position themselves at the forefront of market innovation.
Looking ahead, one might envision companies selling enriched datasets that distill granular insights on climate impact to production values, offering novel perspectives on commodities pricing. For industry leaders, adopting a data-centric approach isn't just about gaining a competitive edge — it’s about unlocking a trove of possibilities.
In this rapidly changing market landscape, the enterprises that embrace data are setting the standard for innovation and industry leadership. Rising data categories continue to unfold, promising to redefine how we perceive and act on commodities pricing in the future.
Appendix
Multiple roles and industries stand to benefit vastly from the development and availability of rich commodities data, birthing transformative solutions across varying sectors.
Consider the financial domain, where institutional investors and analysts employ commodities data to frame investment strategies and forecast market movements. The fluidity of real-time pricing information inputs is crucial for stress-testing investment portfolios against market volatilities.
Within consulting, advisory firms capitalize on data-driven insights to guide clients through market challenges. Commodities pricing data forms a foundation to enhance market analysis and develop solutions for practitioners aiming to thrive despite systemic disruptions.
Market researchers delve into these datasets to unravel intricate web patterns in supply chain dynamics or consumer behavior, facilitating comprehensive market-driven reports that assist stakeholders in navigating complexities.
Insurance companies, adept at managing risks, find value in commodities data, especially in calculating potential claims triggered by shifts in pricing policies or geopolitical events raising operational costs.
Excitingly, the future holds tremendous potential with technological advancements. AI and machine learning will bolster companies to extract value from historic documents and integrate disparate datasets for greater actionable insights. Unraveling patterns hidden within voluminous data is poised to revolutionize sectors beyond traditional confines.