Unlocking Consumer Sales Insights with Comprehensive Transaction Data
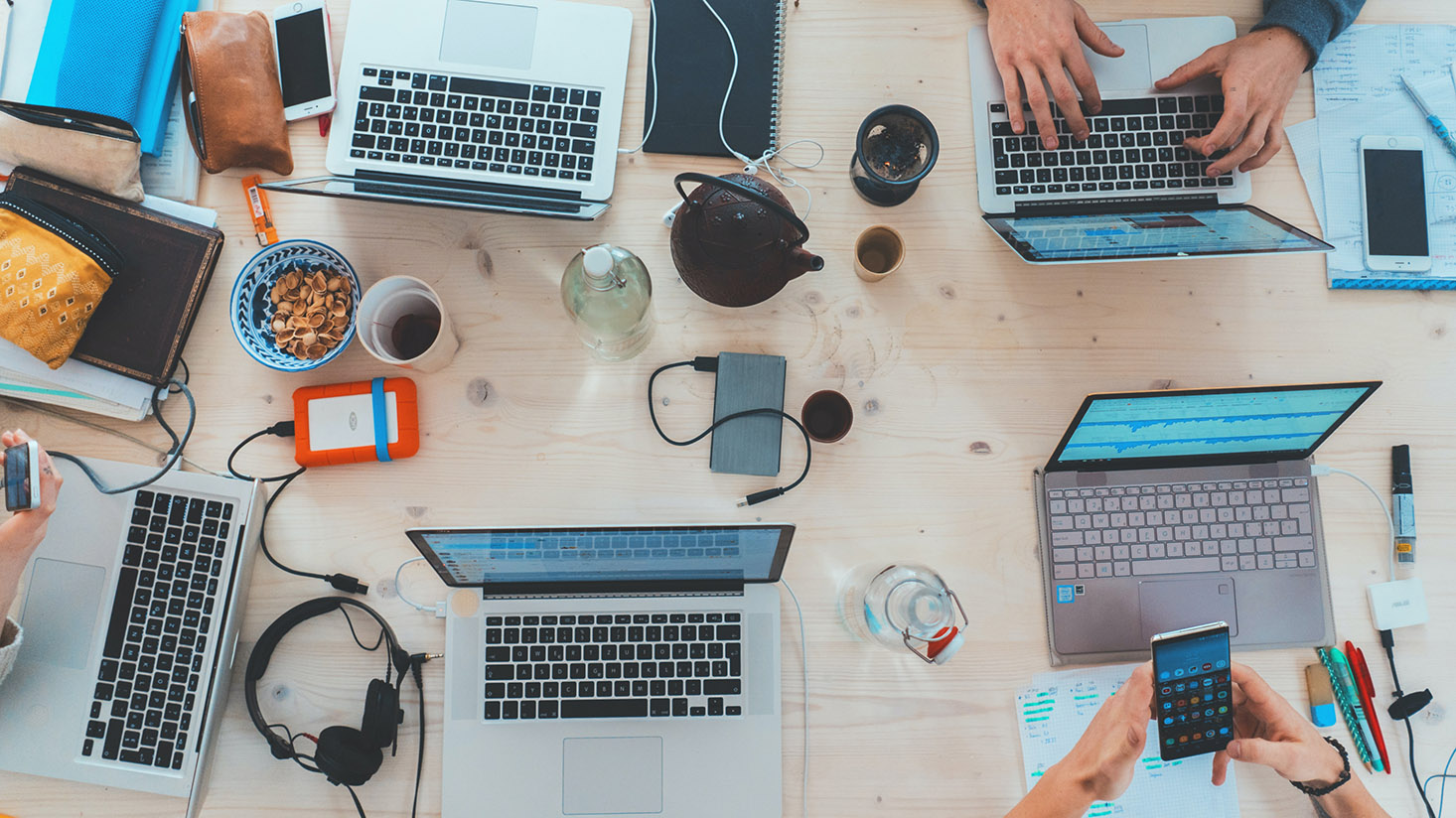
Introduction
Understanding consumer sales trends has long been a challenging task for businesses and market analysts alike. Before the digital revolution, firms primarily relied on an array of anecdotal and loosely gathered qualitative data to make business decisions regarding consumer preferences and sales trends. Market research used to depend heavily on customer surveys, focus groups, and local store feedback, which were not only time-consuming but also lacked the precision and scope we see today.
In earlier times, when most processes were conducted manually, businesses had to employ labor-intensive methods such as manually counting sales transactions and physically inspecting shelves. These antiquated methods provided limited insights and were prone to errors. It was tough to pinpoint exact sales figures, product popularity, or consumer behavior patterns with any accuracy.
With the advent of the internet, sensors, and connected devices, the landscape of consumer sales data began to change dramatically. The proliferation of e-commerce and the digitization of sales processes have made it possible to track and store vast amounts of data effortlessly. This revolution was propelled further by the development of sophisticated databases and cloud storage solutions, which can handle enormous volumes of data and make it instantly accessible.
Today, the importance of data in understanding consumer behavior cannot be overstated. The paradigm shift to a data-driven approach has empowered businesses to transition from reactive to proactive strategies. Instead of making assumptions based on outdated reports, firms can now access real-time data, enabling quicker and more precise decision-making that enhances their market responsiveness.
As we continue to integrate technology with business processes, the ability to gain insights from consumer sales data has transformed dramatically. This article explores how different types of data can illuminate the intricacies of consumer markets, providing businesses with a clearer view of the landscape and enabling them to navigate it successfully.
Point of Sale Data
The Evolution of Point of Sale Data
Point of Sale (POS) data has undergone significant transformation over the years. Originating with simple cash register reports, it has evolved into sophisticated systems that capture every detail of a consumer transaction. Earlier generations of POS systems recorded basic information such as the number of items sold and the total sales amount. However, today's POS data includes vast amounts of detail, capturing data from every transaction, including itemized sales, time stamps, and customer preferences.
Applications Across Industries
The retail industry has been the primary beneficiary of POS data, using it to track inventory, manage shelf space, and optimize sales strategies. Beyond retail, industries such as hospitality, food services, and consumer goods have utilized this data to gain insights into customer purchasing patterns and improve service delivery. By analyzing POS data, businesses can better predict demand, streamline operations, and enhance customer experiences.
Technological Advances
The introduction of cloud computing and machine learning has accelerated the evolution of POS data. Real-time data analysis enables businesses to act swiftly, adjusting their strategies to current market trends. Furthermore, integration with CRM systems allows firms to personalize consumer interactions, greatly enhancing customer satisfaction and loyalty.
Using POS Data to Understand Consumer Sales
With the accumulation and analysis of POS data, businesses can gain valuable insights into consumer sales trends. Some applications include:
- Monitoring Market Trends: Identifying popular products and fluctuating demand patterns to stay ahead of competitors.
- Improving Inventory Management: Reducing stockouts and overstocks with precise inventory controls based on real-time sales data.
- Enhancing Customer Experience: Tailoring offerings to match consumer preferences through personalized promotions and recommendations.
- Understanding Consumer Behavior: Analyzing purchasing patterns to develop targeted marketing strategies and enhance brand engagement.
- Measuring Promotion Effectiveness: Evaluating the impact of sales strategies and promotional campaigns on consumer purchasing decisions.
Alternative Data
The Rise of Alternative Data
Alternative data has gained prominence in providing unique insights not captured in traditional data forms. This data type includes information derived from online transactions, social media, web scraping, and other non-traditional sources. It complements existing data sets by providing additional context and granularity unavailable through standard channels.
Diverse Applications
Alternative data is used extensively in financial services, where it aids in investment analysis and risk management. Retailers and consumer goods companies leverage it to identify emerging trends and enhance product development. Furthermore, policy analysts and marketers use alternative data to understand shifts in consumer sentiment and behavior.
Technological Integration
The integration of alternative data with AI and machine learning technologies has unlocked predictive capabilities. By processing large data sets, these technologies identify patterns, trends, and anomalies that inform strategic decision-making processes. As alternative data streams multiply, businesses gain a broader understanding of the consumer landscape.
Using Alternative Data for Consumer Sales Insights
By leveraging alternative data sources, companies can deepen their understanding of consumer sales patterns in ways they couldn't before:
- Trend Analysis: Assess emerging consumer preferences by analyzing social media trends and sentiment data.
- Competitor Benchmarking: Compare performance metrics against competitors by utilizing web scraping data and market analytics.
- Customer Segmentation: Distinguish between different consumer demographics for targeted marketing efforts.
- Market Forecasting: Use predictive models to anticipate future sales trends and adjust strategies accordingly.
- Enhanced Personalization: Improve customer engagement through personalized offers and recommendations based on behavior analysis.
Transaction Data
The Advent of Transaction Data
Transaction data, often collected at the point of sale, touches on detailed elements of consumer purchases, including SKU-level information. As opposed to aggregated sales data, transaction data offers deeper insights into individual buying habits and preferences. This granular data is key in understanding the broader consumer landscape.
Industries Leveraging Transaction Data
The consumer packaged goods (CPG) industry extensively uses transaction data to assess product performance and market penetration. Financial institutions also rely on transaction data for developing consumer credit profiles and risk assessments. Additionally, businesses across travel, healthcare, and hospitality sectors utilize it to enhance customer service and operational efficiency.
The Technological Edge
The development of big data analytics and cloud storage has allowed businesses to handle and analyze massive transaction datasets efficiently. Real-time processing technologies empower firms to make instantaneous business decisions, optimizing processes and maximizing profitability across each sales channel.
Unlocking Consumer Insights with Transaction Data
Transaction data offers a rich source of information for businesses to explore consumer sales dynamics. Here's how:
- Behavioral Analysis: Understand customer purchasing behaviors at a granular level for refined marketing efforts.
- Sales Optimization: Adjust pricing strategies, promotional offers, and retail displays based on transaction trends.
- Supply Chain Management: Optimize logistics and inventory management by aligning supply chain operations with demand forecasts.
- Competitor Analysis: Benchmark performance against industry peers through detailed transaction comparisons.
- Data-Driven Innovation: Drive innovation in product development and customer experiences by leveraging comprehensive transaction insights.
Conclusion
In conclusion, understanding consumer sales patterns has become more accessible and precise, thanks to the proliferation of data categories like POS data, alternative data, and transaction data. This transformation has equipped businesses with the ability to make informed decisions based on real-time insights, a feat that was nearly impossible with older methods.
Access to diverse types of data has further contributed to this evolution. From retailers and CPG manufacturers to service-based industries, a data-driven approach enables professionals to gain clarity and flexibly adapt to market changes. The consumer sales landscape is defined by its rapid shifts, making agile response mechanisms a necessity for competitive survival.
As organizations increasingly prioritize data-driven methodologies, the need for effective data discovery becomes paramount. Platforms that facilitate data search and offer centralized access to valuable datasets play a pivotal role in this new paradigm of business intelligence.
Globally, there is an increasing trend toward the monetization of data. Companies are exploring new revenue streams by commercializing data collected through everyday operations, offering valuable insights to various stakeholders. Consumer sales data is increasingly part of this ecosystem, providing unique perspectives to businesses looking to understand and serve their markets more effectively.
In the future, we may see the emergence of new data types, such as location-based purchase histories or integrated digital shopping habits, which can offer even more comprehensive insights into consumer behavior. As organizations embrace these data opportunities, the potential to reshape industries through innovative data strategies is immense.
Appendix
Industries Benefiting from Consumer Sales Data
Various industries can benefit from insights gained through consumer sales data, each employing data to solve specific challenges and enhance operations. Among them are:
Investment and Financial Analysis
Investors and financial analysts use consumer sales data to forecast economic trends and make informed investment decisions. By analyzing sales performance, they can predict company growth prospects and market dynamics, ensuring accurate valuations and enhanced portfolio management.
Consulting and Market Research
Consultants and market researchers leverage vast datasets to support strategic planning efforts, conduct market analysis, and provide actionable recommendations to clients. Consumer sales data aids in understanding market dynamics, competitive positioning, and emerging trends.
Insurance
Insurance companies rely on consumer data to assess risk profiles and adjust policies accordingly. By incorporating sales trends and spending patterns, insurers can offer tailored coverage options and identify opportunities for product development and reduction of unnecessary risks.
Unlocking Future Potential
The future holds tremendous potential for data utilization, particularly in the realm of AI and machine learning. AI solutions can unlock hidden value in historical data and provide predictive capabilities for modern analytics. For example, machine learning models can uncover correlations and patterns in consumer behavior, enabling businesses to predict trends and respond proactively.
AI-driven tools further allow companies to extract insights from documents and government filings that once felt impenetrable. By using training data to instruct AI systems, businesses can gain significant competitive advantages through data-driven insights.