Unlocking Driving Risk Insights with Comprehensive Telematics Data
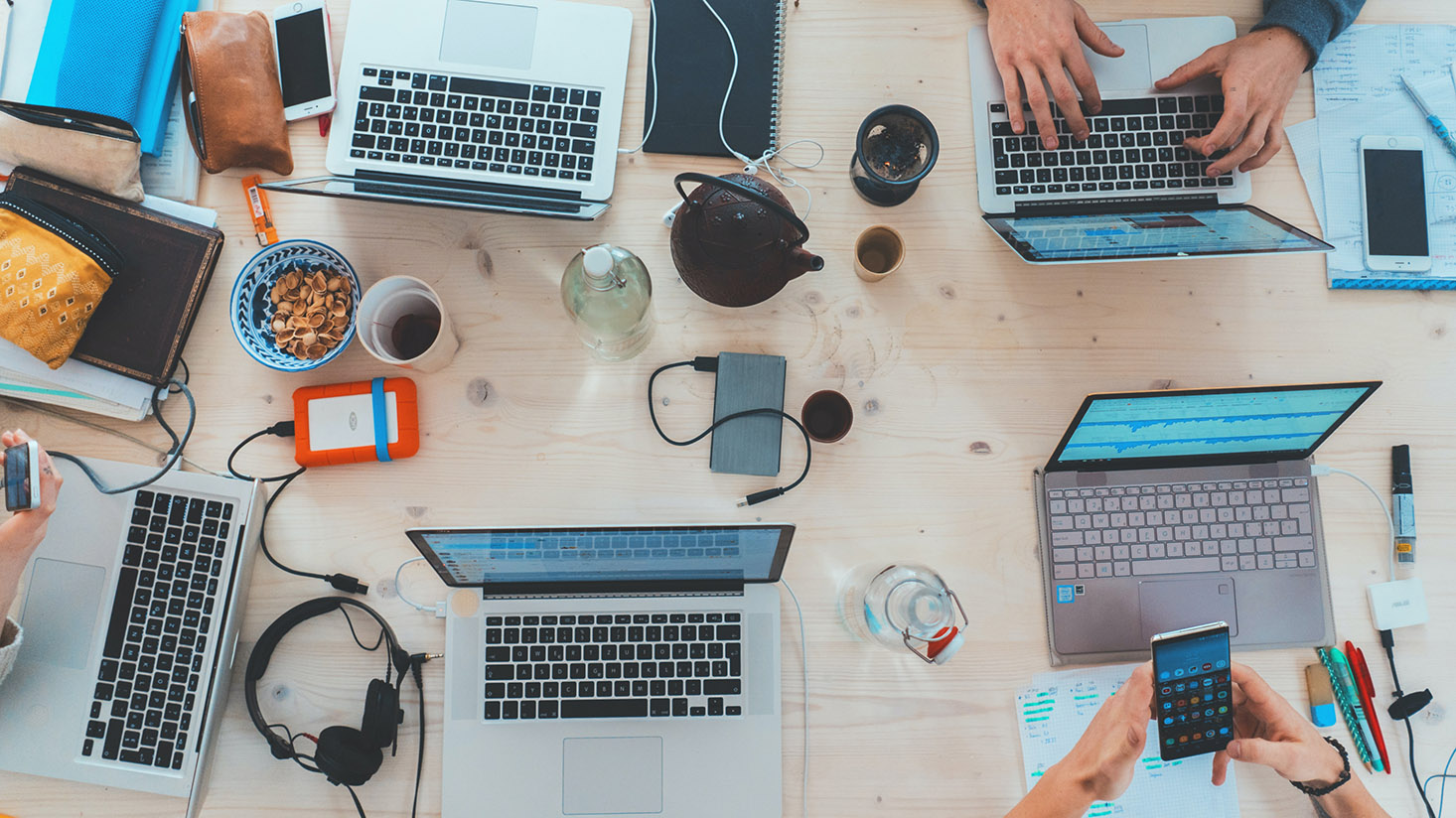
Unlocking Driving Risk Insights with Comprehensive Telematics Data
Introduction
Understanding driving risks has always been a complex endeavor, one that until recent decades was shrouded in mystery and speculation. Historically, the ability to predict driving risk and manage it effectively depended on claims data and reports, often lagging behind by weeks or even months. Before the advent of digital technologies and data analytics, organizations relied on anecdotal evidence, manual record-keeping, and basic statistical methods to assess risk and develop strategies.
Historically, insurance companies and fleet managers depended on sparse data from surveys, observational studies, and post-accident reports. This data collection method was laborious and time-consuming, producing insights that were often outdated by the time they were analyzed. Utilization of such data lacked the precision needed for accurate risk assessments, leading to generalized assumptions rather than actionable insights.
The digital transformation, marked by the widespread use of sensors, the Internet of Things (IoT), and advanced telematics systems, has revolutionized how driving risk is measured and understood. This proliferation of connected devices now enables real-time data collecting and analysis, providing insights that were previously unobtainable. Vehicle telematics, in particular, has transformed the landscape by ensuring extensive tracking and monitoring capabilities that capture driving behaviors and conditions instantaneously.
With databases now recording every event from acceleration to braking patterns, organizations can evaluate driving risk as it happens. This agility in data collection means that insights are not only timely but tailored to individual driving profiles, truly leveling up risk assessment precision. The role of external data has only expanded, providing a wealth of possibilities to leverage detailed information to enhance risk models.
In this evolving space, the importance of collecting and utilizing comprehensive datasets cannot be underestimated. Companies that enhance their strategies with detailed insights from telematics data can swiftly adapt, offering consumers and stakeholders alike a more polished and effective response. The surge in data-driven decision-making marks a new era where adaptability is key to navigating the ever-changing technological landscape.
Today, being data-driven isn't just an industry catchphrase; it's a business imperative. This article explores how categories of data related to telematics can illuminate the shadowy complexities of driving risk, providing clarity and detail that was once the stuff of imagination.
Automotive Telematics Data
Telematics data, which links telecommunications and informatics, has become a pivotal asset in the realm of automotive analytics. This category of data emerged as technology advanced to deliver real-time communications from vehicles to centralized databases. Initially adopted by fleet operators to track vehicle locations and fuel usage, it has now matured into a robust dataset capturing various elements of vehicle operations and driver behavior.
Roles such as fleet managers, insurance underwriters, and urban planners have historically utilized telematics data to enhance their efforts in optimizing vehicle usage, calculating insurance premiums, and planning transportation systems. The rise of the smartphone, GPS technology, and connected car platforms has further propelled the accessibility and depth of this data.
Today, the acceleration of telematics data accumulation is remarkable. Sensors embedded in vehicles track metrics like speed, route patterns, idling times, harsh driving practices, and even the frequency of trips. These data elements converge to create a comprehensive view of driving behaviors, which can be analyzed to assess risk levels. The increased usage of telematics has further accelerated innovation and provided stakeholders with the insights necessary to address the dynamic nature of driving behavior.
Utilizing Telematics Data for Driving Risk Assessment
Telematics data serves as a cornerstone for developing enriched driving risk models. This type of data allows for the identification of potentially hazardous behaviors and conditions, thus enabling proactive risk management strategies. Some tangible applications include:
- Behavioral Analysis: Telematics data logs specific driving behaviors such as harsh braking, speeding, and distracted driving, allowing institutions to profile risk behaviors and address them proactively.
- Real-Time Monitoring: Offering insurance companies the capability to monitor driving in real-time, telematics data can facilitate dynamic policy adjustments that reflect current behaviors and reduce risk exposure.
- Historical Driving Data: By analyzing long-term driving patterns, insurers can create more personalized policies, fostering customer satisfaction and loyalty.
- Operational Efficiency: Fleet managers use telematics insights to optimize route planning, reducing fuel consumption and operational costs.
- Advanced Predictive Modeling: By complementing historical crash data with telematics, companies can refine predictive models, setting aside generic models for more precise and actionable predictions.
With its dynamic approach, telematics data offers a significant advantage over traditional data sources, rendering it indispensable in contemporary driving risk analysis.
Auto Accident Data
Auto accident data, collected through myriad sources, forms an essential part of risk assessment in the driving domain. Historically, accident data came from police reports, insurance claims, and administrative records, which were often fragmented and lacking real-time availability. Furthermore, the granularity and comprehensiveness necessary for informed decision-making were insufficient by today's standards.
Advancements in data capture technology, such as GPS tagging and mobile app reporting, have improved the scope and accuracy of accident data. Platforms collect extensive accident-related data, from collision severity to liability assessments and root cause analyses, rendering accident data datasets more valuable than ever before.
Insurtech companies, vehicle manufacturers, and policy developers have traditionally relied upon this type of data to inform critical decisions, from establishing liability to developing safety enhancements. The demand for detailed, accurate, and readily available accident data continues to expand, driving innovation and improvements in capture methods, including video analytics and forensic telematics reports.
Application of Auto Accident Data in Risk Models
Incorporating auto accident data into risk models is vital to producing high-precision risk assessments, a capability that serves various stakeholders. Some applications include:
- Liability Assessment: Detailed accident data supports accurate fault determination and liability assignments, crucial for legal resolutions and insurance adjustments.
- Root Cause Analysis: Investigating common factors leading to collisions, datasets offer insights into patterns that can be targeted for prevention strategies.
- Enhanced Safety Features: Vehicle manufacturers can develop advanced safety measures by studying accident trends, thus reducing future risk incidences.
- Insurance Premium Optimization: Insurers can accurately categorize risk categories, ensuring fair premium assessments grounded in comprehensive accident data analysis.
- Strategic Urban Planning: Accident data helps urban planners identify problematic roads and intersections, enhancing traffic management systems and infrastructure planning.
By combining actionable insights derived from auto accident data with telematics, organizations can elevate their risk management capabilities dramatically.
Transportation Data
Transportation data encompasses a wide range of metrics related to vehicle movements, traffic patterns, passenger flows, and travel behaviors on road networks. This data has long been vital to organizations focused on understanding and optimizing transportation systems, traditionally captured through surveys, roadside counts, and travel diaries.
The advent of digitalization within transportation systems has created substantial datasets far richer than their predecessors. Leveraging smartphones, GPS systems, and IoT devices, transportation data today offers an unprecedented depth of insight into how and why vehicles move as they do in urban and rural landscapes.
This data category is relevant to a myriad of sectors, including logistics, government planning, environmental studies, and automotive industries. As data collection methods advance, the acceleration of data in this category is profound, leading to transformative insights and refined transportation strategies.
Leveraging Transportation Data for Risk Analysis
Transportation data interlaces with telematics and traffic data to provide more holistic views of the driving domain, addressing risk analysis needs through applications such as:
- Traffic Flow Analysis: Understanding congestion patterns and traffic volume helps route optimization programs reduce accidents associated with traffic stress.
- Driver Behavior Studies: Data on vehicle habits and preferences supports behavior modification initiatives, promoting safer driving practices.
- Route Risk Mapping: Transportation data highlights roads prone to accidents, offering guidance for infrastructure improvements and risk-reducing strategies.
- Insurance Underwriting: As insurers evaluate geographic risk factors, transportation data informs policy assessments with location-driven insights.
- Environmental Impact Studies: Data helps assess emissions and promote policies that decrease environmental risks associated with urban transport systems.
Much like telematics and accident data, transportation data amplifies the ability to perform data-driven driving risk assessments, equipping stakeholders with the information necessary for informed and timely decisions.
Conclusion
In our digital era, the role of data in understanding and interpreting driving risks has shifted from supplementary to foundational. The availability of diverse types of external data facilitates an elevated approach to analyzing driving patterns, accident risks, and environmental impacts, enabling the development of highly sophisticated models.
As businesses and organizations become increasingly data-driven, the ability to synthesize insights from vast datasets becomes a competitive advantage. The ability to align driving strategies with precise predictive analytics translates into optimized efficiencies and minimized risks, a crucial consideration for all driving-related stakeholders.
The growing trend toward data monetization reflects its burgeoning value, as entities seek to harness data potential for economic gain. This demand fuels innovation and diversification in data offerings, setting the stage for a future where data-driven decision-making shapes entire industries.
Looking ahead, the possibilities we imagine today may only scratch the surface. From transformative vehicular technologies to real-time responsive insurance policies, future data types might include bi-directional insights capturing driver wellness and environmental conditions.
As we approach this future, organizations that integrate telematics, accident, and transportation data effectively will not only understand but anticipate and mitigate driving risks, transforming them into opportunities for growth and innovation.
For those seeking to discover training data and elevate driving risk strategies through AI and machine learning, understanding the rich landscape of telematics data is imperative. Companies today must strive to harness the ever-expanding universe of data to pave the way for safer, smarter, and more sustainable driving futures.
Appendix: Industry Applications and Future Prospects
The collection and application of telematics data offer tangible benefits across industries, creating a ripple effect with potential improvements in safety, efficiency, and customer experience. Among the leading sectors benefiting from these data-driven insights are insurance companies, fleet operators, automotive manufacturers, and government agencies.
The insurance industry, for example, transforms these insights into tailored products and services, leveraging telematics-based data to build more accurate pricing models and even innovative pay-as-you-drive insurance solutions. This adaptability is further underscored by the growing necessity of personalizing policies to satisfy customer expectations.
Fleet managers have continuously harnessed the power of telematics to optimize resource allocation, improve vehicle maintenance routines, and measure driver performance. These capabilities reduce operational costs while ensuring compliance and enhancing fleet-wide safety standards.
Automotive manufacturers leverage insights from accident and transportation data to drive product development and innovate vehicular technologies. By identifying and analyzing safety trends, these manufacturers are empowered to incorporate advanced safety features and pioneering autonomous vehicle technologies.
Governments and municipal agencies utilize transportation and accident data insights to inform urban planning, from infrastructure improvements to enhanced public safety measures. The potential to predict and address congestion hotspots results in streamlined traffic flows and potentially saved lives.
Looking toward the future, Artificial Intelligence (AI) promises to unveil untapped opportunities within historical datasets, providing refined forecasts and actionable insights to stakeholders. By tapping into deep learning capabilities, businesses and governments alike can unlock the full potential of expansive datasets.
The road ahead, driven by data, requires innovation and the willingness to explore, adopt, and leverage the most cutting-edge datasets. From telematics to accident data, and beyond, the journey of discovery and application reshapes not only the way we drive and insure but also how we build the world around us.