Unlocking Insights from Brand Sales Data Across Product Categories
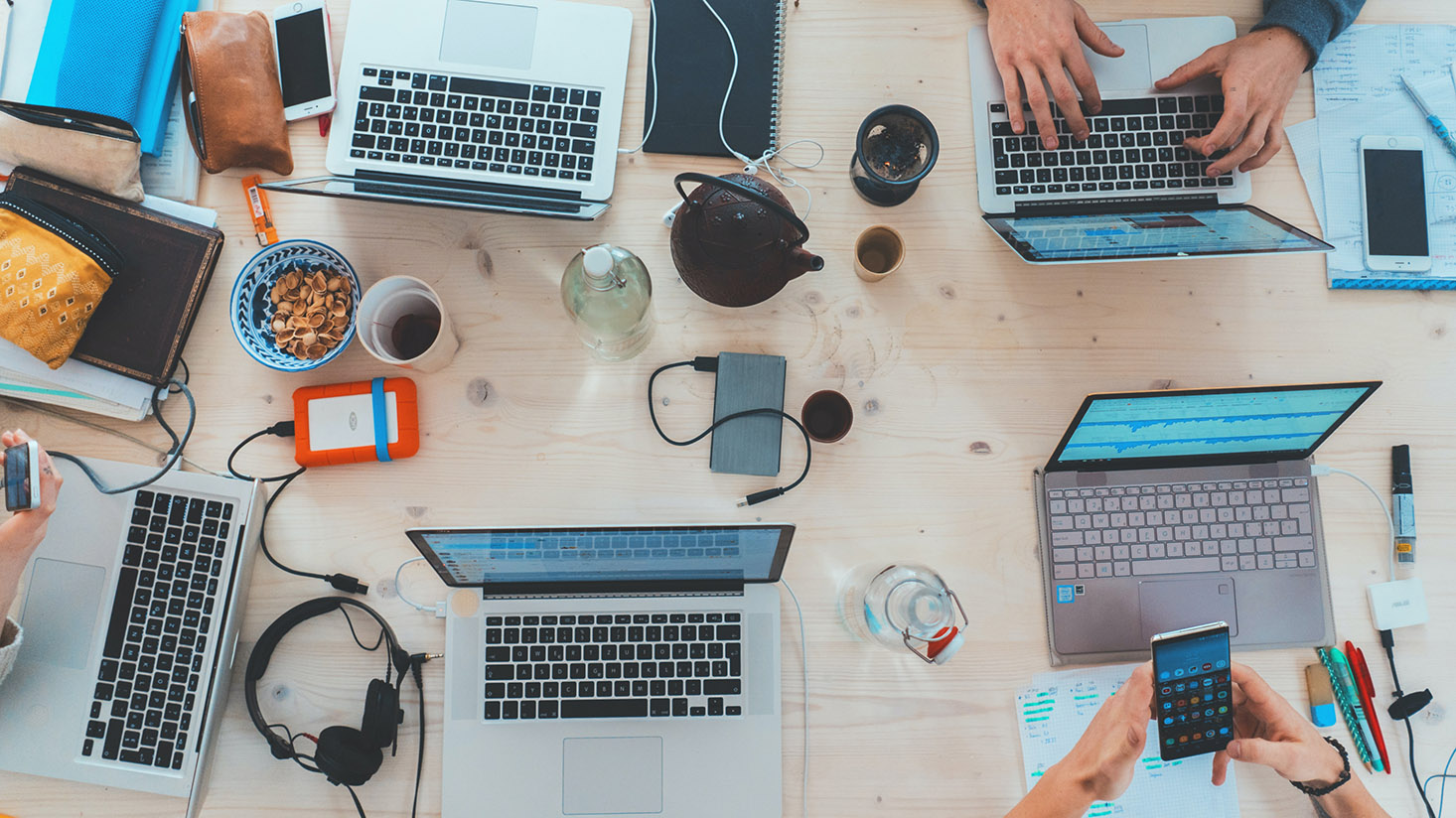
Introduction
In the modern landscape of consumer packaged goods (CPG), understanding brand sales by product category is crucial for businesses aiming to stay competitive. Historically, gaining these insights was challenging, with firms relying on rudimentary methods such as anecdotal evidence, limited survey data, and manual ledger records. Such methods often led to delayed and, at times, inaccurate interpretations of market trends. In a time when data was scarce, business leaders often had to wait weeks or even months to decipher even minor changes in sales patterns, leaving them in the dark about real-time consumer preferences.
The landscape began to shift with the advent of technological advancements. The introduction of sensors and connected devices offered new ways to collect and analyze data. Suddenly, every interaction, every purchase, and every consumer feedback became a recordable event—stored in ever-expanding databases. This proliferation of technology into countless processes laid the foundation for the vast data ecosystem we navigate today. With this, the power to understand market dynamics transitioned from a reactive to a proactive approach.
Importantly, the evolution of external data has played a significant role in this transformation. It allows businesses to track changes in consumer behavior, detect emerging trends, and respond with agility. In today's data-driven world, understanding sales by product type and category can be accomplished with unprecedented precision and speed. This ability to parse voluminous datasets for actionable insights marks a new era in strategic planning.
The information age has made it critically important to have timely and accurate data. Businesses are no longer confined to historical reviews; instead, they can monitor real-time data streams, thus making more informed decisions faster than ever. The need for rapid adaptability underscores the business imperative of aligning with dynamic market conditions to maximize opportunities and mitigate risks.
In this article, we explore how evolving data sources can illuminate sales patterns across different product categories. We will delve into specific data types and their roles in enriching businesses' understanding and decision-making capabilities related to brand sales.
Through comprehensive analysis of various categories of data, businesses can uncover insights that were previously unreachable. This exploration will showcase the significance of Point of Sale, Transaction, and Marketing Intelligence data in deciphering sales by product category, emphasizing the pivotal role of data in shaping business success.
Point of Sale Data
Point of Sale (POS) data has revolutionized the way businesses understand retail environments and consumer interactions. Historically, POS data was limited to basic transaction records, offering insight into what was sold but not necessarily capturing the nuanced details that are critical for thorough analysis. As POS systems have evolved, they have become more than just a means to finalize a transaction. Today, they are powerful data collection tools providing rich insights into sales patterns across diverse product categories.
Enhanced POS data includes information such as product identifier codes, transaction timestamps, and even data on customer demographics. Industries such as apparel, footwear, and everyday consumer goods have increasingly leveraged these insights to finetune their inventory management, pricing strategies, and marketing efforts. The growing sophistication of POS systems means that businesses can now access detailed sales data from thousands of store locations and online channels.
Technology advancements allowed POS data to become more actionable. The integration of advanced analytics into POS platforms enables businesses to identify trends, forecast demand, and implement real-time adjustments to their business strategies. The accelerating volume of POS data ensures that businesses have the information they need to develop tailored strategies for each product category.
Examples of utilizing POS data include:
- Trend Analysis: Retailers can use POS data to track which product categories are gaining or losing popularity over time, enabling them to focus on fast-moving items.
- Inventory Optimization: By analyzing sales velocity and turnover rates, businesses can maintain optimal inventory levels, minimizing excess stock and reducing costs.
- Pricing Strategies: Understanding consumer purchasing patterns helps brands adjust pricing strategies to maximize profits without sacrificing customer retention.
- Customer Segmentation: POS data allows for better segmentation based on purchase history, enabling tailored promotions and advertising campaigns.
- Cross-selling Opportunities: By analyzing complimentary product purchases, retailers can develop effective cross-promotion strategies to increase average transaction values.
These examples highlight the invaluable role POS data plays in shaping strategic decisions across varied industries. Its capacity to offer detailed, actionable insights cultivates a more informed approach to managing sales by product category.
Transaction Data
Transaction data has emerged as a pivotal component of understanding consumer behavior in the retail sector. Traditionally, transaction data was viewed as a byproduct of business activities, but its application has expanded exponentially with the rise of digital commerce. Now, it plays a central role in providing granular insights into brand sales.
Transaction data encompasses a wide array of insights derived from both online and offline purchases. Retailers and eCommerce platforms capture details such as product variety, purchase frequency, geographical distribution, and consumer demographics. Collectively, these data points help decode complex purchase journeys and consumer loyalty patterns.
The technological leap that brought transaction data to the forefront includes advancements in eCommerce platforms, payment gateways, and data analytics. These innovations allow businesses to extract detailed, real-time insights from millions of buyer transactions, tracking patterns over time and across different sales channels. Such data is indispensable for enhancing customer experience and competitive positioning.
Specifically, transaction data can provide businesses with the following insights:
- Multi-Channel Sales Tracking: Businesses can monitor how different sales channels—such as in-store, online, and mobile—perform, helping allocate resources effectively.
- Real-Time Demand Forecasting: Predictive analytics tools analyze transaction data to forecast demand trends, aiding in inventory planning and logistics.
- Customer Retention Strategies: Insights into repeat purchase behavior help develop personalized offers and loyalty programs to enhance customer retention.
- Competitive Benchmarking: By analyzing competitor pricing and sales patterns, businesses can fine-tune their strategies to capture market share.
- Product Lifecycle Management: Transaction data helps identify the lifecycle stages of different products, informing strategies for product launches and phase-outs.
By tapping into the rich vein of transaction data, companies can derive critical insights into sales by product category, dramatically improving decision-making processes.
Marketing Intelligence Data
Marketing intelligence data has traditionally been utilized to inform broader advertising and branding strategies, but its integration into sales analysis has unlocked substantial value. Marketing intelligence encompasses data sourced from varied channels, offering insights into competitor strategies, market dynamics, and consumer sentiments.
This data type has grown in relevance with the proliferation of digital marketing channels and consumer touchpoints. By analyzing sales campaigns, consumer feedback, and digital engagement metrics, businesses can obtain a comprehensive picture of their market position and consumer preferences. As more industries embrace data-driven marketing, the role of marketing intelligence in sales analysis is paramount.
Advancements in digital tools, data analytics solutions, and AI have allowed marketing intelligence to transcend its traditional boundaries, making it an invaluable tool in dissecting sales by product category. Companies now apply marketing intelligence data to drive personalization and engagement with unprecedented precision.
Marketing intelligence data can be leveraged in the following ways:
- Competitive Analysis: Understanding competitors' strategies provides benchmarks for positioning, pricing, and promotional efforts.
- Customer Acquisition: Marketing intelligence reveals which strategies effectively engage new customers, optimizing conversion rates.
- Content Personalization: By understanding consumer sentiment and engagement trends, businesses can tailor marketing content to specific audiences.
- Brand Performance: Tracking the effectiveness of marketing campaigns helps optimize future campaigns and maximize return on investment.
- Market Segment Analysis: Insights into different consumer segments enable businesses to create targeted marketing strategies, enhancing brand reach and awareness.
As companies continue to integrate marketing intelligence into their sales strategies, they find themselves better equipped to navigate competitive markets and grasp opportunities for revenue growth.
Conclusion
In conclusion, understanding brand sales by product type and category is an evermore feasible task with the abundance of available data types. The integration of Point of Sale, Transaction, and Marketing Intelligence data enriches the analytical landscape, transforming how businesses approach strategic decision-making. As organizations strive to become more AI-driven and data-centric, leveraging these rich datasets offers unparalleled insights into consumer behavior.
The significance of data continues to grow as companies aim to monetize useful information that has been generated over years of operations. This trajectory is reshaping business models, where data-driven decisions anchor success.
In light of these changes, organizations must adopt a robust data discovery strategy. As explored in this article, accessing valuable insights from various data sources enables businesses to remain agile and responsive to market shifts.
Furthermore, as companies continue to innovate, we can anticipate the emergence of new data forms that offer even deeper insights into market trends and consumer behavior. For example, real-time inventory analytics, augmented reality shopping experiences, and even more sophisticated consumer sentiment analysis will provide a competitive edge for those who adopt early.
Ultimately, successful adaptation to this data-rich environment requires a shift in mindset where every data point is considered an opportunity for refined decision-making. A future where data is seamlessly woven into all facets of strategic planning is not just expected but necessary for enterprises to thrive.
The successful monetization of valuable data resources will drive future opportunities, underscoring the ongoing quest for transparency and competitiveness in today's data-intensive markets.
Appendix
The potential beneficiaries of robust brand sales data are vast, spanning across numerous industries and roles. Investors, consultants, marketing specialists, product managers, and retailers frequently rely on these insights for improved decision-making.
Investors find brand sales data crucial as it reveals the health and trajectory of companies. It offers clarity on growth patterns and market penetration, informing investment strategies. This data helps identify emerging brand strengths and potential risk factors that can significantly impact portfolio performance.
Consultants employ data analytics to provide actionable insights that guide their clients in reshaping business strategies, optimizing supply chains, and repositioning brands within the market. In an increasingly competitive landscape, consultants who leverage comprehensive sales data can offer value-added services that deliver measurable impact.
Insurance companies utilize brand sales data to assess risks and craft policies that align with market trends. An accurate understanding of sales trends allows for precise risk assessments and premium adjustments, providing enhanced services to their clients.
As for the future, the use of AI and machine learning continues to unlock the hidden potential within decades-old datasets, enabling businesses to parse through complex historical patterns and make informed predictions. From analyzing modern government filings to mining consumer data, AI is poised to elevate the strategic application of brand sales insights.
Despite the strides made in data utilization, there remains untapped potential inside countless digital archives. AI-driven data discovery pathways will illuminate formulating strategic foresight and driving change in industries reliant on comprehensive sales data.