Unlocking Insights into Auto and Truck Collision Claims with Comprehensive Data
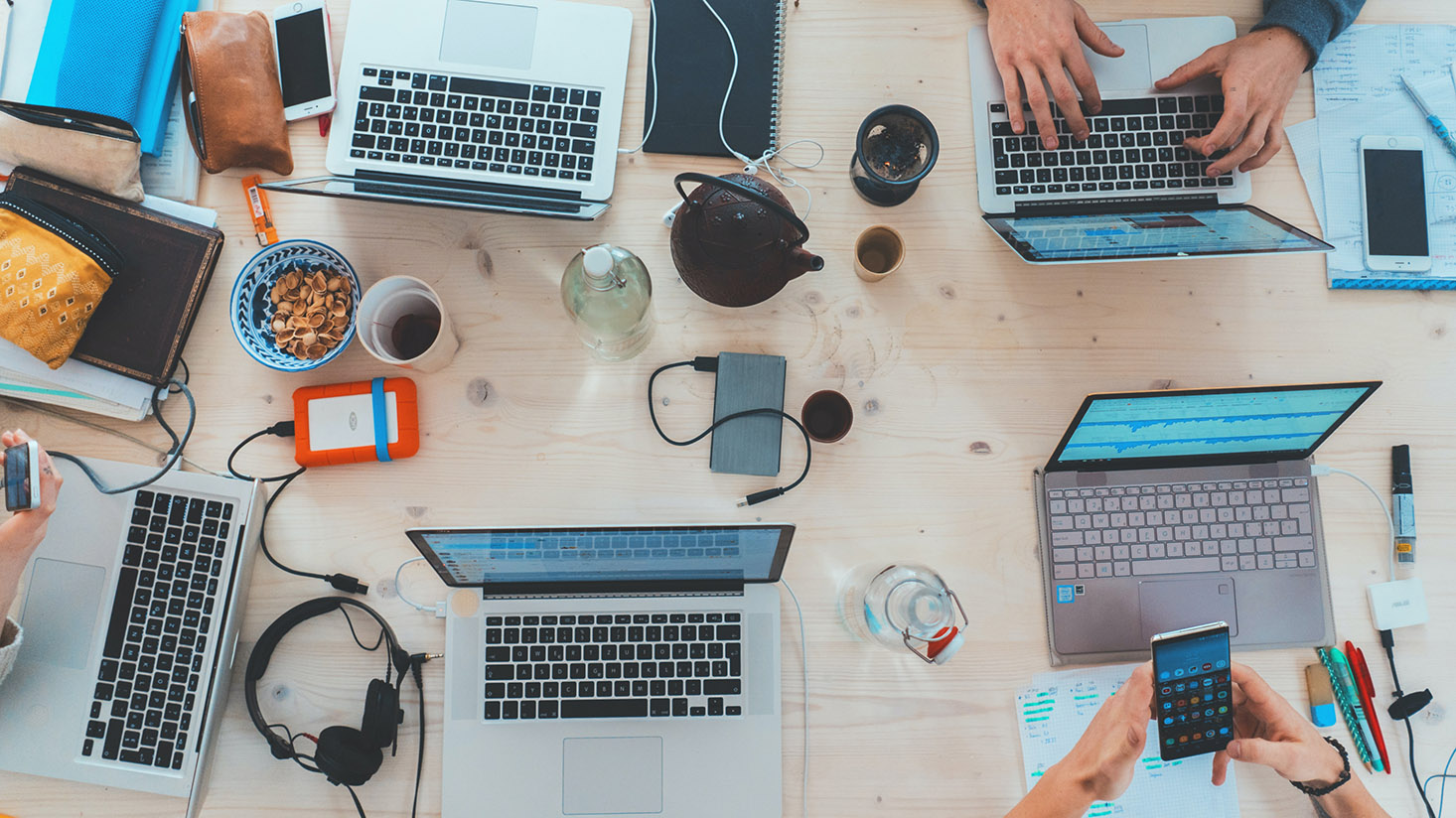
Introduction
The world of auto and truck collision claims has long been shrouded in complexity, with businesses and individuals struggling to grasp the intricacies involved. Historically, understanding these claims necessitated painstakingly slow processes, where insurance companies, automakers, and other stakeholders were left waiting for definitive answers. Before the advent of substantial data collection methods, decision-makers relied on anecdotal evidence and rudimentary accident reports to piece together the necessary information.
In earlier times, the lack of real-time data meant that firms often depended on periodic surveys or simplistic paper records that were prone to errors and inefficiencies. Airing television commercials and relying on paper documentation were among the few techniques that companies resorted to in hopes of forecasting trends or understanding patterns of claims. This method, while somewhat useful, was limited by the time it took to gather information and did not allow for proactive measures.
However, the rise of the internet and connected devices has revolutionized the way we access and analyze information. The proliferation of advanced sensors in vehicles, coupled with the increasing prevalence of smartphones and GPS technology, has enabled real-time data collection that was previously unimaginable. This digital transformation has opened new avenues for collecting valuable data on auto and truck collision claims, offering comprehensive insights to businesses and consumers.
By leveraging modern data-driven approaches, businesses can now gain unprecedented access to detailed claims information, providing insights necessary for predicting trends and adjusting strategies accordingly. The transition from outdated methods to dynamic data analysis allows firms to make informed decisions faster and with greater accuracy, enabling them to manage risks and improve operational efficiency.
The emergence of rich data sources not only helps reduce costs but also enhances the digital experience for users, aligning with the growing need for transparency and instant gratification. Today, companies can track external data like never before, enabling them to understand patterns across various segments of the industry, including accident frequency, severity, and root causes, all in real time.
Insurance Company Data
One of the primary sources of detailed information on auto and truck collision claims comes from insurance companies. Historically, these firms have collected a vast amount of data, although much of it remained underutilized due to technological and analytical constraints. However, as technology evolved, insurance companies have embraced digital innovation, paving the way for richer datasets that provide deeper insights into collision claims.
Insurance data often includes factors such as the vehicle's make, model, and year, along with claim details, loss causes, and financial metrics like loss payments and expenses. This treasure trove of information encompasses a range of metrics vital for understanding risk factors and devising effective loss prevention strategies. Industries like automotive manufacturing, repair, and insurance management have historically relied on this data to optimize their operations and gain competitive advantages.
Technological advances have significantly accelerated the collection and analysis of insurance data. With the introduction of advanced telematics and machine learning, companies can now sift through massive datasets to uncover patterns and associations that would have otherwise remained hidden. This capability enhances risk prediction models, facilitating proactive interventions that mitigate losses and boost profitability.
Insurance data can reveal significant insights, including:
- Risk Assessment: The data aids insurers in evaluating the likelihood of future claims, allowing them to tailor their coverage options accordingly.
- Loss Cause Analysis: By diving into the specifics of each claim, companies gain an understanding of common accident triggers and develop targeted safety programs.
- Geographical Insights: The ability to link claims data with geographical locations helps identify hotspots for accidents or fraud.
- Vehicle Trends: Insights into vehicle types involved in most claims can guide design improvements and inform manufacturing strategies.
- Financial Insights: Understanding the breakdown of loss payments and expenses aids in budgeting and financial planning.
Overall, insurance company data serves as a lynchpin in the broader ecosystem of auto and truck collision information, providing the raw material needed to unlock meaningful, actionable insights.
Industrial Data
Another pivotal data category in exploring auto and truck collision claims is industrial data, particularly datasets focusing on vehicular crash events. While such data doesn't cover every aspect of a claim, it offers insight into the frequency and circumstances surrounding accidents. Traditionally, industrial data providers have focused on logistics and vehicular statistics, offering data that facilitates the understanding of incident prevalence.
These crash event datasets are invaluable for professionals needing a grasp on vehicular safety aspects and broader trends in accident occurrence. The tools for tracking industrial data have evolved alongside the data landscape, with technological advancements like AI and IoT sensors playing a crucial role in enriching the available datasets.
With an accelerating influx of information due mainly to real-time data feeds, industrial datasets have become indispensable to a variety of sectors. They enable analyses that drive safety protocols and inform maintenance schedules, underscoring heavy reliance from industries such as manufacturing, fleet management, and logistics planning.
Industrially-sourced data provides a series of key insights:
- Accident Frequency: Understanding the number of related incidents to prioritize preventive measures.
- Incident Context: Offering details about the situations in which accidents occur, enabling better preparedness and response planning.
- Collision Patterns: Detecting recurring scenarios that lead to accidents can drive systemic adjustments and training.
- Safety Enhancements: Data-driven improvements in safety can lower the likelihood of future claims.
- Operational Planning: Logistical businesses can adjust routes and schedules based on historical crash events.
Overall, industrial data represents a crucial dataset that complements traditional insurance data, providing additional dimensions that can enhance understanding and help mitigate collision-related risks.
Conclusion
In an ever-evolving landscape, the role of data in understanding auto and truck collision claims cannot be understated. As businesses increasingly integrate various categories of data into their strategic efforts, the advantages of harnessing detailed datasets become evident. The ability to gather and analyze information in real-time equips businesses to respond quickly and effectively, transforming decision-making processes and enhancing operational resilience.
Data-driven strategies not only empower firms to anticipate potential risks but also pave the way for improved risk management and mitigation plans. The continuous evolution of data analytics promises a future where organizations become more adept at leveraging the wealth of information available to them, ensuring better outcomes and more sustainable results.
As organizations aim to become more data-centric, they increasingly recognize that data monetization is a vital aspect of modern business strategy. Efforts to monetize data ensure that the valuable insights derived are used to their full potential. In this context, auto and truck collision claims offer a compelling example of the importance of this approach.
Looking forward, the types of data that companies may seek to utilize will likely expand, as technologies like Artificial Intelligence continue to develop and advanced sensors become more accessible. These innovations could yield entirely new data categories, offering even deeper insights into the nature of accidents and vehicle safety.
Organizations that successfully embrace a data-driven culture will be best positioned to thrive in the rapidly-changing automotive landscape. By utilizing varied data resources, they can ensure comprehensive insights that support their strategic goals and drive sustainable competitive advantages.
Appendix
The diverse roles and industries that can leverage data on auto and truck collision claims are numerous and widespread. Insurance companies have long been a natural fit, relying on this data to refine their claims processing and enhance risk assessment. As data sources improve, their ability to fine-tune policies and offerings likewise strengthens, leading to more personalized customer experiences.
Consultants in the automotive or logistics industries use this wealth of information to advise on best practices, from optimizing supply chains to advising on road safety protocols. They provide invaluable expertise to businesses seeking to reduce claim frequency and severity, enabling clients to incorporate data-driven strategies effectively.
Market researchers benefit immensely by using collision claims data to unpack industry trends, aiding them in identifying emerging areas of interest and opportunity. By analyzing accident patterns, they offer insights that inform product development and service launches, underpinning strategic growth initiatives.
With the growing importance of training data, industries coupled with AI providers find themselves reliant on this information for developing advanced risk prediction models. By unearthing trends hidden within massive datasets, they design sophisticated tools that bring value to all industry stakeholders.
Insurance and reinsurance sectors utilize rich claims data within their actuarial processes, applying sophisticated models to better gauge risk exposures and drive profitable policy enhancements. Improved data accuracy leads to more precise pricing models, optimizing their offerings while aligning with evolving consumer expectations.
In the future, greater emphasis on automated systems and predictive analytics within the realm of claims and risk management suggests a likely approach whereby AI-driven solutions will unlock elusive patterns, ultimately revolutionizing the industry's ability to preempt and mitigate risks effectively.