Unlocking Insights into US Traffic Patterns with Geolocation and Automotive Data
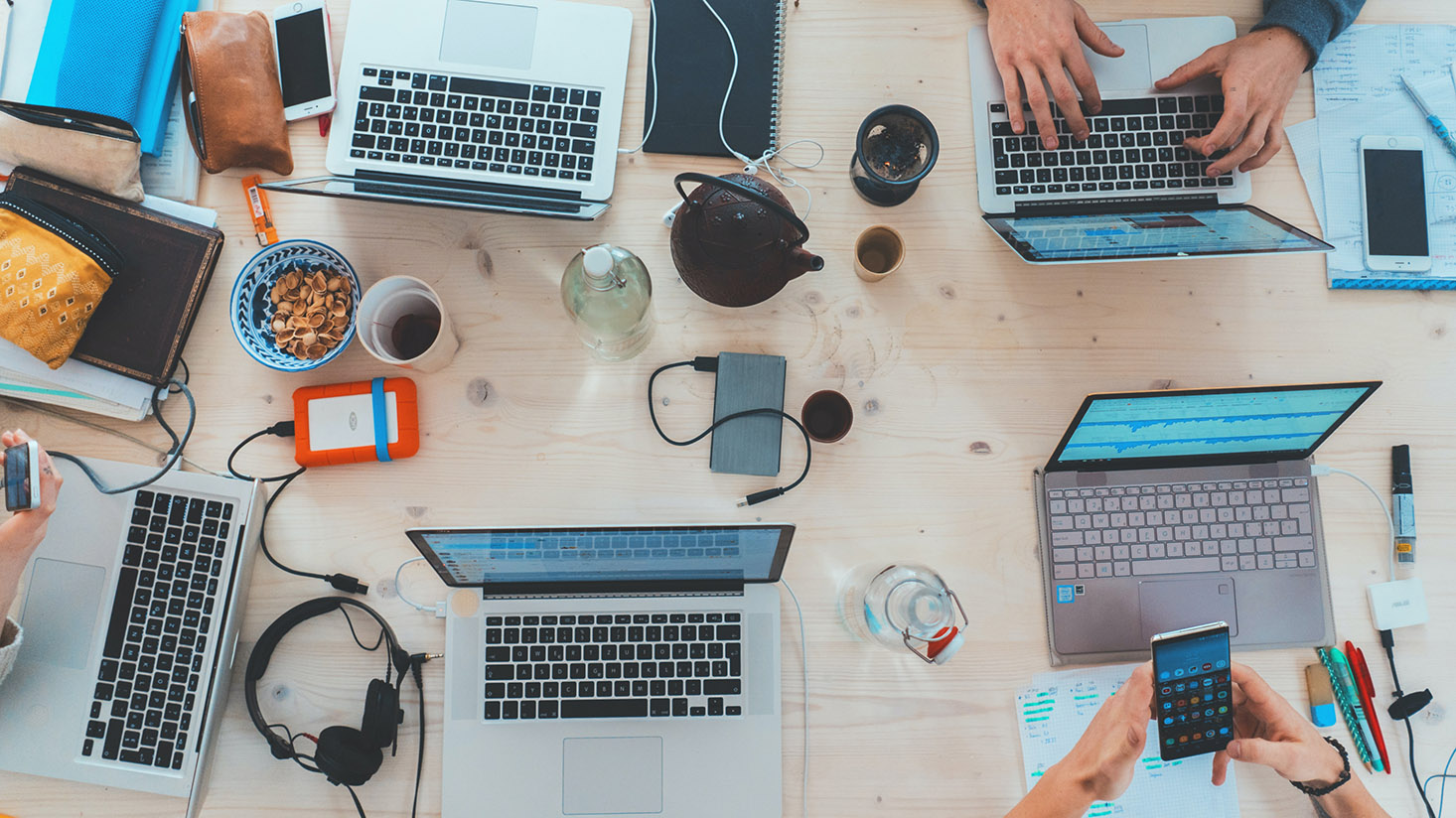
Understanding US car traffic and accident patterns has historically been an exercise in patience, guesswork, and aging technology. For decades, transportation analysts relied on antiquated methods such as manual traffic counting and one-size-fits-all accident reports that lacked granularity. Tools like pneumatic road tubes and handheld clickers were standard fare, capturing traffic volumes and patterns in snapshots, rather than in continuous streams.
Before the widespread use of data, understanding traffic and accidents mainly depended on observational reports which were both time-consuming and expensive, often limited to small geographic areas. Insights gathered through these methods were delayed, leaving stakeholders in the dark about real-time conditions. Aggregated annual reports, infrequent surveys, and public records served as the cornerstone of infrastructure planning. This outdated approach meant decision-makers had to wait weeks or even months for accurate data summaries to be compiled and disseminated.
With the advent of the internet and increasingly connected devices, the landscape for gathering insights into US traffic and road incidents changed dramatically. The proliferation of smartphones, in-vehicle sensors, and GPS technologies fueled the rise of Big Data, offering an unprecedented look into traffic flows and accident locations. This digital influx enabled the collection and analysis of massive data sets in real time, erasing the delays of earlier methods.
Today, understanding traffic congestion and road safety issues is essential not only for urban planners and government agencies but also for businesses in transportation, insurance, and logistics sectors. With the ability to access detailed real-time data, stakeholders are better equipped to respond swiftly to evolving conditions on America's highways and byways. These advancements have been critical in reducing response times to accidents and managing traffic flow effectively.
Data is increasingly crucial for traffic management, with its capacity to shine a light on previously unseen trends and patterns. By analyzing data points from various connected devices, traffic wardens to business strategists can forecast congestion, improve route planning, and even predict areas prone to accidents. For many firms, having timely and accurate traffic data can mean the difference between success and failure in managing logistical constraints and safety challenges.
In this modern context, the importance of data cannot be overstated. As businesses and government bodies aspire to understand, predict, and respond to traffic dynamics in real time, they can leverage a variety of categories of data that are discoverable through platforms offering large-scale traffic datasets. Below, we will explore the diverse types of data that are crucial in comprehending US traffic and accident trends.
Geolocation Data
Geolocation data has become an indispensable tool for unraveling traffic patterns and accident hotspots across the United States. This dataset primarily consists of the positional information gathered through connected devices, such as GPS-enabled smartphones and in-vehicle navigation systems, providing insights into traffic flow and congestion levels across the nation.
The emergence of geolocation data began in the early 2000s, following technological advancements in GPS technology. These developments paved the way for real-time traffic updates and advanced mapping services that now serve an array of industries beyond transportation, including retail, real estate, and financial services.
Industries such as urban planning, logistics, and automotive rely heavily on geolocation data. Urban planners use it to design smarter cities, logistics companies optimize delivery routes, and automotive companies improve navigation features in vehicles. Over time, the precision and scope of this data have improved significantly, allowing for more accurate and localized insights.
With the current acceleration in data volume from multiple data sources, including crowd-sourced information from driving apps and connected vehicle sensors, geolocation data offers unprecedented granularity in understanding traffic dynamics. Great strides have been made in machine learning algorithms, enhancing the predictive capabilities associated with this data type.
Examples of how geolocation data can be used to understand US traffic patterns include:
- Real-time traffic monitoring: Provides insights into congestion levels, enabling better decision-making for route planning and emergency responses.
- Accident hotspot identification: Identifies areas where accidents frequently occur, assisting in targeted infrastructure improvements.
- Evaluating traffic management policies: Helps assess the effectiveness of traffic control measures, such as new traffic signals or lane configurations.
- Urban planning: Aids in designing efficient road networks by analyzing traffic flow patterns.
- Improving public transport routes: Supports optimization of public transport routes by understanding passenger travel patterns.
Automotive Data
Automotive data offers a deeper glimpse into vehicle operations and driver behaviors on US roads. This type of data encompasses a broad range of information sourced directly from vehicles, including speed, location, acceleration, braking patterns, and engine diagnostics.
Historically, automotive data collection was limited to basic mechanical diagnostics accessible only through workshops and specialized equipment. However, advancements in telematics systems and the integration of IoT technologies in vehicles have transformed the scope and accessibility of this data.
Key players in the automotive industry, such as car manufacturers, fleet operators, and insurance companies, have embraced this data to enhance vehicle performance, manage fleets more effectively, and calculate insurance premiums based on driving behaviors.
The pace at which automotive data is being generated is accelerating due to an increase in connected vehicles and smart infrastructure. With every passing mile, vehicles not only transport passengers but also transmit telemetry data that, when aggregated, yields valuable insights into traffic patterns and safety issues.
Specific ways automotive data helps in understanding traffic and safety trends include:
- Fleet management: Optimizes routes and maintenance schedules for commercial fleets, leading to reduced costs and improved safety.
- Insurance analytics: Assists insurers in developing personalized insurance products based on actual driving records and patterns.
- Predictive maintenance: Utilizes engine diagnostics to anticipate potential mechanical failures, preventing breakdowns on busy roads.
- Simulation of traffic scenarios: Provides data for simulating traffic conditions to inform infrastructure planning and development.
- Monitoring and improving driving behaviors: Encourages safer driving by offering feedback on driving patterns such as harsh braking and acceleration.
Transportation Data
Transportation data is a comprehensive dataset that encompasses a wide array of information regarding vehicle movements, road conditions, and driver behaviors. This data type aggregates input from mobile apps, GPS devices, and smart infrastructure, presenting a panoramic view of regional and national traffic trends.
Historically, transportation data was pieced together through a combination of direct observation and intermittent surveying. The technological leap brought forth by cloud computing and sophisticated analytics has allowed for more immediate and precise collection methods.
Roles that have traditionally harnessed transportation data include policymakers, infrastructure planners, and economic analysts. These professionals utilize the dataset to forecast traffic demand, inform construction projects, and conduct cost-benefit analyses that drive investment in public infrastructure.
The explosion of mobile application usage and connected device networks has enriched transportation data, providing deeper insights into both historical trends and emerging traffic phenomena. This has revolutionized how traffic models are crafted and how resources are allocated to combat congestion.
Examples of how transportation data can help in traffic and accident analysis include:
- Enhanced traffic modeling: Supports the development of accurate simulations for predicting traffic congestion and accident likelihood.
- Real-time incident detection: Identifies and reports accidents and congestion, enabling quick incident response.
- Infrastructure optimization: Guides decisions on road repairs and expansions to align with actual usage patterns.
- Public transport planning: Improves scheduling and route planning for buses and trains based on traffic and passenger flow data.
- Environmental impact assessment: Analyzes vehicular emissions and their impact on urban air quality, especially in areas prone to traffic jams.
Consumer Behavior Data
Consumer behavior data, while not immediately obvious as a primary source for traffic information, plays a significant role in understanding traffic dynamics through its insights into destination choices and travel patterns. This dataset includes information gathered from mobile apps, online searches, and point-of-interest analytics.
Gaining traction over the past decade, this data has become essential for industries like retail, hospitality, and real estate that require in-depth knowledge of where and when consumers converge in particular locations.
Consumer behavior data is increasingly being utilized by traffic authorities to plan beyond the scope of simple road layouts, instead looking into the varied and often changing motives behind traffic flows, such as shopping, dining, and event-based travel spikes.
The rapid evolution of digital commerce and geo-targeted marketing has further enriched consumer behavior datasets, providing more precise insights into how and when people move, which correlates with traffic trends.
Applications of consumer behavior data in traffic analysis include:
- Destination trend analysis: Reveals consumer hotspots, aiding traffic management near popular commercial centers.
- Demand-based transport services: Informs the demand for rideshare services and public transport in high footfall areas.
- Traffic origin-destination studies: Enhances understanding of how consumer habits influence travel patterns across regions.
- Retail and event planning: Supports infrastructure planning for major events by forecasting traffic influx.
- Travel behavior profiling: Assists in developing targeted traffic regulations to address specific travel behaviors.
Conclusion
The importance of leveraging diverse data types to understand US car traffic and accident patterns cannot be overstated. In this era where timely and accurate data can transform outcomes, having access to a variety of data sources allows industry leaders to make better-informed decisions, optimize operations, and anticipate future challenges effectively.
As highlighted in this article, Geolocation, Automotive, Transportation, and Consumer Behavior data each contribute uniquely to building a holistic view of traffic dynamics. By integrating these data types, businesses can develop more nuanced strategies to navigate the complex landscape of road traffic and safety.
As organizations evolve towards data-driven decision-making, the role of data discovery will be pivotal. More than ever, businesses are striving not just to access data but to unlock its potential through comprehensive analysis and predictive capabilities offered by emerging technologies like AI.
The future promises further innovations in data types and how organizations might monetize their data. The traffic and accident data sphere is no exception, as corporations realize the potential locked within decades' worth of driving data and burgeoning connected infrastructure.
Speculation arises about the future of data-driven insights, such as the monetization of diverse data types, including integrated mobility data from autonomous vehicles that can offer granular, real-time insights into traffic and accident trends. The potential for extracting even greater value from these data sets lies on the horizon, destined to reshape how traffic and safety issues are approached.
The opportunity to transform today's data into tomorrow's insights is vast, and organizations ready to capitalize on these developments will find themselves ahead of the curve, steering through the complexities of US traffic with unprecedented precision.
Appendix
Various roles and industries stand to gain remarkable insights from the application of data to US traffic and accident analysis. By delving into rich datasets, investors, consultants, insurance companies, and market researchers, among others, can address long-standing industry problems efficiently.
Investors benefit from traffic data by assessing infrastructure investment opportunities. Understanding traffic patterns can help predict potential growth areas, influencing real estate and commercial property investments. Consultants utilize this data to advise municipalities on road improvements and urban planning strategies.
Insurance companies harness the power of detailed accident and traffic data to better assess risk, adjust premiums, and identify fraud. By employing fresh insights into driver behaviors and accident hotspots, they can develop tailored insurance products.
Market researchers analyze consumer behavior and traffic data to gain a comprehensive view of market dynamics. By understanding congestion patterns and consumer movement, they can provide strategic advice on retail locations and marketing initiatives.
As we look to the future, the role of AI in unlocking the potential of historical traffic data and modern government filings is bound to expand. AI's ability to sift through large datasets will uncover hidden patterns and forecast future traffic trends, offering advanced decision-making tools to businesses.
In summary, the data revolution in understanding US traffic and road safety is transforming numerous industries. The integration of advanced analytics and machine learning can offer tangible benefits, translating into enhanced competitiveness and operational excellence across sectors.