Unlocking Insights with Commercial Automobile Analytical Data
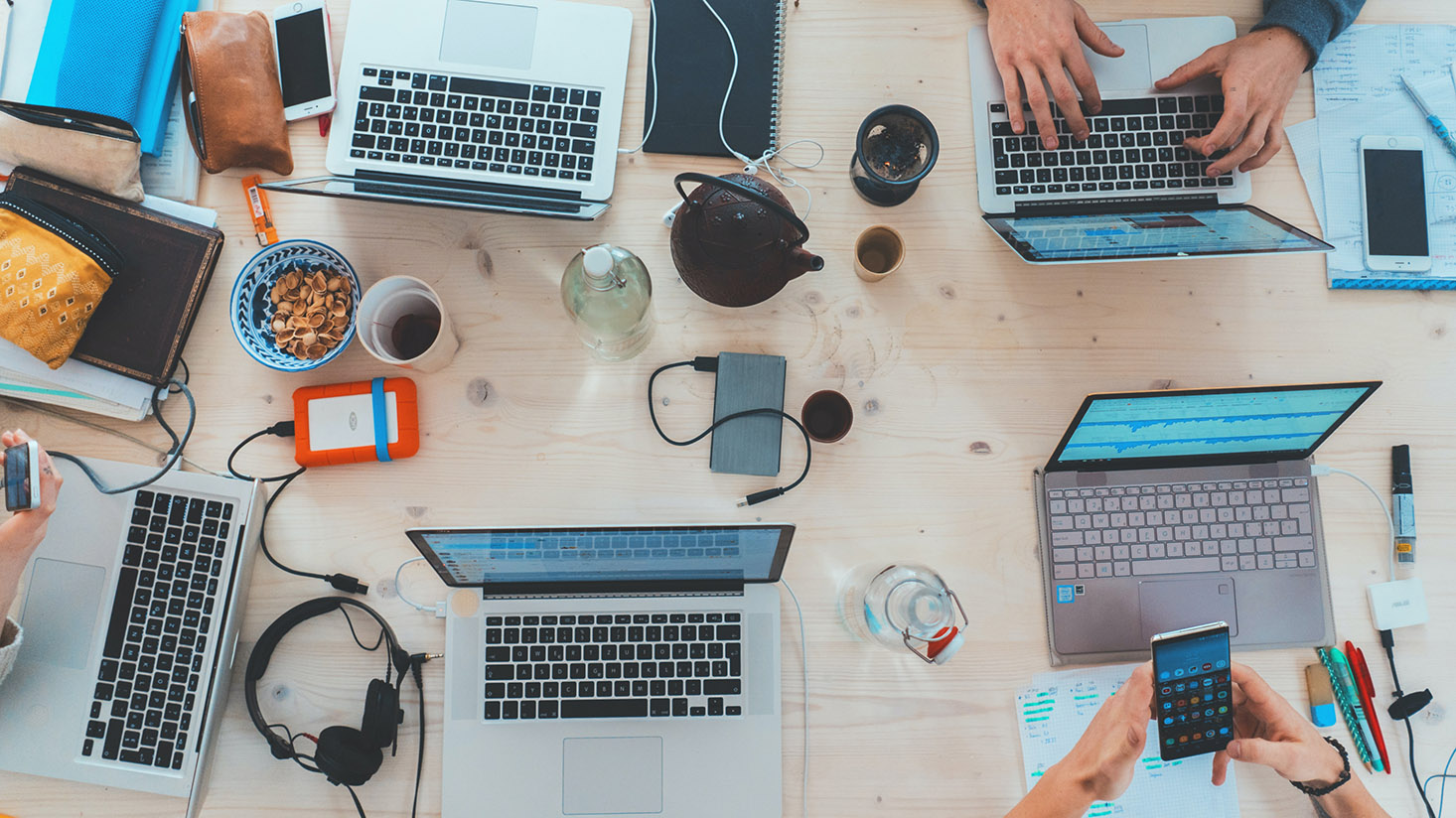
Introduction
The commercial automobile sector has long been a cornerstone of global economic activity, playing an indispensable role in transportation, logistics, and enterprise efficiency. Historically, gaining insights into commercial automobile operations and risks has been shrouded in complexity. Without data, industry stakeholders relied heavily on fragmented, manual processes, where anecdotal evidence and rudimentary assessments guided decision-making.
For decades, industry experts sought to predict and mitigate risks in commercial automobile operations using traditional tools. Methods like driver surveys, paper-based incident reports, and periodic maintenance logs informed risk assessments and operational planning. However, these methods often fell short, leading to time-consuming data collection, dissonance between actual and reported incidents, and delays in comprehending industry trends.
The digital revolution set the stage for unparalleled advancements in data collection, making it possible to capture vast quantities of real-time information. Sensors, telematics, the Internet of Things (IoT), and big data analytics converged to transform commercial automobile data availability. The migration to digital platforms facilitated a paradigm shift, allowing comprehensive datasets to be stored and accessed effortlessly.
Before converging technologies, companies awaited weeks or months for actionable insights, hindering their ability to rapidly adapt to changes. Today, continuous streams of data provide a granular view, enabling stakeholders to harness valuable insights in real-time. This evolution underscores the importance of data in realizing predictive capabilities and adaptive responses.
Businesses are no longer content with retrospective analyses. Now, organizations utilize analytical, external data to empower themselves with instant, informed decision-making. With this data, organizations can track incident trends and anomalies, uncover risk patterns, and intervene proactively, dramatically improving risk management in commercial automobile contexts.
Ultimately, the readiness and precision of data redefine how commercial automobile stakeholders navigate their complex landscape. As venture into this fascinating domain, understanding the intricacies of diverse data categories becomes essential for driving strategy and competitiveness.
Automotive Data
The landscape of Automotive Data has witnessed groundbreaking transformations, offering crucial insights into vehicle operations, safety, and performance. Historically, automotive data was scarce and rudimentary, primarily derived from user-generated logs and reactive records collected during service appointments or post-incident analyses.
Today, automotive data encompasses a wide array of metrics, ranging from safety and compliance to vehicle health diagnostics. Vehicle sensors, telematics, and cloud-based platforms form the backbone of contemporary automotive data, with vehicles themselves becoming complex data sources. These innovations have radically altered the collection, breadth, and application of commercial automotive insights.
The congregate of these data points has proven beneficial across various industries and roles. Automotive manufacturers, insurance providers, fleet owners, and regulatory bodies extensively employ this data for optimizations and predictive analyses, driving enhanced operational efficacy and risk management.
Technology has undoubtedly accelerated the capabilities of automotive data. The integration of artificial intelligence, machine learning, and IoT has made it possible to predict and preempt potential faults, reduce accident risks, and foresee maintenance needs. This real-time analytical depth ensures robust solutions and innovations in publishing and utilizing commercial automobile datasets.
In terms of applications, automotive data enables stakeholders to achieve:
- Predictive Maintenance: By analyzing vehicle health and performance data, fleet managers can proactively schedule maintenance, reducing downtime and prolonging vehicle lifespan.
- Risk Assessment: Telematics and accident data help identify high-risk routes and drivers, aiding in targeted interventions and insurance underwriting.
- Compliance Monitoring: Data facilitates regulatory adherence by tracking vehicle compliance with safety and environmental standards.
- Route Optimization: Data-driven insights streamline fleet routes, enhancing efficiency and reducing fuel consumption.
- Enhanced Safety: Collision and near-miss analyses contribute to better safety protocols and driver training programs.
Risk Data
Risk Data is a linchpin in commercial automobile analytics, pinpointing areas susceptible to high-severity incidents and potential litigations. Historically reliant on delayed and limited claim records, the industry has embraced comprehensive risk datasets powered by predictive analytics and cross-industry benchmarking—a substantial leap forward from mere anecdotal evidence.
Risk data primarily involves claim frequency and severity analyses, clarification of litigation potentials, and predictive modeling through historical trends and claim characteristics. These dimensions provide unparalleled foresight to anticipate and manage complicated scenarios in commercial automobile operations.
With the augmentation of types of data, risk datasets have evolved, facilitating intuitive platforms that offer detailed insights into loss reserves, claim duration, and more. This enables companies to juxtapose their performance against industry benchmarks, flagging or forestalling deviations.
Incorporating real-time risk data allows businesses to react swiftly to emerging threats while actively streamlining claims processing and budgeting. This dynamic capability has proven invaluable to insurance entities, fleet managers, and claims adjusters looking to preemptively address adverse trends.
Here, risk data proves instrumental by:
- Early Detection of High-Severity Claims: Advanced predictive models identify problematic patterns early, allowing for targeted intervention to mitigate costly claims.
- Litigation Potential Recognition: Flagging features in the data signal potential litigations, equipping companies with foresight to limit legal expenditures.
- Industry Benchmarking: Comparative insights empower stakeholders to align their practices with peer and market standards.
- Data-Driven Decision-Making: Customizable dashboards enable filtering and reporting, rendering data actionable for specific business needs.
- Real-Time Data Access: Expedited access to real-time updates informs proactive measures to confront imminent challenges.
Auto Accident Data
The domain of Auto Accident Data has gained prominence due to its direct impact on insurance assessments, legal processes, and fleet management strategies. Before sensors and digital footprints, auto accident documentation depended heavily on human eyewitness testimonies and police reports, often resulting in subjective, incomplete, or delayed accounts.
In contemporary settings, auto accident data is generated by in-vehicle systems, independent collision analyzers, and expert-validated datasets. These include data on collisions, near misses, driving behavior events, and geolocation aspects, as well as analytical models for crash reconstructions and risk root causes.
This revolution in data collection benefits several stakeholder groups, delivering accurate claims settlements, insights into driver behavior, and enhanced road safety initiatives.
The acceleration and precision with which auto accident data can be acquired transforms how entities approach risk mitigation and liability assessments. Deploying advanced cameras, in-cab monitors, and GPS services offers granular insights into vehicular incidents.
Notable uses of auto accident data include:
- Collision Reconstruction: Utilizing accurate video and sensor data facilitates comprehensive crash scene reconstructions.
- Driver Behavior Analysis: Assessing driving patterns to flag risky maneuvers or tendencies aids in driver improvement programs.
- Claims Validation: Authentic datasets avert fraudulent claims, expediting genuine claim processing.
- Performance Benchmarking: Extensive databases of anonymized cases assist in industry-wide analysis of accident trends.
- Geospatial Risk Identification: Identifying accident hotspots informs safety upgrades and policy formulations.
Conclusion
As the commercial automobile industry continues to navigate its complexities, data emerges as a formidable ally, bridging gaps in knowledge and capability. The transformations brought forth by automotive data, risk data, and auto accident data redefine operational strategies and enhance safety standards.
The availability of multifaceted datasets allows businesses to harness comprehensive insights across diverse scenarios, driving informed decision-making. This underscores the vitality of a data-driven culture, positioning organizations to not only respond but foresee, adapt, and innovate.
Organizations increasingly recognize the potential to monetize their data, leveraging proprietary insights accumulated over decades. Such initiatives encourage data accessibility and foster a rich ecosystem of data-driven innovation.
The benefits of external data consumption extend to tangible and strategic outcomes—reducing costs, elevating safety, and championing proactive risk management.
The future of commercial automobile insights rests on the emergence of new data sources and analytical techniques. From sensor-based analytics to open vehicle architectures, emerging tools will continue to enhance prediction accuracy and oversight visibility.
Anticipating future developments, businesses stand to gain by embedding new technologies and data discoveries into their operations. The power of data—ever-evolving and universally consequential—will unlock unprecedented opportunities, curating a resilient and adaptive commercial automobile landscape for decades to come.
Appendix
The dynamic spectrum of commercial automobile data benefits numerous roles and industries. Insurance companies, market researchers, fleet operators, and litigation specialists increasingly unlock data to derive multifaceted insights.
Insurance entities face the perennial challenge of meticulous risk assessment and efficient claims processing. With automotive data analytics, risk identification and mitigation become integral, swiftly advancing proactive approaches in underwriting and claims management.
Fleet managers grapple with untapped efficiencies and productivity metrics. Leveraging IoT-driven vehicle health and route data, managers streamline operational logistics, reducing revenue loss and reinforcing profitability paradigms.
Consultants and market researchers frequently encounter transformative shifts in commercial vehicle utilization patterns. Equipped with data, these professionals unveil predictive insights, allowing for adaptive, tailored market engagement strategies.
Moreover, regulatory individuals are tasked with compliance oversight and policy optimization. By integrating real-time data sources, regulations evolve dynamically, addressing emergent safety challenges and holistic transportation needs.
The future remains brimming with possibilities, especially considering the impact of AI in deciphering latent potential within both historic archives and contemporary filings. As AI permeates the data landscape, commercial automobile sectors will unearth and harness understudied insights, underscoring the indispensability, sophistication, and breadth of data in shaping future operations.