Unlocking Insurance Insights with Comprehensive Credit Score Data
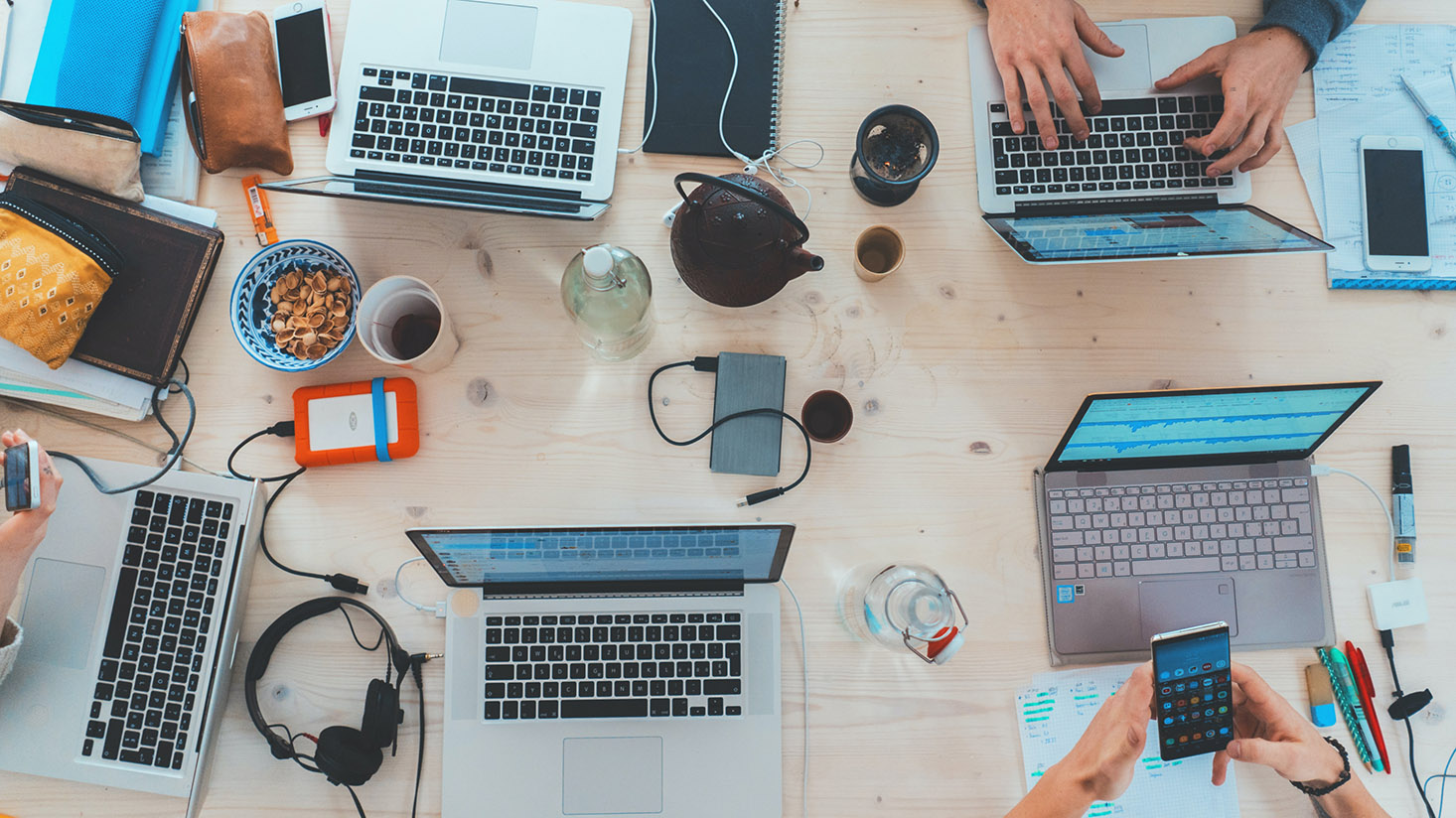
Introduction
In the ever-evolving landscape of the insurance industry, understanding risk is paramount. Historically, firms have struggled to gain precise insights into the factors influencing policyholder risk, particularly in the homeowners insurance domain. Before the digital revolution, insurance companies relied on rudimentary methods such as direct interviews and lengthy consumer surveys to gather data. They often evaluated risk based on observable factors such as the age of the structure or the geographical risk of natural disasters. These methods were time-consuming and often lacked the desired accuracy.
In earlier days, the lack of sophisticated data meant that insurers had to wait weeks, sometimes months, for data to be collated, analyzed, and reported. This delay invariably led to reactive rather than proactive decision-making. With the advent of the internet, sensors, and connected devices, this dynamic has drastically transformed. The proliferation of software capturing every consumer interaction and transaction has contributed vastly to data storage, allowing insurers access to real-time data that was previously unthinkable.
Credit score data is a game-changer in this context. Credit scores, which once were a peripheral consideration, are now recognized as a critical factor in understanding the propensity for future loss both in severity and frequency. As credit score data became more digitized, it provided an enlightening view of consumer behavior and financial health. Insurers can, therefore, leverage this data to tailor their premiums and predict potential risks with greater accuracy.
Historically, the insurance industry operated somewhat in the dark, relying heavily on outdated methods and generalized statistics to shape their models. However, the introduction and integration of specific data types have shone a new light on old challenges. As a result, the insurance industry can now understand changes in consumer behavior and risk in real-time, allowing more fine-tuned policy adjustments.
The importance of data cannot be overstated. As industries become increasingly digital and interconnected, the volume, variety, and velocity of credit information gathering continue to grow. Consumer credit behaviors, such as mortgage payments and other lending behaviors, are being tracked meticulously, providing insurers with a clearer picture of a policyholder's risk profile. This transition marks a shift towards data-driven decision-making processes, enabling companies to offer competitive pricing, mitigate risks, and improve overall customer satisfaction.
In essence, credit score data is now a tool not only for understanding consumer behavior but also for predicting and evaluating potential risks. Insurers and related stakeholders need to unlock this potential and leverage it as part of the evolving landscape of data analytics in insurance. The journey is no longer just about gathering data but interpreting it with precision and speed to cultivate a more robust, informed approach to risk and pricing models.
Consumer Behavior Data
Consumer behavior data represents a potent type of external data source that insurance companies can harness to uncover hidden insights about their customers. Historically, credit report information provided a foundational understanding of a consumer's purchasing behavior, debt levels, and overall creditworthiness. These insights were gathered through cumbersome processes requiring authorization, which limited their use in broad-scale analytics.
With the advancement of data technologies, consumer behavior data has become more comprehensive and nuanced. Modern datasets such as those from the Analytic Dataset 3.0 offer rich, unbiased samples that encapsulate monthly consumer credit performance, helping analysts assess intricate behavior patterns over extended periods. This kind of data has an extensive application scope, being instrumental in areas like whole loan trading, securitization, and regulatory compliance.
The insurance sector can gain proprietary insights into a household’s likely claim tendencies or potential financial stability by integrating datasets encompassing vast arrays of financial attributes beyond just credit scores. For instance, Experian offers data featuring thousands of consumer attributes at both individual and household levels, enabling more personalized and precise modeling for pricing and risk assessment.
When leveraging consumer behavior data specifically for homeowners insurance, the predictive power is significantly enhanced, allowing companies to:
- Optimize premium pricing models by incorporating multidimensional insights gleaned from various consumer attributes.
- Predict claims through refined assessment metrics that explore historical credit behaviors in correlation with incident frequencies.
- Enhance customer segmentation by identifying profitable demographic groups using rich data composites.
- Mitigate risk by uncovering correlations between credit scores and specific loss propensities.
- Understand market shifts by analyzing data trends, thereby helping insurers navigate macroeconomic pressures and demand inconsistencies.
As the volume of data continues to expand, insurance companies are positioned to acquire increasingly accurate insights, enabling them to stay competitive and responsive to the ever-changing consumer market dynamics.
Conclusion
The modern insurance landscape is one marked by an ever-increasing reliance on data-driven insights. By employing comprehensive credit score data, organizations can predict losses with more accuracy, improve pricing models, and deliver enhanced customer satisfaction. The growth in data availability underscores its critical importance in helping insurers navigate future challenges more effectively. The evolution from rudimentary data collection methods to sophisticated analytics underlines the transformative impact of data in the insurance sector.
The shift towards more organized data collection and analysis represents a broader trend towards the adoption of data-driven strategies across different industries. Insurers must embrace this transition, utilizing data, such as credit scores, to drive innovation in their service offerings. This need places an emphasis on data discovery and analytics as key competitive differentiators.
Moreover, organizations that can adapt their approaches, making use of data monetization strategies to capitalize on their unique insights, stand to benefit significantly. For insurance professionals and domain experts, understanding these dynamics fosters improved decision-making and sharper strategic focus, ultimately guiding policy and operational adjustments.
Data proliferation presents a long-term opportunity beyond its immediate applications. Speculatively, corporations might find themselves capitalizing on alternative datasets, such as behavioral analytics merged with digital footprints, offering even richer insights into consumer behaviors and potential risks. These new data streams could further refine predictive models, allowing insurers to mitigate risks proactively.
The insurance industry’s forward trajectory will undeniably be propelled by its relationship with data. Embracing a data-driven culture fosters innovation and leadership, ensuring insurers remain relevant and responsive to the complexities of modern-day risk assessment. No sector exemplifies a greater need for transformational insight from data than the ever-evolving field of homeowners insurance.
Appendix
The potential for AI and data analytics to transform industries is immense, particularly within the domain of financial services. The wealth of data available promises significant advantages for various roles and sectors interested in harnessing its potential. For instance, consultants and market researchers can develop nuanced methodologies to predict and prevent high-loss scenarios using historical datasets combined with advanced modeling techniques.
Investors can use credit score data to inform their investment decisions, honing portfolio strategies that minimize exposure to high-risk profiles while maximizing returns from safer consumer bases. Similarly, insurance companies stand to benefit tremendously by operationalizing insights derived from rich datasets, enabling they to offer more competitive products and improve customer experience.
Data roles across all sectors are increasingly tasked with integrating cross-functional insights gained from various data types for strategic advantage. Market analysts and risk managers, leveraging AI and machine learning techniques, have the ability to decode complex datasets, transforming raw information into actionable insights that drive industry innovations.
The benefits extend further to industry regulators, who can use comprehensive data to enhance public policy frameworks, thus promoting responsible lending and maintaining financial system integrity. Insurers can capitalize on these shifts with enhanced predictive modeling capabilities informed by rich data insights.
Looking towards the future, data analytics is poised to enter a new age facilitated by smarter AI algorithms and robust machine learning frameworks. By discovering training data from decades-old records alongside modern filings, companies can unlock new insights into consumer behavior, fundamentally shifting the risk landscape.
Ultimately, the advent of data-enriched insights will necessitate a strategic focus on data acquisition, governance, and implementation. Organizations that embrace the changing tides towards data innovation will not only pave new paths toward improved customer service and enhanced operational efficiency, but they will also set benchmarks in an increasingly competitive insurance market landscape.