Unlocking Investment Potential with European Equity Consensus Data
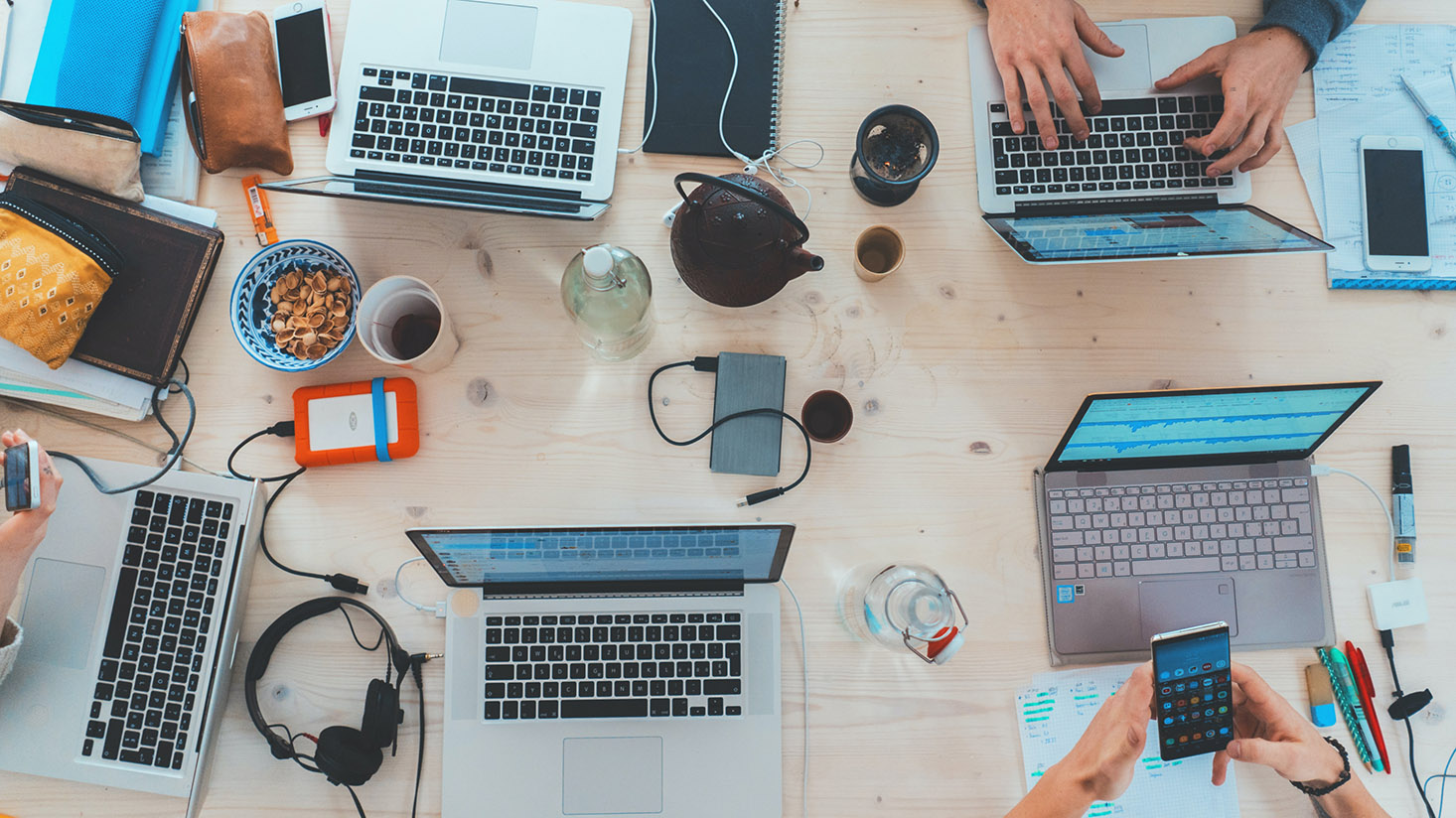
Introduction
In the fast-paced world of financial markets, having a clear and precise understanding of European equities has always been a complex endeavor. In the not-so-distant past, accessing reliable data and insights on European equities, especially on consensus data such as revenue or net sales, required painstakingly gathering information from diverse, scattered sources. Financial analysts and investors relied heavily on quarterly reports, company filings, and a myriad of data points gathered through manual processes. These methods, while groundbreaking for their time, left much to be desired in terms of speed and accuracy.
Before the age of technological advancement, the financial community was akin to navigating a ship through a dense fog, waiting weeks, or even months, for essential data to surface from annual reports. The delay often meant flawed forecasting and investment strategies that couldn't rapidly adjust to market dynamics. Investors were in the dark, waiting arduously for the next piece of the puzzle to emerge.
However, with the advent of technology—sensors, the Internet, and especially the proliferation of connected software—the landscape has been irrevocably transformed. These innovations have made it possible to amass vast amounts of financial data and transfer these data points seamlessly into databases for comprehensive analysis. Financial professionals now have the opportunity to engage with data in real time, obtaining insights as events unfold.
The profound importance of real-time data cannot be overstated. Having prompt and accurate data empowers businesses to make informed decisions swiftly, giving them the ability to anticipate emerging trends and market shifts without the cumbersome wait times of yesteryear. With [external data](https://www.nomad-data.com/connect) playing a pivotal role, organizations can make strategic decisions that propel them ahead of their competitors.
At the heart of financial analytics and forecasting is the European Equity Consensus Data, which paints a comprehensive picture of financial health and market projections. Acquiring insights into KPIs like revenue and EBITDA is now streamlined, reducing uncertainties and maximizing investment strategies.
This article delves into the multifaceted layers of European Equity Consensus Data, exploring the different types of data available, their historical applications, and how today's data professionals leverage these insights to make more informed decisions.
Financial Data
Historical Context and Evolution
Financial data has long been the backbone of investment strategies, driving decision-making across industries and markets. Historically, this data was sourced from analysts' reports and company disclosures, presenting a fragmented view of market trends. In the past, financial models were based on static reports, which were limited in scope and timeliness.
Roles such as financial analysts, portfolio managers, and corporate accountants were among the primary users of historical financial data. Before modern technological advances, these professionals relied on a mix of printed reports, telecommunication updates, and lengthy personal interpretations. Company visits and manual data entry were common practice to ensure the accuracy of their datasets.
Technology has significantly revolutionized this aspect of financial data. Digital transformation and database technologies have sped up data processing, ensuring rapid information dissemination. The advent of big data analytics, along with cloud storage solutions, has marked a turning point, allowing for vast datasets to be captured and stored efficiently.
Accelerating Data Availability and Usage
Today, the amount of financial data available is accelerating at an unprecedented rate, fueled by the interconnectedness of global markets. [Financial Data Providers](https://www.nomad-data.com/whats-new) now offer granular and timely-released coverage of global Analyst Consensus Data for European and international equities. As data providers compile information of broader scope and precision, investors seek to harness these insights to redefine their investment blueprints.
Examples of Financial Data Applications:
- Predictive Modeling: Leveraging Point-in-Time data for estimates, financial institutions build predictive models that anticipate market trends and inform strategic allocations.
- Comprehensive Performance Metrics: Access to datasets including earnings per share (EPS), revenue surprise forecasts, and macroeconomic indicators to paint a full canvas of company performance.
- Historical Analysis: By keeping track of historic analyst consensus data revisions, investors can evaluate past financial performances and subsequent projections.
- Global Equity Insights: Through consensus estimates aggregated from contributors, analysts analyze companies across 90+ countries, offering insights into diverse market dynamics.
- Corporate Strategy Evaluation: Using extensive datasets, businesses can align their strategies with financial expectations, assessing target prices and dividend potential.
Applications in Learning More About European Equities
When it comes to deepening understanding of European equities, financial data provides the necessary foundation. The applications are diverse, catering to a variety of industry needs. Here are several ways financial data is utilized:
- Equity Valuation Models: Built on comprehensive datasets, these models enable investors to make informed decisions based on meticulous financial analysis.
- Sector-Specific Insights: Different sectors have unique metrics, and insights may include industry-specific KPIs.
- AI and Machine Learning Integration: Modern financial models frequently incorporate [AI](https://www.nomad-data.com/blog/while-ai-has-stolen-the-show-its-always-about-the-data) and machine learning to enhance predictive capabilities and perform risk assessments.
- Real-Time Market Analysis: By accessing timely financial data, analysts can keep an ongoing pulse on market fluctuations and adapt strategies promptly.
- Investment Risk Management: Analysts use financial data to identify and mitigate investment risks, comparing variations in consensus and self-calculated estimates.
Conclusion
In summary, the importance of data in understanding European equities cannot be understated. Financial data, enhanced through modern technological advances, helps illuminate the once shadowy landscape of the financial markets. With access to diverse types of data, such as consensus estimates and predictive models, business professionals are equipped to make well-informed decisions that ensure resilience in changing market conditions.
The transformation into data-driven organizations is becoming increasingly critical. As organizations continue to utilize diverse types of data, the move towards [data discovery](https://www.nomad-data.com/whats-new) becomes inevitable. As firms analyze historical performance, they seek to also capitalize on valuable business prospects that lie within the financial sector.
In the quest for new revenue streams, corporations are contemplating the potential in [data monetization](https://www.nomad-data.com/data-sellers). Decades of historical data, once filed and tucked away, could emerge as crucial resources in understanding not only individual organizations but the broader scope of the market.
Looking ahead, one can speculate about innovative types of data yet to be harnessed. The realm of artificial intelligence offers numerous explorative pathways. With the emergence of AI-driven algorithms to seamlessly integrate disparate data sources, new opportunities for predictive analyses and seamless integration emerge.
Ultimately, financial data serves as the cornerstone for strategic planning across industries. By unraveling historic and [modern datasets](https://www.nomad-data.com/data-sellers), organizations are poised to achieve their business objectives with newfound precision.
As business landscapes continue to evolve, the ability to access and leverage data to devise strategic solutions will be at the heart of continued financial success. Organizations attuned to these advances are likely to maintain a competitive edge, grounded by the power of timeless financial insights.
Appendix
The role of financial data is far-reaching, impacting diverse industries and positions. Investors, consultants, insurance companies, market researchers, and financial analysts represent a small segment of [roles that benefit](https://www.nomad-data.com/whats-new) from data analytics.
The integration of financial data into industries resolves pressing issues, such as timely forecasting, strategic decision-making, and resource allocation. For instance, investors can utilize consensus data to navigate market fluctuations and prioritize high-yield sectors. Meanwhile, insurance companies assess financial trends crucial for developing competitive policies.
As technology advances, artificial intelligence plays a significant role in reshaping financial landscapes. [AI](https://www.nomad-data.com/blog/while-ai-has-stolen-the-show-its-always-about-the-data) unlocks data hidden in traditional formats, revealing insights from historical documents, alongside modern government filings and disclosures.
In the future, evolving financial technologies promise further illumination. Through comprehensive data analytics and predictive modeling, organizations are poised to craft adaptable strategies that align with market developments.
Ultimately, the continued insights offered by financial data position industries to thrive in dynamic environments, tapping into novel opportunities ripe for discovery.
With a keen emphasis on unlocking the value within datasets, organizations stand at the threshold of innovation, ready to chart new courses for sustained growth.