Unlocking Medical Insights Using Whole-Slide Digital Health Image Data
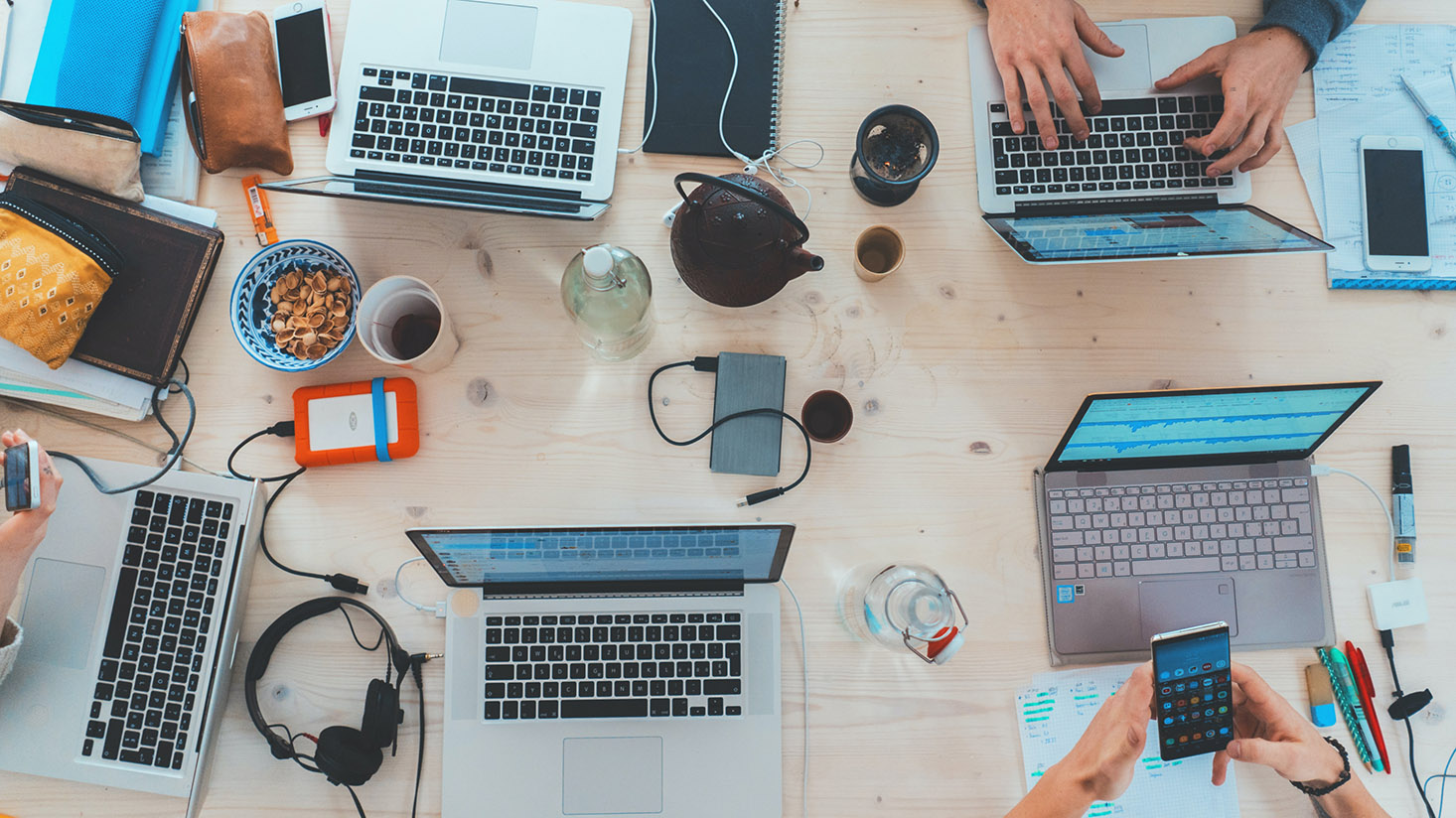
Unlocking Medical Insights Using Whole-Slide Digital Health Image Data
Introduction
In the rapidly advancing realm of medical research and healthcare, whole-slide digital health images represent a fascinating frontier, poised to transform the landscape of diagnostics and therapeutic interventions. Traditionally, the examination of tissue samples under a microscope has been a cornerstone in the diagnosis of numerous diseases. However, the physical and logistical limitations of these methods often impeded timely and effective diagnosis, leaving clinicians grappling with uncertainties.
For decades, pathologists relied on glass slides to examine biopsy samples, painstakingly interpreting what they observed through the lens of a microscope. This method, albeit revolutionary during its inception, was inherently limited by human proficiency and the need for physical storage and retrieval. Prior to the inception of data-driven methodologies, healthcare professionals were distant from the lucidity they needed, as conveying findings from one location to another entailed physically mailing these fragile slides, which took weeks, if not months.
However, the advent of digital technologies and the proliferation of sensors and connected devices have catapulted whole-slide imaging into the digital age. The digitization of health slides and advanced imaging technologies have opened new doors for real-time analysis, overcoming the hurdles of traditional microscopy, and significantly reducing the time lag between sample collection and diagnosis.
What once remained in the shadows for weeks or months is now brought to light instantly, with clinicians and researchers able to analyze vast amounts of data and make informed decisions with unprecedented speed and accuracy. This revolution, driven by rich datasets and high-resolution digital images, is transforming the way healthcare professionals approach diagnosis and treatment.
Understanding whole-slide digital health images is fraught with complexities, not least due to the immense size and intricate details of these datasets. Characteristics that might appear insignificant at a cursory glance can be pivotal when viewed under an appropriate lens. This is where the role of expansive data becomes crucial, bridging the chasm between raw imaging and actionable insights.
The significance of data in deciphering these high-detail images cannot be overstated. Today, the ability to analyze whole-slide images at a large scale brings a wave of opportunities in personalized medicine, allowing healthcare specialists to track disease progression with remarkable specificity. As industries begin to unravel the potential lying dormant in digital health images, the reliance on accessible, real-time data to drive timely and effective healthcare decisions becomes increasingly critical.
AI Training Data
Historically, the advancement of AI-driven healthcare solutions has hinged significantly on the availability of robust training datasets. AI training data plays a pivotal role in enhancing the algorithms that power diagnostic tools and predictive models. This type of data has long been the foundation upon which many groundbreaking technologies have been built, serving as the engine of innovation in industries reliant on machine learning and artificial intelligence.
AI training datasets are meticulously curated collections identified through medical collaborations, integrated across various platforms, and optimized for machine learning processes. These datasets are filled with de-identified pathology slides and annotations that enable AI models to learn and improve, repeatedly adapting their algorithms to recognize and process intricate patterns.
The medical field has embraced these datasets, funding monumental research projects and training robotic pathologists that can decode medical slides with remarkable accuracy. From AI-assisted surgery to automated radiology, practically every area in healthcare leans on this data, accelerating the pace and precision of clinical decisions.
As technology evolves, so too does the granularity and volume of AI training data. The digitization of medical slides and their corresponding metadata have spurred an unparalleled surge in data availability, empowering healthcare professionals with once-unimaginable access to insights.
How AI Training Data Enhances Understanding
- Pattern Recognition: AI training data fortifies algorithms designed to identify patterns indicative of disease or health abnormalities, forming the backbone of diagnostic accuracy.
- Data Annotation: Training datasets annotated with disease-specific markers allow AI systems to sift through volumes of slides efficiently, pinpointing areas of concern.
- Predictive Models: The use of such large datasets enables the development of models that not only diagnose but predict future health trends.
- Enhanced Accuracy: Utilizing AI for image analysis reduces errors due to human oversight, offering a second opinion that reaffirms initial findings.
- Scalable Solutions: The rapid processing of images facilitated by AI training data allows rapid scaling in operations where time-sensitive decisions are needed.
The exponential growth in AI training data signifies a shift in diagnostic methods, prompting a broader acceptance and further integration of technology into critical healthcare avenues. Discovering training data becomes an essential step in building profound health insights.
Healthcare Imaging Data
Healthcare imaging data offers an exceptional spectrum of opportunity in the comprehensive understanding of medical conditions, enhanced by the transition from physical to digital modalities. Initially dominated by X-rays and scans, imaging within medical science has expanded exponentially to include highly detailed digital renderings, exhibiting phenomenal progress toward unequivocal image fidelity.
This type of dataset encompasses a range of imaging modalities, including MRI, CT scans, and ultrasound, and serves sectors where visual clarity and spatial resolution are paramount, such as oncology, cardiology, and neurology. Moreover, whole-slide imaging falls into this expansive category, providing capabilities to digitize tissue slides for meticulous examination.
Healthcare imaging data's leap into the digital sphere is captured in its expansive use cases that span across diagnosing minute cellular anomalies to rendering comprehensive three-dimensional representations of complex bodily functions. Every pixel or voxel represents a potential insight, offering granular squeals of information previously inaccessible in the pre-digital era.
The transition to comprehensive datasets from rudimentary imagery propelled breakthroughs in personalized healthcare, with patients benefiting from custom treatments tailored through the imaging datasets' insights. This dynamic enables precise interventions by mapping images to recognized disease progressions and structures.
Effective Utilization of Healthcare Imaging Data
- Determining Disease Presence: Identification of pathological anomalies is significantly enhanced through high-definition imaging data visualization.
- Treatment Efficacy Tracking: Clinical outcomes can be quantified by comparing pre- and post-therapy imaging data to assess treatment efficacy.
- Longitudinal Studies: Combined imaging data allows for real-time monitoring of chronic diseases and their progression over time.
- Educational Use: Digitized images serve as valuable educational resources in training the next generation of healthcare professionals.
- Data Integration: Images can be integrated with clinical and genomic data for comprehensive disease profiling and decision-making.
As more pieces of healthcare imaging data come online, the potential to discover nuanced understandings through external data sources exponentially grows, pushing the boundaries of how diseases are visualized, analyzed, and understood.
Conclusion
In summary, the advent of whole-slide digital health images, bolstered by vast datasets, is an exhilarating frontier poised to magnify our medical insights and redefine diagnostics. These digital transformations have their roots in myriad datasets that drive AI, strengthening the decisions clinicians make every day. The use of AI training data has illustrated remarkable utility, underpinning both the complexity and potential of high-fidelity medical imagery.
Healthcare professionals win valuable time and precision by leveraging healthcare imaging data, tracking diseases with thoroughness and responding with unparalleled accuracy. As data availability continues to surge, the insights gleaned from these multifaceted data repositories will craft resonant stages for personalized healthcare, refracting through each spectrum of diagnosis and therapy.
The call to become data-driven is no longer mere speculation; it is a clarion beckoning industries to adopt smarter, faster, and scientifically steered approaches. Organizations must champion this movement toward comprehensive datasets, unlocking insights that had only been dreamt of in data’s absence.
The future of healthcare is toward data monetization, where institutions harness the potential of dormant datasets, creating shared ecosystems where insights flow seamlessly. The diversification of data types — enriched by evolving technologies — paints a vivid picture of the healthcare platforms of the future.
Speculating ahead, tomorrow's data offerings might well include real-time biological processes simulations and digital twin interactions. As datasets become increasingly diverse and novel forms are commercialized, tapping into these will yield answers to questions yet asked, rendering the healthcare field equitable, effective, and pioneering.
Appendix
The shift toward employing data in understanding whole-slide health digital images reverberates across numerous professional domains. Healthcare professionals, investors, consultants, and insurers find immense value in this data transformation, harnessing its insights to tackle industry challenges once deemed insurmountable.
Investors, for instance, can use these datasets to gauge the efficacy of new healthcare technologies and predict trends that steer beneficial investment decisions. By discerning the value captured in data-driven therapies and innovative products, they mitigate risks and fuel the financial backbone of futuristic medical advancements.
Consultants equipped with access to comprehensive data can craft bespoke strategies and empowers healthcare facilities towards operational efficiency, tailored patient care, and adherence to best practices. Through data, they wield the power to illuminate paths otherwise obscured by inefficient legacy processes.
Insurance companies leverage these insights to refine underwriting processes, ensuring precise evaluation of patient risks and the adequacy of coverage plans. Informed decision-making enhances customer satisfaction and enshrines equitable pricing structures for all.
The horizon is bright with potential as AI begins unearthing insights entrenched within decades-old documents and filings. Imagining how these advancements could coax rich insights from historical documents sheds light on transformative prospects for industries awaiting modernization.
Ultimately, these new challenges call for industries to harness the poignant echoes resonating from data – defining futures tasked with improving not just healthcare, but the fabric of societies interwoven by superior wellness standards.