Unlocking Urban Mobility Insights with Traffic Camera Footage Data
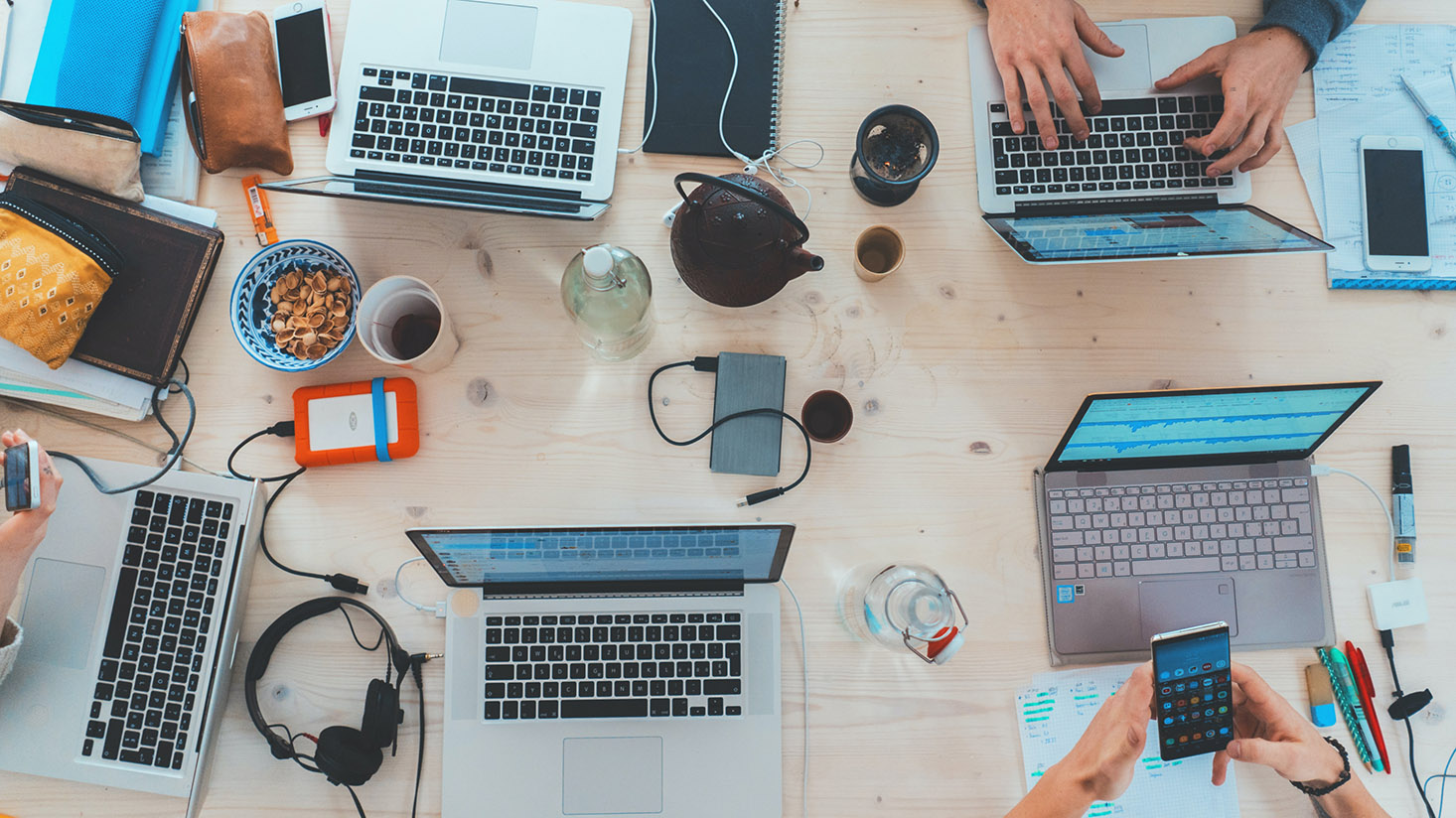
Introduction
The chaos and fluidity of urban road systems have always posed challenges to city planners, commuters, and traffic regulators alike. Historically, understanding and managing traffic flows, congestion, and the myriad interactions that occur at traffic intersections necessitated a cumbersome combination of human observation and rather rudimentary counting devices. In the days before the digital age revolutionized our access to data, city officials and traffic engineers would rely on manual traffic surveys, conducting studies to count vehicles in specific intersections or stretches of road by physically counting cars. These old methods were restricted by their subjective nature and limited by human capability.
However, with the advancement of technology and the rise of the Internet of Things (IoT), the ability to collect real-time data about urban traffic systems has been transformed exponentially. The advent of digital cameras, sensors embedded in vehicles, satellite navigation, and high-speed internet connectivity heralded a new era for traffic management. City planners now have the ability to access comprehensive datasets that include a spectrum of data points from traffic cameras capturing real-time data to detailed timestamped traffic incidents.
Collecting and analyzing traffic data has become indispensable in understanding urban mobility. These data help authorities make informed decisions regarding infrastructure improvements, congestion management, and urban planning efforts. They not only enhance day-to-day operations but also generate long-term strategic insights, effectively paving the way for smarter cities. Through these means, previously opaque trends can now be observed in near-real time, allowing for a more dynamic method of managing city traffic.
Before the availability of advanced data categories, response times to traffic changes lagged dramatically, with data flowing in weeks or months after the fact. In contrast, we now operate in an era where such changes can be acted upon instantaneously. This shift minimizes disruptions and significantly enhances commuter experiences.
Today, the draw of effective traffic management extends beyond governmental agencies to be critical for businesses, particularly those in logistics, ridesharing, and urban development sectors. An accurate understanding of traffic patterns is invaluable, enhancing profitability and service delivery by optimizing route choices, predicting heavy traffic, and avoiding delays.
AI Training Data
The explosion of AI technologies has revolutionized multiple aspects of our modern lives, traffic analysis inclusive. AI Training Data plays a pivotal role in the improvement of traffic-management systems by leveraging vast datasets of driving footage.
Historically, the deployment of AI in traffic management was hypothetical and confined due to a dearth of data and the primitive computing power available. However, with the emergence of advanced camera technologies, including 360-degree driving footage, AI systems can engage in deep learning to improve traffic performance and safety standards. These datasets are not just limited to cars but extend to bicycles and pedestrians within cityscapes, providing a holistic view of urban mobility.
This wealth of detailed footage is utilized extensively by city planners and AI developers to train models that understand, predict, and navigate complex traffic scenarios. Real-time and historical data from AI training datasets create a foundation upon which predictive algorithms are modeled, enhancing the predictive capabilities of traffic systems.
Applications of AI Training Data in Traffic Management:
- **Enhancing Traffic Flow**: AI-trained models can predict ebb and flow in traffic volumes, thereby optimizing signal timing and alleviating congestion at peak times.
- **Accident Prediction**: Identifying potential accident points based on past data and alerting drivers in advance, thereby enhancing safety.
- **Trajectory Analysis**: Analyzing how vehicles move and make decisions, aiding autonomous vehicle navigation systems.
- **Traffic Pattern Recognition**: Identifying unusual or emerging patterns that indicate system inefficiencies or accidents.
- **Road Condition Monitoring**: Using data to assess and predict the impact of weather or road conditions on traffic flow.
Collectively, the comprehensive use of AI training data in traffic management negates the necessity of manual data collection methods, ensuring efficiency, reduced error margins, and better resource allocation.
Automotive Data
Automotive Data plays a vital role in bridging the information gap between vehicles, roads, and regulators. This data is gathered primarily from onboard vehicle sensors, including dashcams and telematics equipment. Traditionally, regulatory agencies and automakers relied heavily on testimonials, accident reports, and physical examinations for data collection. These methods often resulted in partial overviews prone to subjective interpretations.
Recent advances in dashcam technologies and telematics have enabled the collection of extensive data regarding vehicle operations and conditions. Automotive data capture encompasses various aspects – from acceleration and braking patterns to real-time GPS and video footage that records travel anomalies and near misses.
This treasure trove of information is invaluable for understanding driving behavior, traffic anomalies, and efficiently managing roadways. Automotive data is extensively used in various industries, including insurance, to tailor usage-based policies and by fleet operators to enhance logistics and compliance.
Key Uses of Automotive Data in Urban Traffic Insight:
- **Driving Behavior Analysis**: Understanding driving patterns to influence safer driving initiatives and insurance premiums.
- **Incident Response**: Providing immediate context to incidents, thereby streamlining emergency response times.
- **Efficiency Tracking**: Monitoring travel times to improve fuel efficiency and route planning for delivery services.
- **Intersection Efficiency**: Data aids in informing infrastructure improvements and ensuring smoother turns and aggregations.
- **Predictive Maintenance**: Vehicles themselves report potential failures, ultimately reducing roadside delays.
Utilizing automotive data is a testament to the technological advancements that enable comprehensive traffic management and safer roads.
Traffic Camera Data
Traffic Camera Data is perhaps the most direct way of obtaining real-time insights into urban mobility dynamics. Historical approaches to traffic management involved sporadic deployment of traffic cameras for limited durations, primarily as a law enforcement tool. The internet revolution brought forth continuous streaming capabilities and enhancement in camera technologies, transforming traffic cameras into perpetual data generators.
Today's smart cameras do much more than record footage; they analyze it live. They offer data on traffic volume, speed, intersection usage, and even capture misconduct such as signal violations. This insight is crucial for managing urban spaces more intelligently. With an ever-increasing number of smart cities, the density of traffic cameras recording at every intersection has expanded dramatically.
Significant Applications of Traffic Camera Data:
- **Traffic Monitoring**: Continuous observation of flows to manage congestion and adjust operational parameters in real-time.
- **Accident Investigation**: Providing factual evidence for post-accident analysis to hasten claims processing and legal determinations.
- **Logistics Optimization**: Supporting industries in route planning to avoid bottlenecks, leading to timely deliveries.
- **Infrastructure Planning**: Indicating areas for maintenance or expansion based on consistent data-driven evidence.
- **Safety Improvements**: Highlight potential risk zones within the city for interventions like barriers or dedicated lanes.
The ability of traffic cameras to continuously stream and analyze data ensures a living, breathing network that radically transforms traffic oversight capabilities.
Conclusion
Urban traffic may seem chaotic, but with the insights provided by modern data categories, comprehending and managing this chaos becomes manageable and systematic. This article delves into the power of accessing external data sources to offer comprehensive insights into this field, emphasizing why relying on an assortment of high-quality, real-time data is crucial for the large-scale management of city traffic systems.
Business professionals across various sectors benefit from such datasets. By analyzing urban traffic trends, real estate developers, insurance agents, city planners, and logistics companies craft informed strategies that lead to better service offerings and operational efficiencies.
The democratization of data means that organizations now can broadly adopt a data-driven approach, unlocking potential benefits previously unseen. For both public and private entities, real-time traffic information can significantly impact decision-making processes, shaping better futures for cities and residents alike.
As modern-day corporations continue to focus on data monetization, traffic insights will remain a prominent field of interest. Future speculations envisage uniquely formatted datasets applying newer technologies, such as vehicle-to-infrastructure connectivity, to add depth and diversity to data horizons.
Another trend set to drive this progress is the growing integration of AI and smart systems within existing infrastructure frameworks. From smart traffic light algorithms to predictive traffic condition models, the marriage of data and AI propels traffic management into a new frontier of intelligence.
In conclusion, embarking on the path of data discovery and usage is critical in unlocking urban mobility insights. The evolution towards a data-centric decision-making model will continue to prove indispensable in reshaping and optimizing our urban lifestyles.
Appendix: Impact on Industries
The data revolution in traffic management significantly impacts numerous industries, with market players adapting to newfound information to solve age-old issues innovatively. The availability of multiple datasets ensures that decision-makers are better informed, and they can act swiftly and strategically.
In the real estate sector, understanding traffic patterns can enhance the valuation and appeal of properties, while improving infrastructure for optimized livability. Day-to-day commuters stand to benefit from real-time route optimizations and individualized travel itineraries, which have ultimately reduced commute times and improved urban navigation experiences.
The insurance industry extensively leverages data for predictive risk assessments and creating usage-based policies. By understanding driving behaviors and traffic history, insurance companies craft accurate premiums and predict claim probabilities.
The logistics and transportation sectors also find tremendous value in traffic data. By leveraging data-driven insights, delivery companies and rideshare operators can improve routes, lower delivery times, and enhance customer satisfaction.
The advancements in AI hold promise for unearthing hitherto unrevealed trends from older documents and records. Furthermore, AI can revolutionize traffic management, offering predictive insights into future traffic and roadway behaviors.
Future prospects are bright for data integration within these industries. As waves of innovation continue, data will remain center-stage, being both an enabler of success and a motivator for improvement. The ongoing efforts to locate and apply training data effectively represent the vanguard of the impending data revolution in urban mobility management.