Unveiling Building Types with Comprehensive Data in Canada
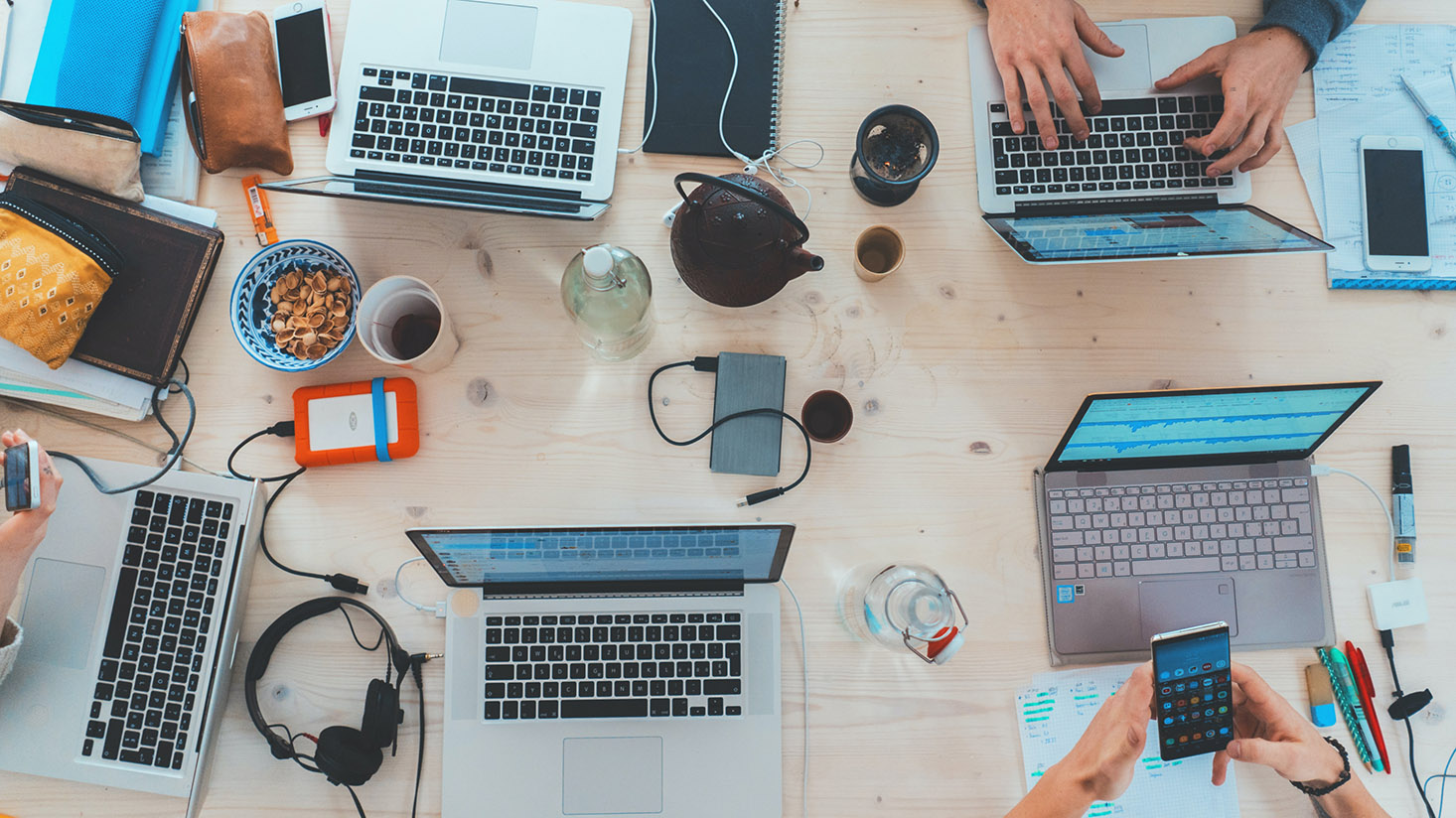
At Nomad Data we help you find the right dataset to address these types of needs and more. Submit your free data request describing your business use case and you'll be connected with data providers from our over
partners who can address your exact need.
Thank you! Your submission has been received!
Oops! Something went wrong while submitting the form.
At Nomad Data we help you find the right dataset to address these types of needs and more. Sign up today and describe your business use case and you'll be connected with data vendors from our nearly 3000 partners who can address your exact need.
Identifying the type of building at a given location is a task that, historically, presented significant challenges. Before the digital era, it was near impossible to accurately determine building types without engaging in time-consuming field surveys or relying on outdated paper records. For instance, before technological advancements, identifying whether an address was home to an apartment building, a shopping mall, or a school required manual visits and inspections or consultations with local government archives—a process fraught with inefficiencies.
Today, this challenge has drastically evolved with the advent of external data sources and technology. In the past, the lack of readily available data left business professionals and enthusiasts alike guessing, often waiting for weeks to months for updates. Now, data streams swiftly from numerous sources, eliminating the veil of uncertainty and enabling real-time insights.
The transformation began with the digitization of records and the emergence of interconnected devices and sensors. The geolocation revolution powered by satellites and the global positioning system (GPS) was a major landmark, lending a new dimension to how data about physical locations could be gathered and utilized. As smartphones, smart sensors, and the internet of things (IoT) penetrated daily life, the landscape shifted dramatically in favor of digital data aggregation.
Furthermore, Artificial Intelligence (AI) and machine learning technologies have exponentially increased the ability to analyze vast datasets. Leveraging AI, we can now piece together fragmented data to form a complete picture of a location's profile. This is crucial for businesses seeking to make data-driven decisions about properties and real estate.
Being informed about building types is not just a matter of academic interest or simple curiosity—it holds practical implications for various sectors. For example, businesses establishing new branches, real estate investors, and urban developers use these insights to strategize effectively. By understanding what type of establishments are within certain locales, they can better anticipate market needs and consumer behavior.
For example, these datasets include precise coordinates and descriptions that map out every conceivable point of interest (POI) within Canada. Such data is invaluable for understanding categories of commercial locations, which could range from retail strips and restaurants to hospitals and educational buildings. Businesses across various industries harness this data to enhance operational strategies and improve customer engagement.
Geolocation data’s evolution is marked by the increasingly granular level of detail it now offers. Today, providers like those tracking hundreds of thousands of POIs in Canada, are able to match any address against a database that includes detailed polygons and property footprints for conclusive building categorization. This **geolocation data** allows for:
- **Accurate Mapping**: Identifying precise locations of buildings and their surroundings. - **Property Footprinting**: Understanding the exact square footage and layout of properties. - **Traffic Patterns**: Monitoring foot traffic to assess building function and use. - **Categorizations**: Assigning building types at a granular level (e.g., schools, offices, malls). - **Market Analysis**: Supporting strategic positioning for businesses venturing into new locales.
The surge in data collection and technological improvements means that actionable insights based on location data are accelerating, empowering industries to make more data-driven decisions.
Web scraping has made it feasible to compile information such as building permits, commercial directories, corporate registrations, and real estate listings. Such data can be joined with other datasets to create a robust database of building types in Canada. This amalgamation of data supports:
- **Up-to-Date Records**: Maintaining current information on property types and statuses. - **Data Correlation**: Linking structural information with other data sources for comprehensive insights. - **Trend Analysis**: Identifying trends in property utilization and urban development. - **Market Intelligence**: Gathering competitive intelligence about emerging commercial zones.
**Structural building information** captured via web scraping can paint a vivid picture of a property's history and current status by aggregating:
- **Property Listings**: Information on available commercial and residential property prospects. - **Zoning and Permits**: Data related to zoning laws and permits impacting building functionalities. - **Infrastructure Details**: Comprehensive details about building materials and layouts.
Data providers like Soleadify facilitate the collection of such comprehensive data, offering a critical advantage to businesses aiming to identify and categorize building types efficiently in a digital-first world.
With the contemporary digital infrastructure, real estate data provides invaluable insights into the commercial property landscape. This data serves realtors, investors, and developers, among other stakeholders, in navigating the vast and intricate real estate markets. Real estate datasets track crucial metrics related to every commercial property, including building classifications, ownership, sale, and lease details.
The advent of technology has empowered real estate data providers to cultivate vast arrays of information that are readily accessible and updateable. This ability ensures that stakeholders are operating with the most current data available, which is essential for informed decision-making:
- **Ownership Records**: Identifying the ownership history and current owner of commercial properties. - **Transactional Data**: Compiling data related to sale and lease transactions. - **Building Attributes**: Documenting vital information such as age, size, and amenities of properties.
### Leveraging Real Estate Data
Utilizing this robust data warehouses strengthens stakeholders' understanding by providing:
- **Predictive Analysis**: Utilizing historical data to forecast future real estate trends and building requirements. - **Competitive Strategy**: Gaining actionable insights into competitor properties and market saturation.
The acceleration of this data type is undeniable, as the real estate business landscape continues to evolve rapidly, shaped by data-driven decisions.
The value gained from accessing such **categories of data** cannot be overstated. The complex web of geolocation patterns, property footprints, and market data reveals the true nature of our environments, driving better decisions. This need for a more data-centric approach is why organizations must continue to place emphasis on becoming more data-driven in their pursuits.
With the emergent trend of data monetization, companies realize the untapped potential within their archives, leading to a new monetization channel where valuable [[data](https://www.nomad-data.com/data-sellers)] can be commercialized. Consequently, building exploration is ripe for future innovations in data-based insights, pointing to even more detailed categorizations, the impact of evolving urban layouts, and the influence of societal trends.
Future advancements will undoubtedly include new layers of data, perhaps capturing building sustainability features, real-time structural conditions, or even symbiotic relationships between property placements and environment patterns. This continual evolution of data types promises to offer additional insights into buildings as we have not previously imagined.
In light of these advancements, it's crucial to stay engaged with what's possible—businesses and individuals must remain vigilant, exploring new ways to augment and apply data for groundbreaking insights and competitive advantages.
For real estate investors, having a detailed topography of building types within their investment region is invaluable. This real-time data enables them to strategically position investments, predicting market demands and potential returns based on the typology and geographic distribution of properties. Data-driven decision-making helps them identify untapped markets and optimize their investment portfolio.
Financial consultants and analysts leverage building data to provide strategic recommendations to clients. By understanding the types of properties within specific areas, they can better predict market trends and advise on potential acquisitions and divestments. With the aid of AI, they can uncover value in decades-old property records and synthesize intricate government filings, pushing analytical boundaries.
Urban planners and developers, integral to the growth and functionality of cities, use this data-driven lens to influence infrastructure development, zoning amendments, and community planning. This enables them to enhance urban productivity and growth in line with evolving demographic and industry needs. AI and data forecasting pave the way for sustainable urban plans that meet future demographic changes efficiently.
Additionally, market researchers find building type data ideal for conducting comprehensive analyses. They can segment commercial activity zones, examine shifts in industry distributions, and evaluate new opportunities for market entry.
This viewpoint is not confined to data access alone. Understanding building types through data insights is pivotal, bringing together multiple industry perspectives for a cohesive, smarter tomorrow.
Today, this challenge has drastically evolved with the advent of external data sources and technology. In the past, the lack of readily available data left business professionals and enthusiasts alike guessing, often waiting for weeks to months for updates. Now, data streams swiftly from numerous sources, eliminating the veil of uncertainty and enabling real-time insights.
The transformation began with the digitization of records and the emergence of interconnected devices and sensors. The geolocation revolution powered by satellites and the global positioning system (GPS) was a major landmark, lending a new dimension to how data about physical locations could be gathered and utilized. As smartphones, smart sensors, and the internet of things (IoT) penetrated daily life, the landscape shifted dramatically in favor of digital data aggregation.
Furthermore, Artificial Intelligence (AI) and machine learning technologies have exponentially increased the ability to analyze vast datasets. Leveraging AI, we can now piece together fragmented data to form a complete picture of a location's profile. This is crucial for businesses seeking to make data-driven decisions about properties and real estate.
Being informed about building types is not just a matter of academic interest or simple curiosity—it holds practical implications for various sectors. For example, businesses establishing new branches, real estate investors, and urban developers use these insights to strategize effectively. By understanding what type of establishments are within certain locales, they can better anticipate market needs and consumer behavior.
Geolocation Data
Geolocation data has been at the forefront of the building identification evolution. Initially confined to military and scientific research, geolocation technologies have broadened their application to include commercial uses such as building categorization. Geolocation data providers gather extensive amounts of information from satellites and GPS systems, which they then compile into comprehensive datasets.For example, these datasets include precise coordinates and descriptions that map out every conceivable point of interest (POI) within Canada. Such data is invaluable for understanding categories of commercial locations, which could range from retail strips and restaurants to hospitals and educational buildings. Businesses across various industries harness this data to enhance operational strategies and improve customer engagement.
Geolocation data’s evolution is marked by the increasingly granular level of detail it now offers. Today, providers like those tracking hundreds of thousands of POIs in Canada, are able to match any address against a database that includes detailed polygons and property footprints for conclusive building categorization. This **geolocation data** allows for:
- **Accurate Mapping**: Identifying precise locations of buildings and their surroundings. - **Property Footprinting**: Understanding the exact square footage and layout of properties. - **Traffic Patterns**: Monitoring foot traffic to assess building function and use. - **Categorizations**: Assigning building types at a granular level (e.g., schools, offices, malls). - **Market Analysis**: Supporting strategic positioning for businesses venturing into new locales.
The surge in data collection and technological improvements means that actionable insights based on location data are accelerating, empowering industries to make more data-driven decisions.
Web Scraping Data
Another pivotal aspect in identifying building types is **web scraping data**. The practice of web scraping involves extracting large amounts of data from websites in an automated manner. This method has proven essential in collecting real-time data about buildings from publicly accessible online databases and websites. Websites often contain valuable information about property attributes, zoning classifications, and occupancy data.Web scraping has made it feasible to compile information such as building permits, commercial directories, corporate registrations, and real estate listings. Such data can be joined with other datasets to create a robust database of building types in Canada. This amalgamation of data supports:
- **Up-to-Date Records**: Maintaining current information on property types and statuses. - **Data Correlation**: Linking structural information with other data sources for comprehensive insights. - **Trend Analysis**: Identifying trends in property utilization and urban development. - **Market Intelligence**: Gathering competitive intelligence about emerging commercial zones.
**Structural building information** captured via web scraping can paint a vivid picture of a property's history and current status by aggregating:
- **Property Listings**: Information on available commercial and residential property prospects. - **Zoning and Permits**: Data related to zoning laws and permits impacting building functionalities. - **Infrastructure Details**: Comprehensive details about building materials and layouts.
Data providers like Soleadify facilitate the collection of such comprehensive data, offering a critical advantage to businesses aiming to identify and categorize building types efficiently in a digital-first world.
Real Estate Data
Real estate data is undeniably a cornerstone for identifying building types in Canada. Historically, this data was primarily aggregated through public records and real estate boards, which posed accessibility challenges. The modernization of real estate data collection has paved the way for a more streamlined and comprehensive analysis.With the contemporary digital infrastructure, real estate data provides invaluable insights into the commercial property landscape. This data serves realtors, investors, and developers, among other stakeholders, in navigating the vast and intricate real estate markets. Real estate datasets track crucial metrics related to every commercial property, including building classifications, ownership, sale, and lease details.
The advent of technology has empowered real estate data providers to cultivate vast arrays of information that are readily accessible and updateable. This ability ensures that stakeholders are operating with the most current data available, which is essential for informed decision-making:
- **Ownership Records**: Identifying the ownership history and current owner of commercial properties. - **Transactional Data**: Compiling data related to sale and lease transactions. - **Building Attributes**: Documenting vital information such as age, size, and amenities of properties.
### Leveraging Real Estate Data
Utilizing this robust data warehouses strengthens stakeholders' understanding by providing:
- **Predictive Analysis**: Utilizing historical data to forecast future real estate trends and building requirements. - **Competitive Strategy**: Gaining actionable insights into competitor properties and market saturation.
The acceleration of this data type is undeniable, as the real estate business landscape continues to evolve rapidly, shaped by data-driven decisions.
Conclusion
The journey from antiquated methods to sophisticated data-driven strategies in determining building types represents a significant leap in productive capability for various sectors. By embracing data, we gain insights once unthinkable, transforming the landscape of property and urban development into an era defined by informed precision and strategic foresight.The value gained from accessing such **categories of data** cannot be overstated. The complex web of geolocation patterns, property footprints, and market data reveals the true nature of our environments, driving better decisions. This need for a more data-centric approach is why organizations must continue to place emphasis on becoming more data-driven in their pursuits.
With the emergent trend of data monetization, companies realize the untapped potential within their archives, leading to a new monetization channel where valuable [[data](https://www.nomad-data.com/data-sellers)] can be commercialized. Consequently, building exploration is ripe for future innovations in data-based insights, pointing to even more detailed categorizations, the impact of evolving urban layouts, and the influence of societal trends.
Future advancements will undoubtedly include new layers of data, perhaps capturing building sustainability features, real-time structural conditions, or even symbiotic relationships between property placements and environment patterns. This continual evolution of data types promises to offer additional insights into buildings as we have not previously imagined.
In light of these advancements, it's crucial to stay engaged with what's possible—businesses and individuals must remain vigilant, exploring new ways to augment and apply data for groundbreaking insights and competitive advantages.
Appendix: Industry Benefits from Building Data
Various roles and industries stand to gain significantly from data related to building identification in Canada. These stakeholders have unique challenges that data has the power to profoundly address.For real estate investors, having a detailed topography of building types within their investment region is invaluable. This real-time data enables them to strategically position investments, predicting market demands and potential returns based on the typology and geographic distribution of properties. Data-driven decision-making helps them identify untapped markets and optimize their investment portfolio.
Financial consultants and analysts leverage building data to provide strategic recommendations to clients. By understanding the types of properties within specific areas, they can better predict market trends and advise on potential acquisitions and divestments. With the aid of AI, they can uncover value in decades-old property records and synthesize intricate government filings, pushing analytical boundaries.
Urban planners and developers, integral to the growth and functionality of cities, use this data-driven lens to influence infrastructure development, zoning amendments, and community planning. This enables them to enhance urban productivity and growth in line with evolving demographic and industry needs. AI and data forecasting pave the way for sustainable urban plans that meet future demographic changes efficiently.
Additionally, market researchers find building type data ideal for conducting comprehensive analyses. They can segment commercial activity zones, examine shifts in industry distributions, and evaluate new opportunities for market entry.
This viewpoint is not confined to data access alone. Understanding building types through data insights is pivotal, bringing together multiple industry perspectives for a cohesive, smarter tomorrow.