Using Financial and Economic Data to Understand Monthly GDP Trends in Germany
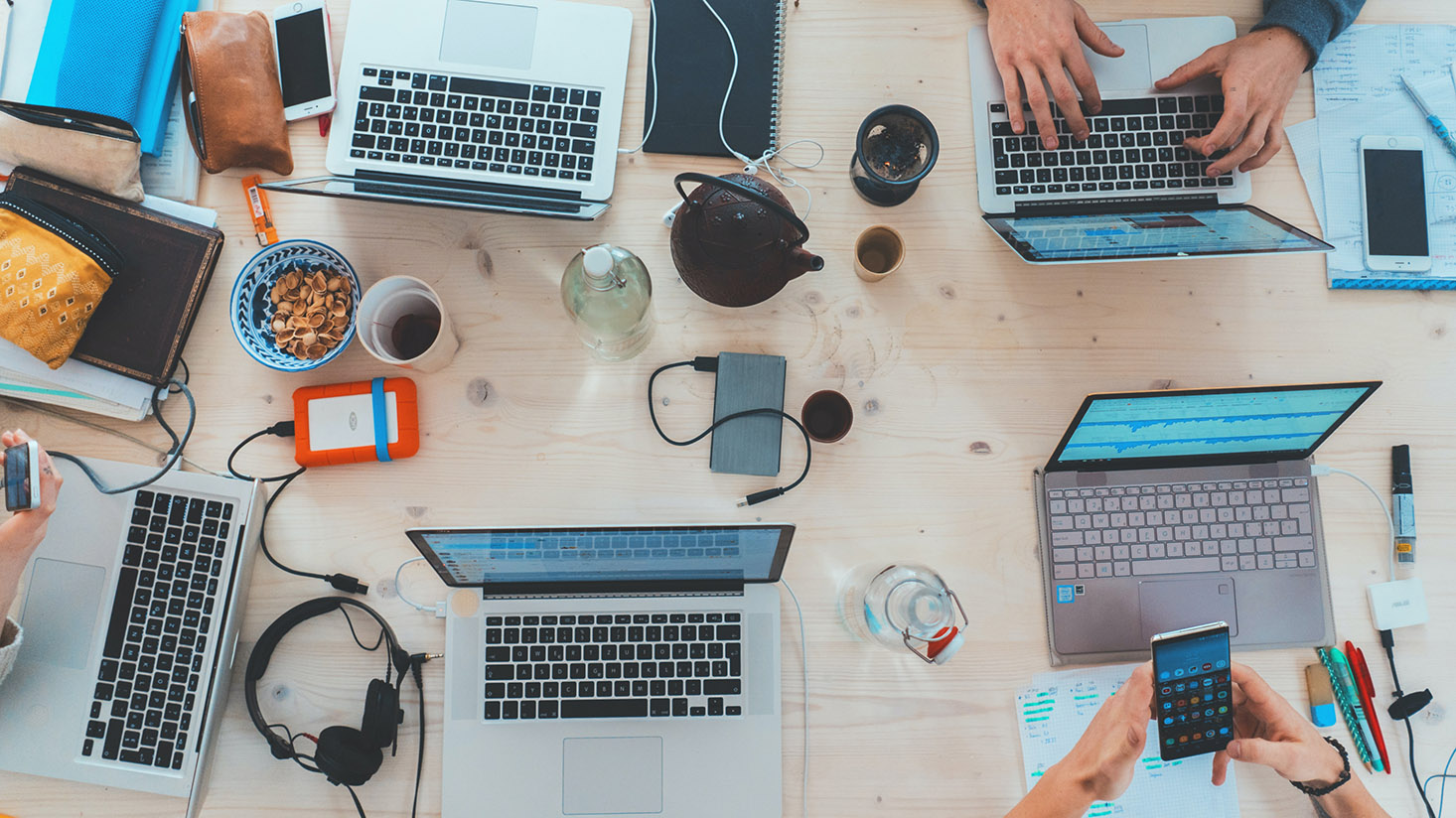
Introduction
The landscape of understanding economic health, particularly tracking GDP trends in countries like Germany, has transformed dramatically over the years. Historically, analyzing GDP data was rife with challenges, as it relied heavily on outdated methodologies and infrequent data release schedules. Academics and policymakers would pour over quarterly reports, pencil in hand, trying to make sense of stagnant numbers and delayed trends. Before the advent of comprehensive digital data, economic analysts depended on a patchwork of surveys, journalistic interviews, and scarce governmental updates to piece together a holistic view of economic performance.
In the earlier days of economic analysis, methods were painstakingly manual. Researchers had to sift through actual ledgers and printed financial accounts which were often incomplete or outdated. Data scarcity was a mainstay, and analysts were left in the dark, working with estimates and projections until official reports were available. The information gap meant that critical economic decisions could only be made retroactively, leading to remedies that arrived too late to mitigate emerging issues. The data behind GDP and economic analysis were sparse, leaving many in blind reliance on intuition rather than data-driven insights.
The advent of the internet, sensor technologies, and connected digital devices has been nothing short of revolutionary for economic data collection and analysis. Digital advancements have ushered in an era where data points are continuously captured and updated in real-time. The proliferation of software systems integrated into every facet of the economic chain has allowed the capture of continuous streams of data, turning every financial transaction, employer payroll adjustment, or trade invoice into a part of a larger picture. No longer are analysts hamstrung by lagging reports and scarce census data; real-time analysis of monthly GDP trends in Germany is achievable, and insights can be generated instantly.
Today’s data-driven world means that companies can make informed decisions faster, with unprecedented accuracy. By tapping into comprehensive datasets from external data sources, businesses can dynamically track economic indicators such as GDP, consumer spending, and industrial output. Continuous data flows mean that variances in economic performance can be identified and accounted for without the historical lag times. Such real-time insight is a substantial leap forward, catalyzing dynamic decision-making and strategic planning.
Moreover, access to burgeoning datasets has democratized economic understanding. A wider array of industries can now utilize sophisticated data categories to forecast future trends, analyze current conditions, and optimize their operations in response to on-the-ground realities. Access to this data empowers end-users, allowing both large corporations and smaller enterprises to function with similar competitive advantages in a rapidly globalizing economic landscape.
In summary, data has shifted from a nice-to-have to an essential component for understanding economic trends such as Germany's monthly GDP. As technology advances, the granularity and immediacy of economic insights only grow, paving the way for more accurate forecasting and enhanced economic strategizing.
Financial Data
Historical Context and Evolution
Financial data has been a cornerstone of economic analysis throughout history. From ledgers scrawled by hand centuries ago to today’s dynamic digital dashboards, financial data reflects the heartbeat of economic activity. Historical reliance on quarterly and annual financial statements illustrated a period where data was primarily retrospective. It was not until the digital revolution that access to real-time financial data became widely feasible.
Examples of financial data include but are not limited to GDP figures, inflation rates, currency exchange rates, and various forms of employment statistics. Industries such as banking, investment firms, and governmental agencies have long relied on financial data to shape policy and drive investment strategies. As technology has advanced, so too has the capability of financial data providers to offer more detailed, frequent insights.
Technological advances such as cloud computing, solid database architecture, and robust analytics tools have contributed immensely to the growth of financial data. Increased data storage and faster processing capabilities allow for more refined data analysis across multiple languages and currencies, making financial data more accessible than ever before.
Accelerating Data Availability
The velocity of data generation in the financial sector has accelerated continuously over the last decade. Real-time data streams, mostly available due to advancements in software and networking capabilities, have rendered previous reporting schedules obsolete, giving rise to proactive instead of reactive strategies. This immediacy allows analysts to gauge monthly GDP with unprecedented precision.
Specific Applications
Financial data is particularly potent in understanding monthly GDP trends in Germany. Here are several ways in which financial data can be instrumental:
- Real-time Analysis: Use data streams to interpret daily changes, providing a clearer picture of how market fluctuations impact GDP in real-time.
- Forecasting: Historical and real-time data facilitate predictor models that can forecast economic trends with greater accuracy.
- Trend Identification: Recognize patterns that might indicate cyclical trends or the emergence of underlying issues affecting GDP.
- Policy Impact Evaluation: Analyze how specific monetary or fiscal policies affect economic growth metrics over a calendar month.
- Investment Strategy Development: Improve tactics for financial and corporate investments by understanding how GDP forecasts impact market conditions.
Economic Data
Historical Context and Usage
Economic data, distinct yet intrinsically linked to financial metrics, creates nuanced portraits of a nation’s overall fiscal health. In early economic history, much like financial data, economic indicators were sparse and less frequently published. Key metrics such as productivity rates or sector-specific growth patterns were compiled manually, frequently by exhaustive survey or census data.
This category encompasses data points like employment rates, retail sales, industrial production measures, and consumer price indexes. Economists and policymakers have depended on such data for crafting economic policies and advising on growth strategies, particularly within governmental and non-profit sectors focused on welfare and community growth.
Modern Data Collection Methods
Modern collection methods for economic data involve extensive use of digital systems and smart technology. These tools harvest data utilizing both governmental databases and private sector collaborations, making the collection methodologies incredibly broad in scope and depth.
Applications in Monthly GDP Analysis
Economic data provides a vital lens through which to view GDP dynamics. Here are detailed applications:
- Macro-Micro Economic Analysis: Break down data into macroeconomic trends—such as unemployment rates—and microeconomic trends within specific industries to understand broader GDP impacts.
- Sector Health Tracking: Continuously monitor growth sectors such as technology or healthcare and their contributions to the national GDP.
- Policy Formation Insights: Utilize economic insights to shape or adjust national economic policies aimed at sustainable growth.
- Predictive Modelling: Use real-time and historical data to forecast monthly GDP fluctuations prior to official report releases.
- Market Sentiment Analysis: Understand public sentiment through consumer spending trends which serve as precursor indicators to GDP changes.
Conclusion
The transformative power of diverse datasets is reshaping how we understand economic indicators like Germany's monthly GDP. The continual evolution of data collection advances allows business professionals to make data-backed decisions, optimizing responses to market shifts and policy changes. The reliance on comprehensive external data sets the stage for far-reaching analyses capable of shaping the economic landscape.
Organizations must evolve to become more AI-driven and data-centric if they wish to sustain competitive advantages. The strategic use of data parcels not only provides insights but can also yield expansive market opportunities for emerging firms. The future is data-driven, and leveraging data is imperative to stay relevant in today’s cutthroat market.
Furthermore, the trend towards monetizing valuable data sets has gained traction across numerous industries, with many businesses looking to monetize their data through insights sales. Economic impacts reflect horizontally across sectors; thus, data vendors will likely expand offerings to include terrain-specific insights, catering to diversified business needs.
In the future, innovative data offerings will encompass not only today’s sought-after metrics but trend toward newly created data types as markets continue to evolve. Sophisticated data solutions will cater to granular queries, enhancing organizational agility in day-to-day operations.
Embracing the full spectrum of data resources enables industries to remain proactive, innovative, and increasingly constructive in dynamically volatile market environments. Additionally, as technology advances, the sophisticated tapestry of economic indicators will grow richer, providing even deeper insights into monthly GDP analysis.
In closing, engaging with the flow of economic metrics brings clarity and actionable insight to businesses aspiring to lead in financial strategy and economic understanding. The road ahead promises innovations in data gathering and ¬¬ analysis propelling transformational shifts in economic intelligence.
Appendix: Industry Roles and Future Perspectives
Data has progressively shaped various industries, offering tailored insights tailored to their unique needs, particularly when considering the multifaceted nature of GDP analysis. Prominent roles such as investors, consultants, and market researchers have seen data redefine their operational strategies.
Investors have utilized forecasts based on economic and financial data to mitigate risks and elevate decision-making processes. Being able to predict economic downturns or identify growth pockets allows them to refine investments, thus securing profitability in diversified market climates.
Consultants, on the other hand, draw from a pool of economic metrics to advise on strategic business ventures. Whether coaching businesses on mergers or suggesting market entry strategies, real-time datasets provide essential guidance based on empirical evidence rather than assumptions.
Insurance companies have leveraged this data to shift underwriting practices, pricing policies according to expected economic performance, thus ensuring premium structures reflect potential risk accurately. This practice provides a safety net against unknown economic variables.
Market researchers depend heavily on data to craft marketing plans and corporate messaging that resonates with prevailing economic conditions. They identify consumer trends, guiding product development, and promotional angles tailored to the pulse of the market.
As AI progresses, future roles will uncover latent potential within decades-old data, reinvigorating its utility. Organizations may leverage AI-driven insights to extract actionable intelligence from historical datasets, fostering a revitalized perspective on organizational roadmap development.