Utilizing Diverse Data Streams for Comprehensive Wildfire Causation Insights
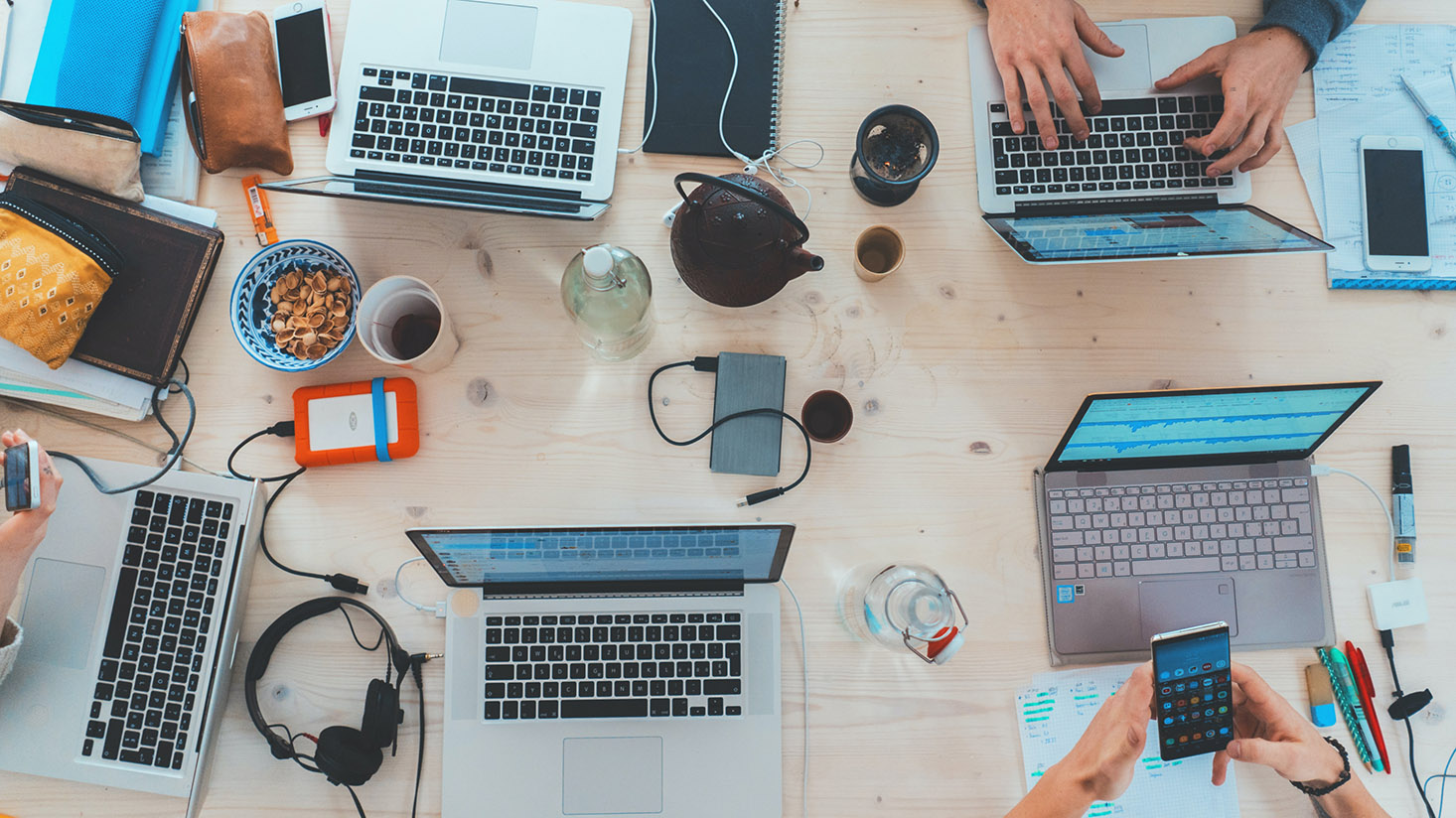
Introduction
The devastation wrought by wildfires is a pressing concern across North America. Historically, understanding the causation and potential risks associated with these destructive forces has been fraught with challenges. Before the advent of modern data collection methods, communities relied heavily on rudimentary tracking methods—smoke signals, news reports, and word-of-mouth were primary means of assessing wildfire spread. Firewardens, local safety officials, and even ranchers served as the earliest 'data points,' manually keeping tabs on fire breaks and assessing damages post-disaster.
In those days, the ability to forecast or mitigate fire risk was hindered by limited data. With dynamic ecosystems and a tapestry of contributing factors, insights were few and painfully accumulated over weeks or even months of observation. The long latency in obtaining actionable information left decision-makers perpetually on the back foot, often scrambling to organize preventive measures or respond to active threats.
Fast forward to today, and the landscape of understanding wildfire causation has transformed remarkably. Enabled by a proliferation of AI, sensors, satellite imagery, and the internet, the availability and granularity of data have grown exponentially. Now, various types of data focus on understanding the whims of nature, not just reactively but proactively.
Data related to weather patterns, vegetation, human habitation, and topography are now interconnected in databases accessible in real time. Decision-makers, through various data dashboards and analytics platforms, can now perceive the imminent risks posed by wildfire patterns almost instantaneously. This data-driven approach provides crucial insights, helping local authorities and business professionals alike in crafting more effective risk management strategies.
As technology continues to evolve, the speed and accuracy with which we can acquire data will only improve. Advanced predictive analytics powered by extensive datasets not only shed light on past occurrences but also enhance the capability to predict future threats. As a result, organizations are becoming better equipped to make timely, database decisions in safeguarding property and human lives from the caprices of nature.
The journey towards a comprehensive understanding of wildfire causation is far from complete. Yet, the technological strides made over recent years have set a strong foundation. This comprehensive article delves into the types and sources of data that hold the key to unlocking further insights into wildfire causation in North America.
Climate Data
Historically, climate data has been fundamental in understanding wildfire risk. Early in the field, researchers depended on regional climate reports—often drawn from localized weather stations—to surmise wildfire trends. These initial efforts paved the way for the sophisticated climate models we possess today.
Modern climate data goes beyond simplistic metrics like temperature and precipitation. It encompasses multifaceted data streams, including flame height, burn probability, and extreme fire weather days. Each of these parameters paints a nuanced picture of wildfire risk and can be dissected in five-year increments well into the mid-21st century.
The utility of climate data extends across numerous roles and industries. Forestry departments, urban planners, and insurance firms historically rely on such data to strategize and assess potential wildfire impact and devise mitigation strategies. Technological advances—ranging from enhanced computing power to more precise sensors—have enabled the availability of richer and more granular climate datasets.
Due to these advancements, the quantity of available climate data continues to accelerate. Increased granularity enables stakeholders to draw insights at a property level, ensuring risk assessments are tailored to specific geographies.
The possibilities for leveraging climate data to gain insights into wildfire causation are broad. Here are a few:
- Predictive risk analysis: Utilize historical climate data to anticipate areas more susceptible to wildfires.
- Resource optimization: Allocate firefighting resources efficiently based on risk metrics.
- Development planning: Guide urban development away from high-risk zones.
- Insurance policy formulation: Design insurance products based on location-specific historical and predicted climate risks.
- Sustainable land management: Develop forestry practices minimizing the intensity of potential fires.
Real Estate Data
Real estate data plays an unexpectedly crucial role in wildfire risk modeling. Though often linked primarily with property transactions and valuations, real estate data provides a wealth of insights related to wildfire causation and risk.
Traditionally, professionals in real estate and property management relied primarily on transaction details and static risk codes. In the modern world, frequent updates and API-driven interfaces allow users to obtain dynamic risk scores—key for any property in the United States in relation to wildfire likelihood.
This formalizes how property is evaluated relative to fire risk, enhancing the ability of real estate professionals, homeowners, and urban developers to make informed decisions. APIs deliver rapid access to the latest datasets, providing instant insights, facilitating automation, and allowing expansive geospatial analysis.
The future of real estate data is increasingly intertwined with metrics on wildfire causation. Professionals can utilize this data to craft a proactive approach to property development and risk mitigation. Some examples include:
- Location-specific risk assessment: Customize architectural safety based on property-level wildfire data.
- Property insurance planning: Use real estate data to offer differentiated insurance based on risk levels.
- Investive advisement: Inform potential buyers about property fire risks during purchase considerations.
- Urban zoning: Configure zoning regulations to reduce fire spread potential in vulnerable areas.
- Infrastructure reinforcement: Advise on infrastructure enhancements in wildfire-prone zones.
Weather Data
Delving into weather data reveals it as a contemporary cornerstone for deciphering wildfire causation. This extensive dataset includes classic measures like temperature, humidity, and wind patterns, which are crucial for predicting wildfire susceptibility.
Early meteorologists primarily focused on predicting daily weather, while wildfire risk remained a less accessible aspect of those predictions. However, today's interconnected and alive datasets have broadened what we mean by weather-related wildfire data. Emerging technologies develop geo-spatial databases housing years of wildfire-centered climatology data.
The versatility inherent in weather data makes it indispensable for diverse industries. Environmental agencies, agricultural sectors, and disaster response teams all rely on this intricate data to better prepare for and respond to wildfire threats.
As technology advances, the scope and detail of weather data continue to grow, offering adaptability to a myriad of designations. Here are potential uses specific to wildfire insight:
- Historical trend analysis: Identify regions with cyclically high wildfire risk.
- Climatic pattern correlations: Spot correlations between climatic parameters and historical wildfire events.
- Fire modeling simulations: Use datasets to build virtual models of potential fire spread following given analysis.
- Drought and precipitation monitoring: Align wildfire predictions with areas undergoing extended dry spells.
- Systemic precautions: Provide data-based alerts that pre-emptively limit activities known to cause fires in certain conditions.
Satellite Data
No discussion on wildfire causation data would be complete without touching on satellite data. This data type heralds from a history of space exploration where early satellites aimed for large-scale terrestrial surveillance, generally tracking landmass and atmospheric shifts.
Today, satellites adeptly monitor terrestrial activities at previously impossible resolutions, providing real-time feedback at a macro level while zoning in on hotspots such as evolving wildfires.
Satellite data’s potential extends into diverse sectors—including environmental science, national defense, and disaster management—where its applications may focus on wildfire detection based on spectral image analysis, among other sophisticated techniques.
With its broad perspective and scalability, satellite data enhances how stakeholders monitor, and even predict, the causation of wildfires. Typical applications include, but are not limited to:
- Remote monitoring: Continuously watch potential wildfire sites from afar.
- Behavioral spotting: Observe behavioral anomalies indicating heightened fire risk areas.
- Large-scale analysis: Study fire spread across multiple fronts.
- Topographical impact analysis: Ascertain how topography impacts fire dynamics.
- Mitigation resource allocation: Allocate resources based on live data from satellite monitoring.
Risk Data
Risk data, emerging largely from insurance and financial sectors, has evolved to present a calculated approach toward evaluating wildfire susceptibility at various geographic scopes. Although initially focused on covering assets, risk data has assumed broader interdisciplinary significance for wildfire analysis.
What sets modern risk data apart is its multidimensional nature. It assimilates climate risks, demographic markers, and infrastructure assessments into a singular schematic layer, allowing users to visualize systemic vulnerabilities.
Diverse stakeholders—from public safety officials to financial institutions—tap into risk data to understand and mitigate community-wide wildfire threats. Enhanced by computational power, this data set cultivates insights that translate directly into preparedness strategies and safety codes for vulnerable populations.
The breadth of risk data’s applications is reflected in diverse uses. Here are some:
- Socioeconomic profiling: Merge demographic data with fire risk data to identify at-risk populations for targeted assistance.
- Environmental impact studies: Study the ripple effects of wildfires on specific environmental and community factors.
- Resource mobilization strategies: Devise rapid deployment logistics based on analyzed risk data.
- Community safety protocols: Establish preventive community protocols aligned with identified risk benchmarks.
- Urban planning considerations: Integrate risk analytics into future city planning efforts to minimize wildfire exposure.
Conclusion
In summary, the wealth of diverse data sources significantly enhances how we perceive and strategize against the multifaceted threat of wildfires in North America. The introduction of varied datasets—from satellite imagery to predictive climate models—offers a multidimensional understanding that was previously unachievable.
As industries navigate an increasingly data-centric world, there is immense scope for adopting a data-driven mindset. This aligns with the future of decision-making, where entities leverage real-time insights for timely interventions and strategy optimization. Companies looking to harness such insights should consider employing external data as a pivotal element in their tactical arsenals.
Beyond individual case scenarios, the era of data monetization unlocks the opportunity for organizations to capitalize on historical data repositories. These repositories, previously an untapped goldmine, may spawn new product lines or services that further enrich data-informed operations against wildfires.
Anticipating future trends, continual innovations in data extraction and processing will likely birth novel categories. Expect developments around wildfire-specific social media sentiment analysis or AI-powered “smart” environmental sensors—contributing additional layers of risk identification and management.
In conclusion, the pursuit of comprehensive wildfire causation insights hinges on diligent exploitation of existing datasets and innovation in acquiring unexplored sources—fueling a proactive measure against the unpredictable wildfires that threaten North America.
Appendix
The revelation of comprehensive wildfire insights through data is invaluable to a spectrum of roles and industries. The implications for investors, insurance companies, consultants, and market researchers are substantial, covering the gambit of analysis prerequisites for risk evaluation and mitigation.
Insurance sectors, for example, leverage data streams from analytical dashboards to frame and manage wildfire coverage options, estimating potential damages accurately. Market researchers strategically use categorial data to chart environmental trends and inform environmentally conscious investments.
Consultants harness data insights to guide decision-making processes for urban development firms, streamlining plans to incorporate considerations that mitigate fire risks proactively. Industry professionals visualize how environmental datasets forecast relations between infrastructural developments and potential fire threats.
As organizations further their digital transformation pursuits, translating existing workflows into data-driven schemas becomes a collective goal. The potential of AI emerges as integral in unlocking the value ingrained within grandfathered documents, providing refined lenses for viewing data-driven futures.
Evaluating what might lie ahead, the integration of AI initiatives will likely achieve more granular data interpretations. This could transform how archaic filings reveal insights parallel to real-time analysis metrics gathering contemporary wildfire data.
Thus, empowering industries with meaningful data insights today remains a considerable opportunity. As the evolutionary arc of data ecosystems continues, fostering adaptive frameworks will drive the advancement of informed decision-making critical to wildfire resilience efforts advancing into the upcoming decades.